Interpretable machine learning for predicting evaporation from Awash reservoirs, Ethiopia
EARTH SCIENCE INFORMATICS(2023)
摘要
An in-depth understanding of a key element such as lake evaporation is particularly beneficial in developing the optimal management approach for reservoirs. In this study, we first aim to evaluate the applicability of regressors Random Forest (RF), Gradient Booting (GB), and Decision Tree (DT), K Nearest Neighbor (kNN), and XGBoost architectures to predict daily lake evaporation of five reservoirs in the Awash River basin, Ethiopia. The best performing models, Gradient Boosting and XGBoost, are then explained through an explanatory framework using daily climate datasets. The interpretability of the models was evaluated using the Shapley Additive explanations (SHAP). The GB model performed better with (RMSE = 0.045, MSE = 0.031, MAE = 0.002, NSE = 0.997, KGF = 0.991, RRMSE = 0.011) for Metehara Station, (RMSE = 0.032, MSE = 0.024, MAE = 0.001, NSE = 0.998, KGF = 0.999, RRMSE = 0.008) at Melkasa Station, and Dubti Station (RMSE = 0.13, MSE = 0.09, MAE = 0.017, NSE = 0.982, KGF = 0.977,RRMSE = 0.022) as the same as of XGBoost. The factors with the greatest overall impact on the daily evaporation for GB and XGboost Architecture were the SH, month, Tmax, and Tmin for Metehara and Melkasa, and Tmax, Tmin, and month had the greatest impact on the daily evaporation for Dubti. Furthermore, the interpretability of the models showed good agreement between the MLAs simulations and the actual hydro-climatic evaporation process. This result allows decision makers to not only rely on the results of an algorithm, but to make more informed decisions by using interpretable results for better control of the basin reservoir operating rules.
更多查看译文
关键词
Gradient Booting Regression,XGboost,Interpretable Machine Learning,SHAP,Daily Lake Evaporation
AI 理解论文
溯源树
样例
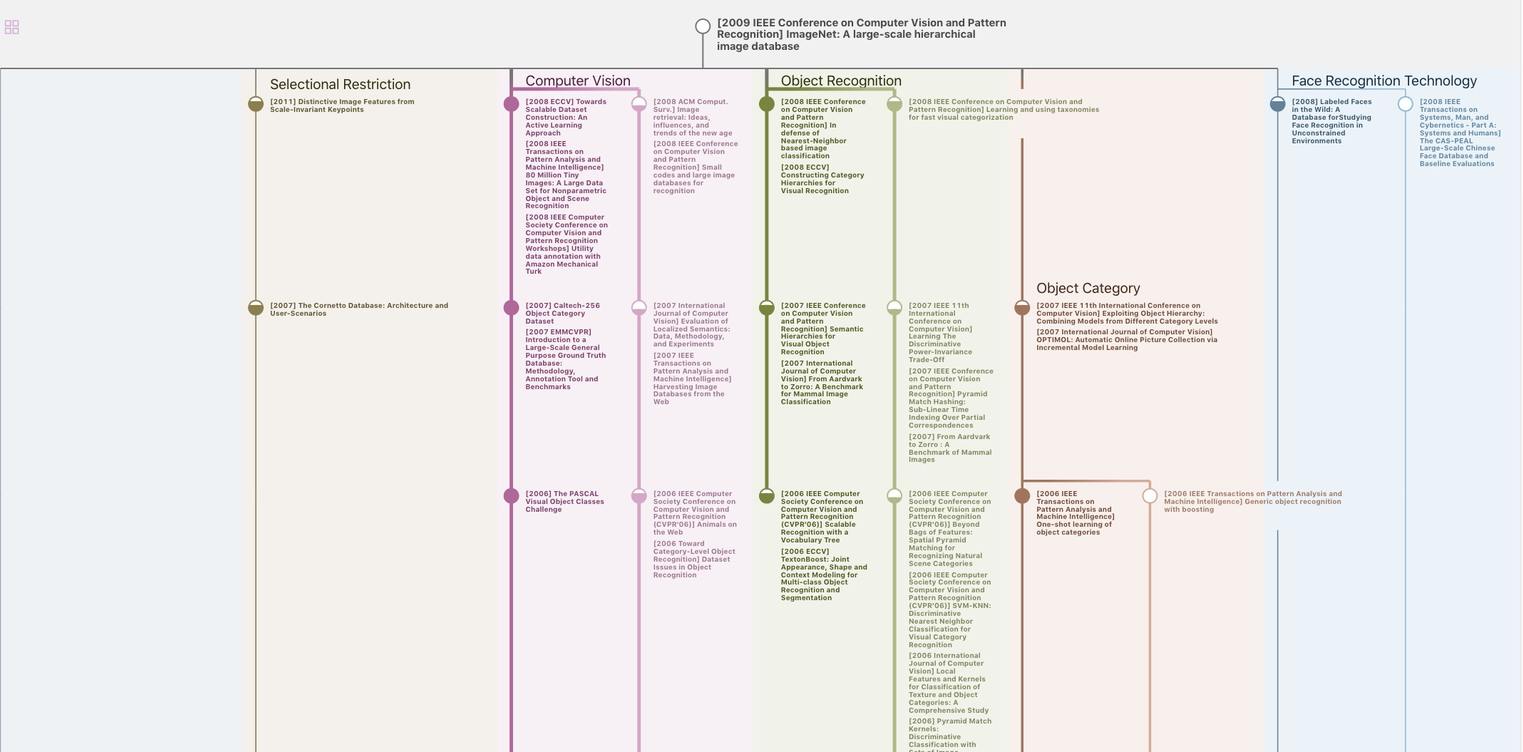
生成溯源树,研究论文发展脉络
Chat Paper
正在生成论文摘要