Training Deep Learning Spacecraft Component Detection Algorithms Using Synthetic Image Data
2023 IEEE AEROSPACE CONFERENCE(2023)
摘要
On-orbit satellite servicing can benefit from increased local autonomy when long time delays prevent effective teleoperation. One required perception capability is automated detection and classification of spacecraft components. Recent developments in Deep Learning (DL) object detection can be applied to spacecraft component detection and classification. However, training such algorithms requires large amounts of labeled training images. In the satellite servicing domain, large datasets of labeled on-orbit satellite images generally do not exist. In this paper, we compare two approaches for training DL object detectors for data starved applications. In the first approach, a small number of real satellite images were manually labeled and then augmented via transformations to obtain sufficient training images. In the second, synthetic images were generated in simulation and automatically labeled, creating four datasets, one the same size as the augmented real dataset and three larger datasets. Our main objective was to demonstrate that synthetic images can be used to successfully train spacecraft component detectors, eliminating the need to collect and manually label large sets of real satellite images. Our secondary objective was to assess the effect of the size of the synthetic training dataset on detection performance. The small set of real images used are photographs of the Hubble Space Telescope (HST) taken during HST servicing missions. The Unity video game engine was used to simulate the HST and space environment, and to generate synthetic image datasets in which the lighting, viewing geometry, and surface material properties were varied. Labeling involved drawing bounding boxes around five object classes: the telescope aperture cover, telescope baffle, solar panels, antennas, and spacecraft bus. Multiple DL Faster Regional Convolutional Neural Network (Faster R-CNN) object detectors were trained to detect all five classes using multiple random subsets of the augmented real dataset and of the synthetic datasets. All of the detectors were tested on random subsets of images from the augmented real dataset. Receiver Operating Characteristic (ROC) curves were generated and the Area Under the Curve (AUC) was calculated for each class. For each Faster R-CNN detector, the average AUC across all classes was used as a single figure of merit for object detection performance. We found that detectors trained on synthetic Unity images can perform as well or better than detectors trained on an augmented set of a small number of real images, and that performance improves as the number of synthetic training images increases.
更多查看译文
关键词
augmented real dataset,augmented set,data starved applications,effective teleoperation,Faster R-CNN detector,HST servicing missions,Hubble space telescope,increased local autonomy,labeled on-orbit satellite images,labeled training images,long time delays,multiple DL faster regional convolutional neural network object detectors,object classes,object detection performance,on-orbit satellite servicing,required perception capability,satellite servicing domain,secondary objective,spacecraft bus,spacecraft component detection algorithms,spacecraft component detectors,spacecraft components,sufficient training images,synthetic datasets,synthetic image data,synthetic image datasets,synthetic images,synthetic training dataset,synthetic training images increases,synthetic unity images
AI 理解论文
溯源树
样例
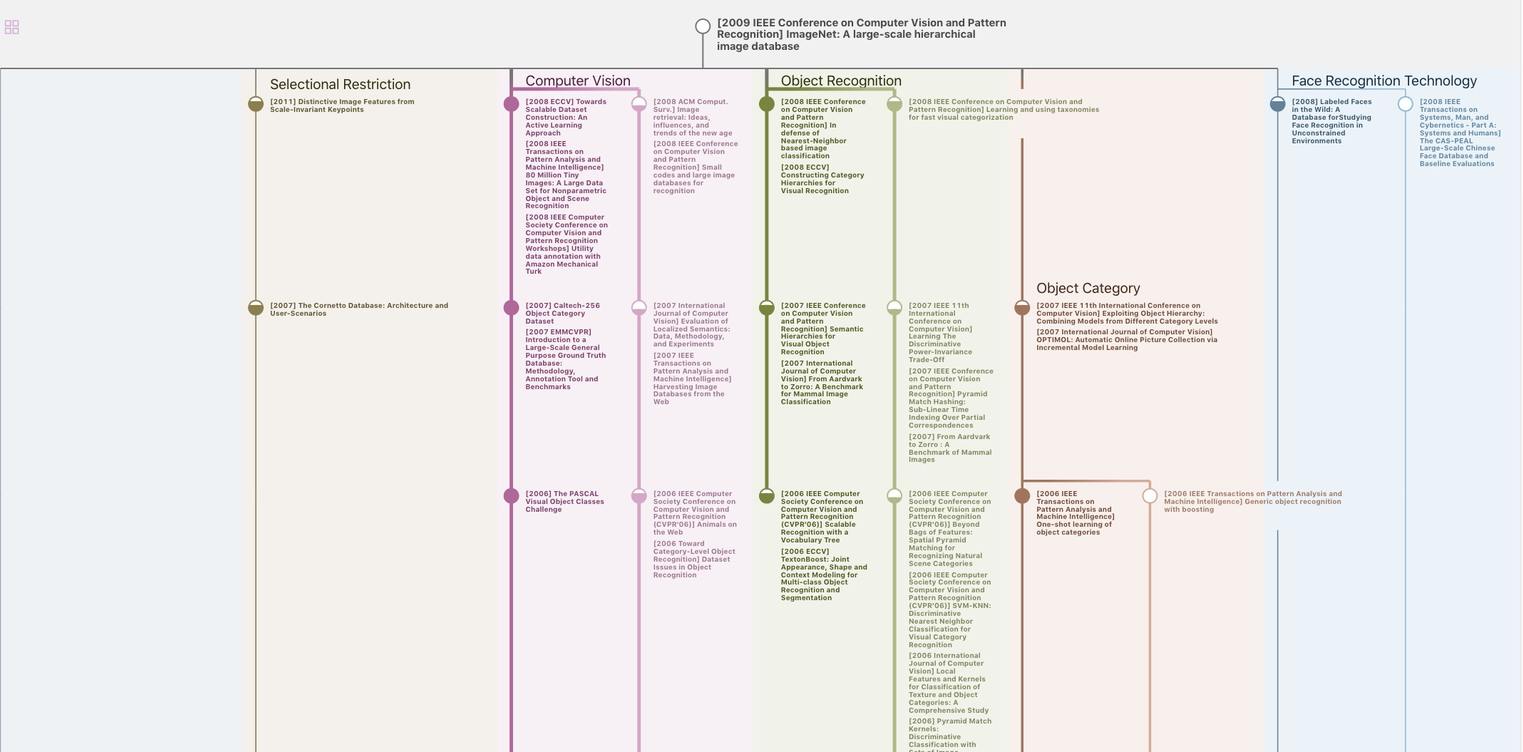
生成溯源树,研究论文发展脉络
Chat Paper
正在生成论文摘要