Near-Optimal Active Learning for Multilingual Grapheme-to-Phoneme Conversion
APPLIED SCIENCES-BASEL(2023)
摘要
The construction of pronunciation dictionaries relies on high-quality and extensive training data in data-driven way. However, the manual annotation of corpus for this purpose is both costly and time consuming, especially for low-resource languages that lack sufficient data and resources. A multilingual pronunciation dictionary includes some common phonemes or phonetic units, which means that these phonemes or units have similarities in the pronunciation of different languages and can be used in the construction process of pronunciation dictionaries for low-resource languages. By using a multilingual pronunciation dictionary, knowledge can be shared among different languages, thus improving the quality and accuracy of pronunciation dictionaries for low-resource languages. In this paper, we propose using shared articulatory features among multiple languages to construct a universal phoneme set, which is then used to label words for multiple languages. To achieve this, we first developed a grapheme-phoneme (G2P) model based on an encoder-decoder deep neural network. We then adopted a near-optimal active learning method in the process of building the pronunciation dictionary to select informative samples from a large, unlabeled corpus and had them labeled by experts. Our experiments demonstrate that this method selected about 1/5 of the unlabeled data and achieved an even higher conversion accuracy than the results of the large data training method. By selectively labeling samples with a high uncertainty in the model, while avoiding labeling samples that were accurately predicted by the current model, our method greatly enhances the efficiency of pronunciation dictionary construction.
更多查看译文
关键词
multilingual, grapheme-to-phoneme conversion, pronunciation dictionaries, low-resource languages, active learning
AI 理解论文
溯源树
样例
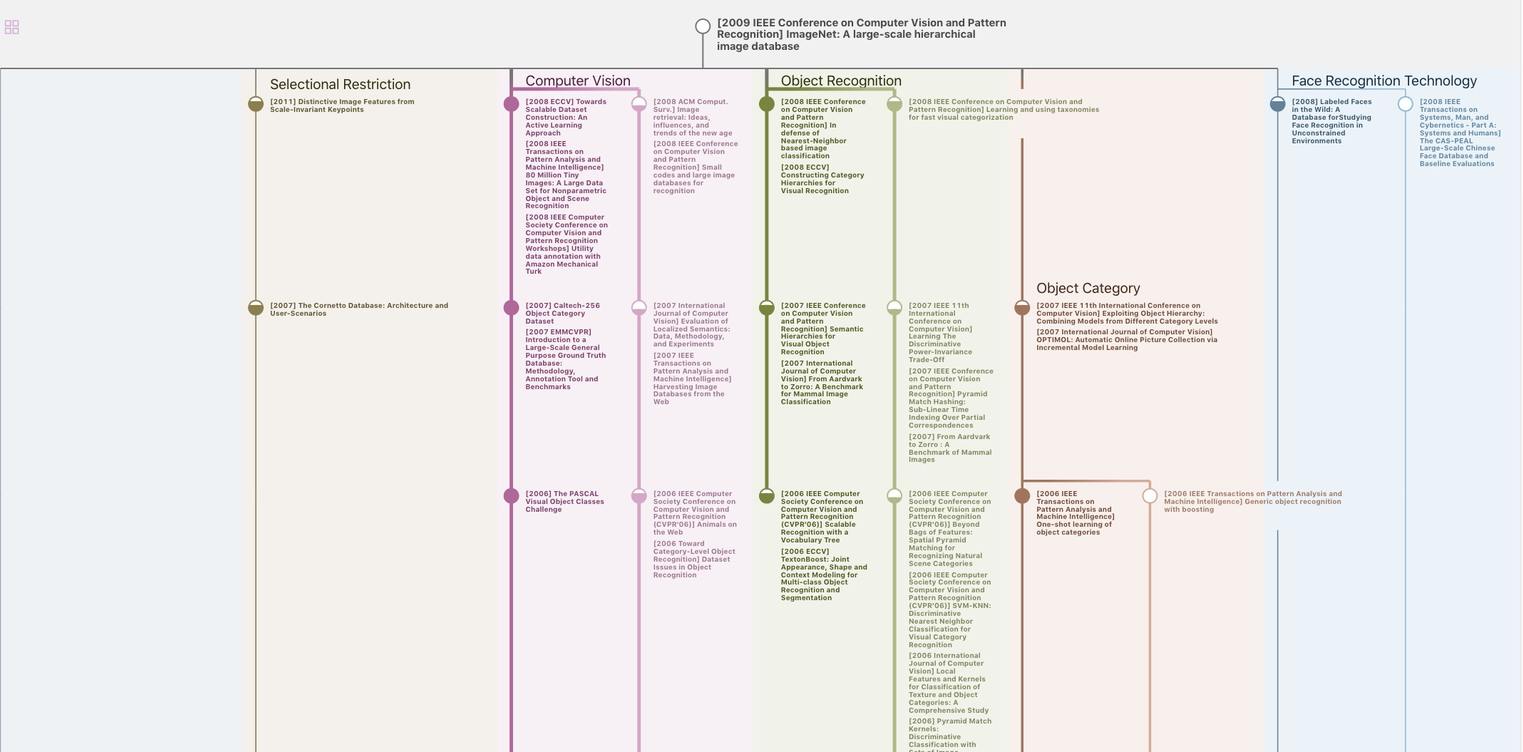
生成溯源树,研究论文发展脉络
Chat Paper
正在生成论文摘要