Multi-task learning for the bearing based on a one-dimensional convolutional neural network with attention guidance mechanism and multi-scale feature extraction
INSIGHT(2023)
摘要
Fault type and fault degree identification are the main aim in the bearing multi-task learning. However, a large number of on-site accidents have shown that the bearing working condition plays an important role in bearing service life and fault diagnosis. In current studies, the bearing working condition identification task is often used for auxiliary tasks and is easily ignored. Thus, this paper studies the bearing multi-task learning, which regards the working condition identification task as an equally important task. However, simply adding the working condition identification task to the frequently used multi-task model will lead to a reduction in the overall performance of the network. To solve the network performance degradation problem, a succinct and effective multi-task one-dimensional convolutional neural network with attention guidance mechanism and multi-scale feature extraction (MAM-1DCNN) is proposed. Firstly, the time-series signal is selected as the input of the MAM-1DCNN model. Secondly, the shared network of the MAM-1DCNN model applies a double-layer multi-scale convolutional neural network structure to extract more complete information. Finally, the MAM-1DCNN applies an improved attention guidance mechanism to enhance the feature application ability of different branch tasks. Through two general bearings datasets, this paper verifies the effectiveness and generalisation ability of the MAM-1DCNN model.
更多查看译文
关键词
bearing,attention guidance mechanism,feature,multi-task,one-dimensional,multi-scale
AI 理解论文
溯源树
样例
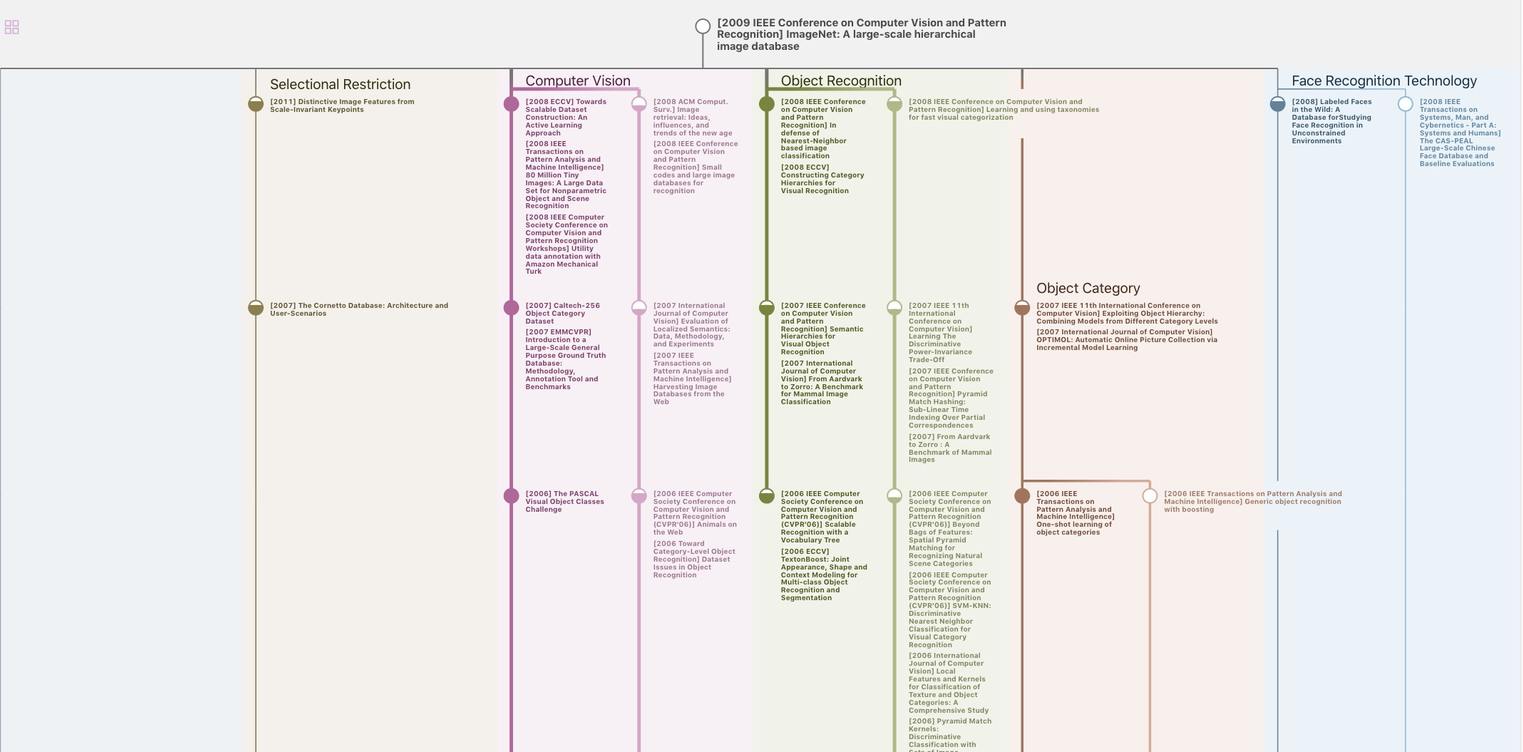
生成溯源树,研究论文发展脉络
Chat Paper
正在生成论文摘要