Energy consumption auditing based on a generative adversarial network for anomaly detection of robotic manipulators
FUTURE GENERATION COMPUTER SYSTEMS-THE INTERNATIONAL JOURNAL OF ESCIENCE(2023)
摘要
This paper presents a generative adversarial network (GAN)-based energy auditing and anomaly detection framework for the health and security monitoring of robotic manipulators. The system features two key components: a side-channel measurement of the energy consumption and a GAN model to learn the data patterns during normal operations only. Anomalies in energy consumption during online testing are then discovered as outliers to the normal data by scrutinizing the anomaly score produced by the discriminator of the GAN model. This semi-supervised network circumvents the need for vast data labeling and exploits the overfitting during the training stage, hence magnifying the difference between the normal patterns learned in training and the unobserved abnormal energy profiles in testing. Moreover, considering the physical aspects of the robotic manipulator, a modified GAN architecture containing multiple discriminators to account for distinct contributions from individual joints/motors. A dynamic thresholding approach that continuously updates the statistical characteristics of the anomaly score is also developed to detect outliers and mitigate the effect of environmental variations during system operation. The proposed framework is tested on the custom dataset for performance evaluation. The results demonstrate the feasibility of the proposed method, especially for detecting physical attacks, which achieves an accuracy of approximately 0.93 in instant-level detection and over 0.84 in event-level detection.
更多查看译文
关键词
Robotic manipulator,Energy auditing,Generative adversarial network,Anomaly detection,Side-channel mechanism,Cyber–physical attack
AI 理解论文
溯源树
样例
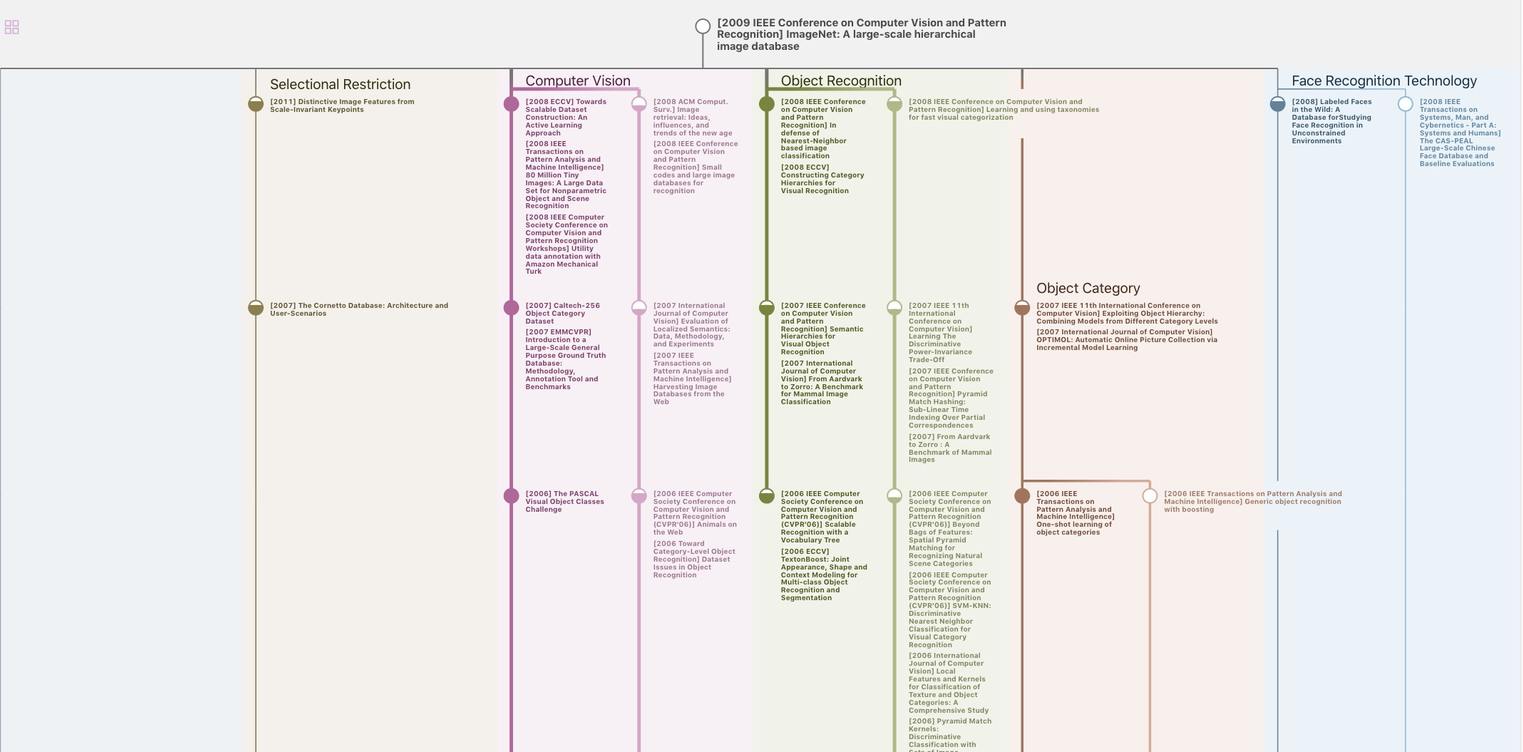
生成溯源树,研究论文发展脉络
Chat Paper
正在生成论文摘要