Efficient framework for low-resource abstractive summarization by meta-transfer learning and pointer-generator networks
EXPERT SYSTEMS WITH APPLICATIONS(2023)
摘要
Recently, large language models have shown great success on various abstractive summarization datasets. These datasets consist of numerous data that are enough to train a large number of parameters. However, for a new domain, there is a lack of labeled data to train those parameters and the model is easily overfitted to a small amount of data. In addition, because annotating document-summary pairs is too expensive and transfer learning using high-resource datasets causes a domain shifting problem, a low-resource abstractive summarization task is becoming necessary. Herein, we propose an efficient framework for low-resource abstractive summarization using a pointer-generator network and a meta-learning technique to address the above problems. Meta-learning using existing high-resource datasets enables our model to rapidly adapt to a new domain using limited data to solve the domain shifting problem. In addition, we explore the copy mechanism using a pointer-generator network that can copy words from a source document when generating a summary. The experimental results on 11 different datasets show that the proposed model outperforms the previous state-of-the-art models in low-resource abstractive summarization on most of the datasets.
更多查看译文
关键词
Low-resource abstractive summarization,Meta-transfer learning,Pointer-generator network,Copy mechanism,Prompt-tuning
AI 理解论文
溯源树
样例
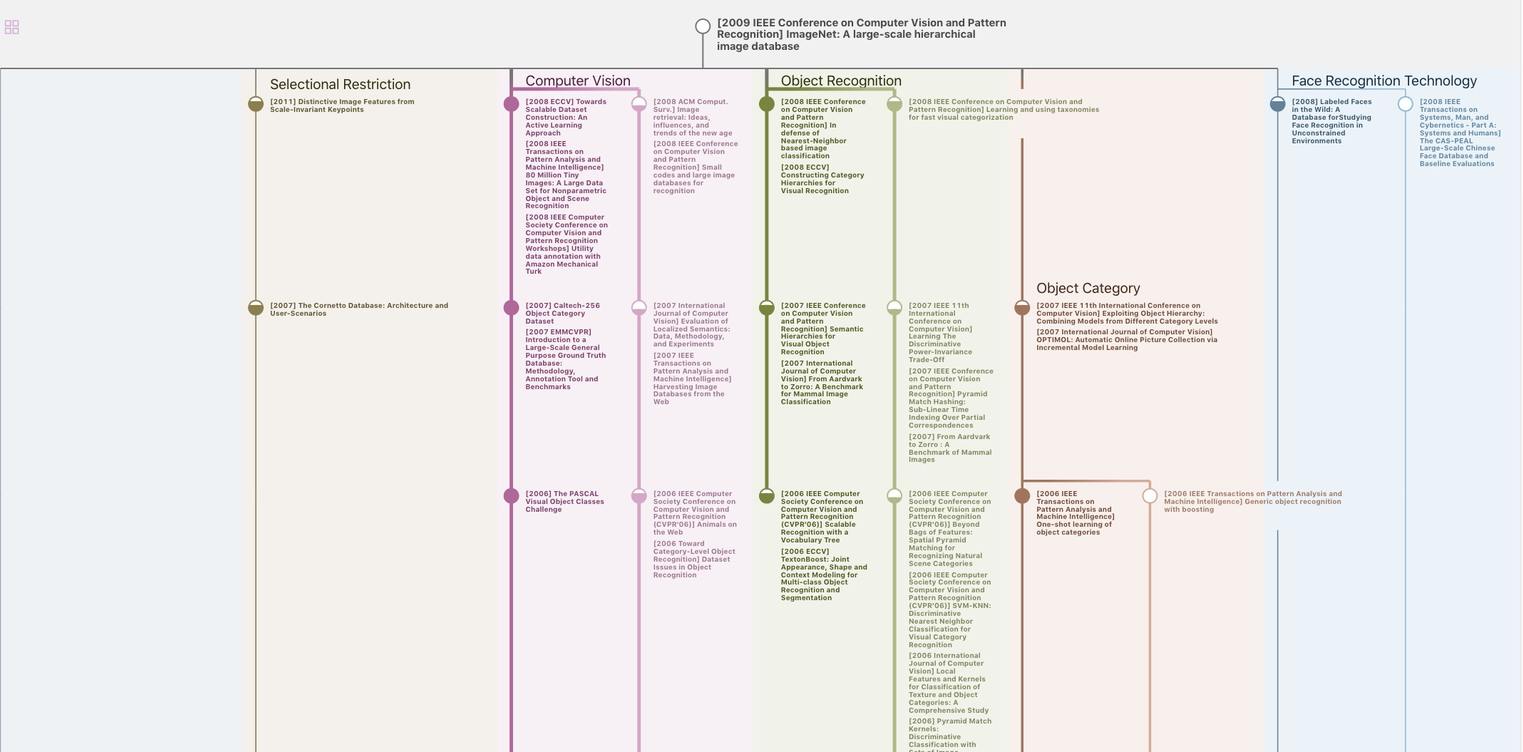
生成溯源树,研究论文发展脉络
Chat Paper
正在生成论文摘要