An Efficient and Accurate Neural Network Tool for Finding Correlation Between Gene Expression and Histological Images
CLINICAL IMAGE-BASED PROCEDURES, FAIRNESS OF AI IN MEDICAL IMAGING, AND ETHICAL AND PHILOSOPHICAL ISSUES IN MEDICAL IMAGING, CLIP 2023, FAIMI 2023, EPIMI 2023(2023)
摘要
Tumor development is clinically characterized through the manual review of histopathological Whole Slide Images (WSI). However, the molecular attributes influencing tumor morphology are not entirely comprehended. Here, we present RNALerner, an innovative tool designed to expedite the identification of correlations between gene expression and tumor morphology as presented in H&E WSI. RNALerner achieves its efficiency by transforming the problem from linear regression to binary classification of high versus low RNA levels, and the use of Resnet18, Convolutional Neural Network (CNN) model. Furthermore, the training phase of the model is halted after only 3 iterations. Upon comparing our results with previous work, we discovered a similar number of statistically significant correlated genes but with a reduction in the number of model parameters and processing time. Analysis of the significant pathways revealed both similarities to and deviations from earlier findings, bringing forth new pathways in the process. RNALerner represents an advancement toward the practical integration of machine learning in WSI analysis, which holds the potential to substantially improve disease diagnosis and guide more effective treatments.
更多查看译文
关键词
Convolutional neural network,H&E Whole slide image
AI 理解论文
溯源树
样例
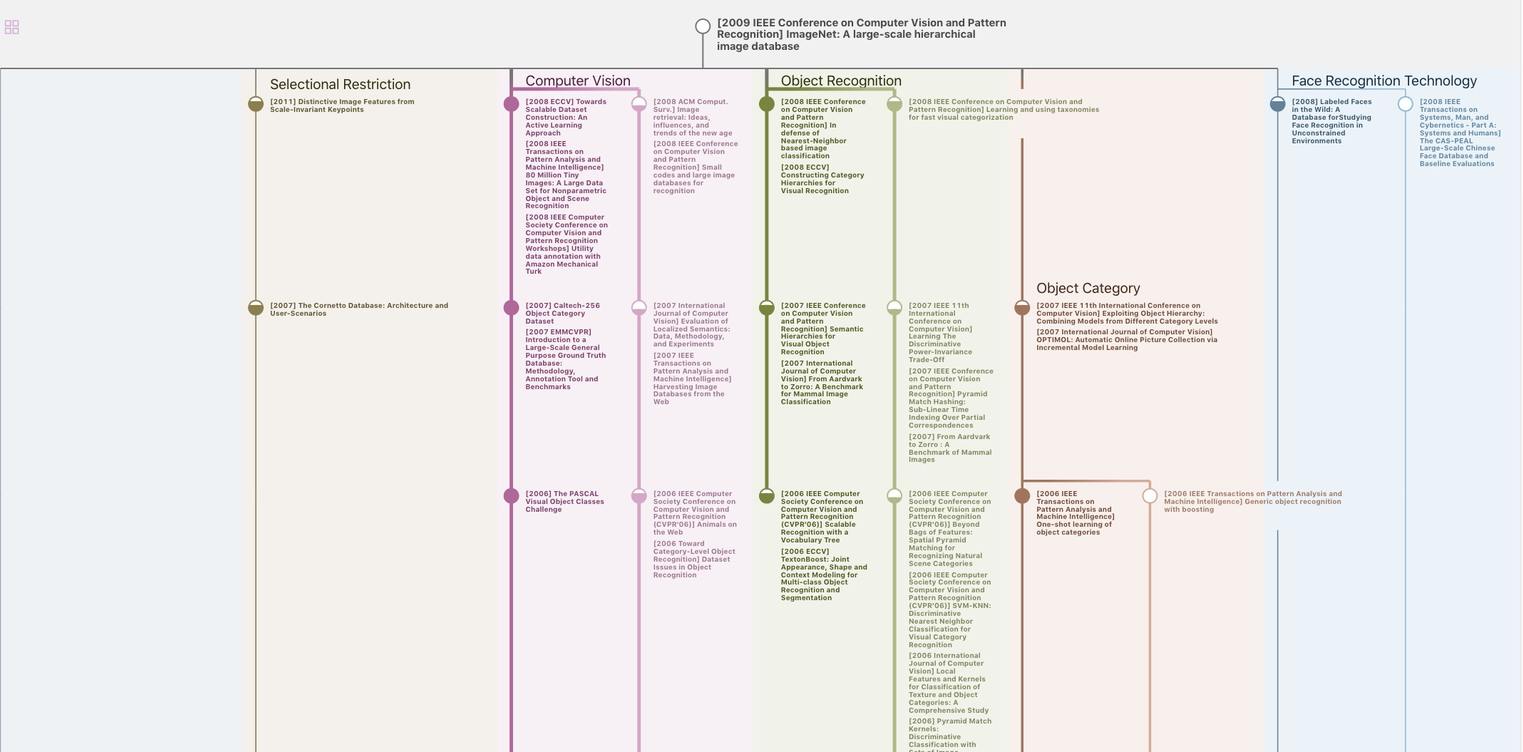
生成溯源树,研究论文发展脉络
Chat Paper
正在生成论文摘要