Benchmarking Deep Learning Models on Myriad and Snapdragon Processors for Space Applications
JOURNAL OF AEROSPACE INFORMATION SYSTEMS(2023)
摘要
Future space missions can benefit from processing imagery on board to detect science events, create insights, and respond autonomously. One of the challenges to this mission concept is that traditional space flight computing has limited capabilities because it is derived from much older computing to ensure reliable performance in the extreme environments of space: particularly radiation. Modern commercial-off-the-shelf processors, such as the Movidius Myriad X and the Qualcomm Snapdragon, provide significant improvements in small size, weight, and power packaging; and they offer direct hardware acceleration for deep neural networks, although these processors are not radiation hardened. We deploy neural network models on these processors hosted by Hewlett Packard Enterprise's Spaceborne Computer-2 on board the International Space Station (ISS). We find that the Myriad and Snapdragon digital signal processors (DSP)/artificial intelligence processors (AIP) provide speed improvement over the Snapdragon CPU in all cases except single-pixel networks (typically greater than 10 times for DSP/AIP). In addition, the discrepancy introduced through quantization and porting of our Jet Propulsion Laboratory models was usually quite low (less than 5%). Models were run multiple times, and memory checkers were deployed to test for radiation effects. To date, we have found no difference in output between ground and ISS runs, and no memory checker errors.
更多查看译文
关键词
snapdragon processors,deep learning models,deep learning,myriad
AI 理解论文
溯源树
样例
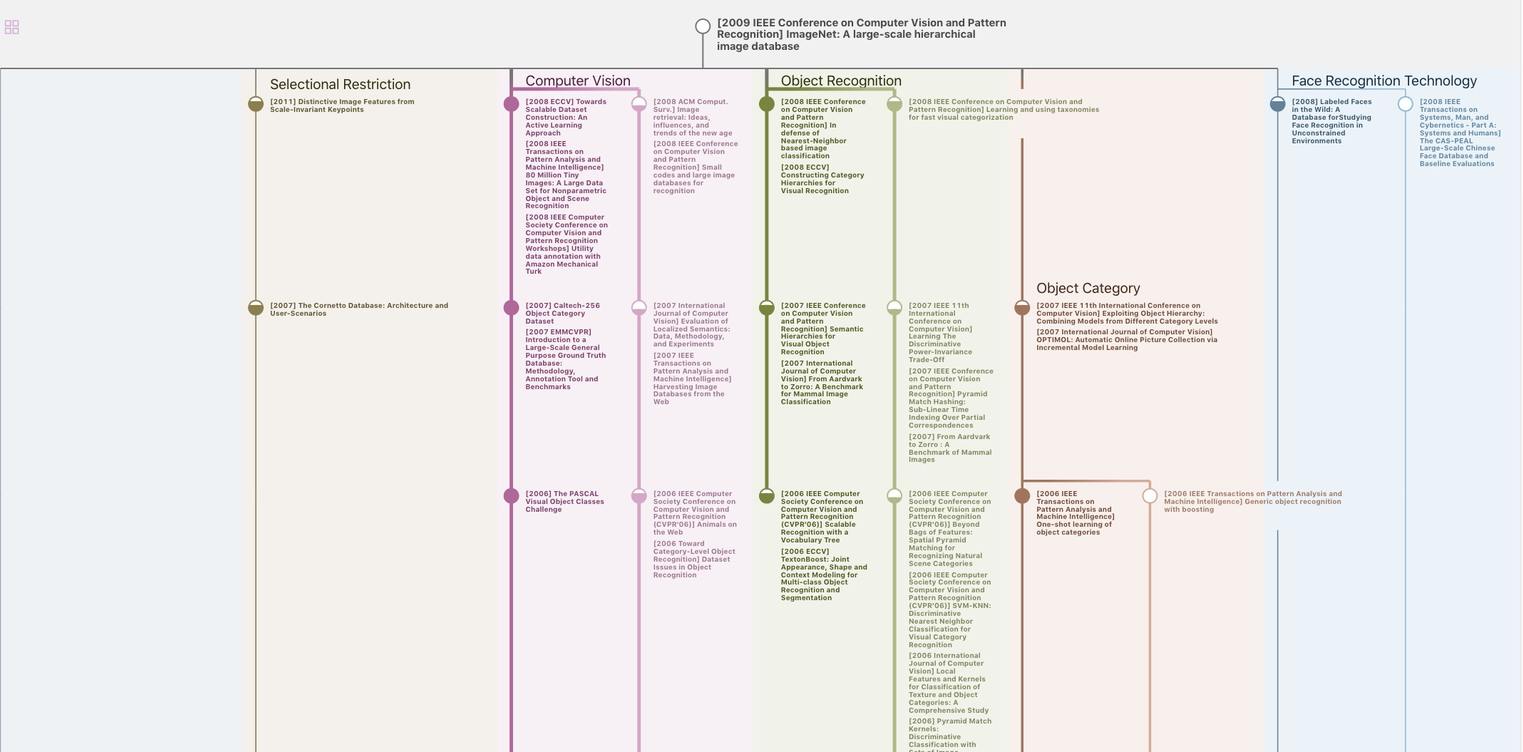
生成溯源树,研究论文发展脉络
Chat Paper
正在生成论文摘要