Improving air quality assessment using physics-inspired deep graph learning
npj Climate and Atmospheric Science(2023)
摘要
Existing methods for fine-scale air quality assessment have significant gaps in their reliability. Purely data-driven methods lack any physically-based mechanisms to simulate the interactive process of air pollution, potentially leading to physically inconsistent or implausible results. Here, we report a hybrid multilevel graph neural network that encodes fluid physics to capture spatial and temporal dynamic characteristics of air pollutants. On a multi-air pollutant test in China, our method consistently improved extrapolation accuracy by an average of 11–22% compared to several baseline machine learning methods, and generated physically consistent spatiotemporal trends of air pollutants at fine spatial and temporal scales.
更多查看译文
关键词
Environmental monitoring,Environmental sciences,Statistics,Earth Sciences,general,Climate Change/Climate Change Impacts,Atmospheric Sciences,Climatology,Atmospheric Protection/Air Quality Control/Air Pollution
AI 理解论文
溯源树
样例
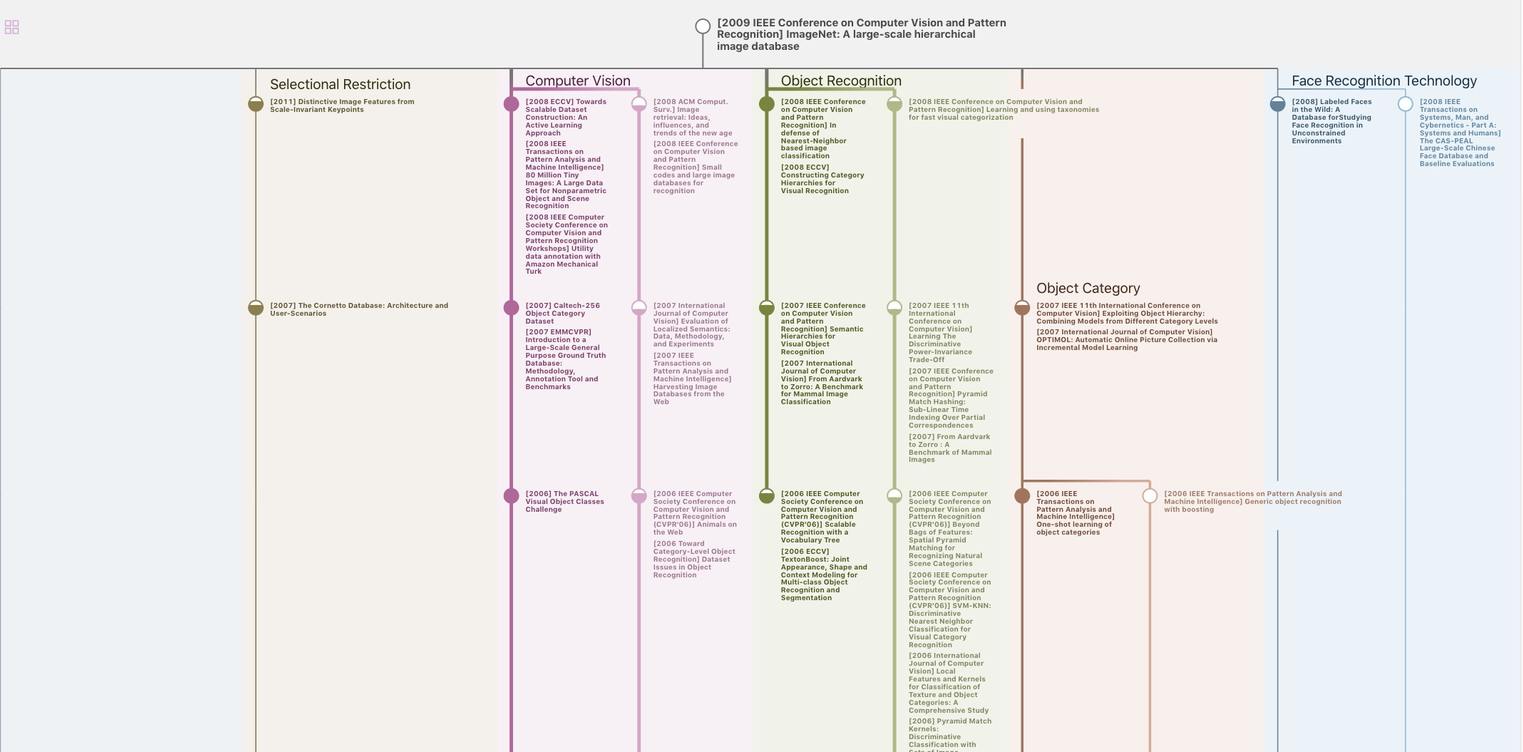
生成溯源树,研究论文发展脉络
Chat Paper
正在生成论文摘要