Using an optimized texture index to monitor the nitrogen content of potato plants over multiple growth stages.
Comput. Electron. Agric.(2023)
摘要
Plant nitrogen content (PNC) is vital for evaluating crop nitrogen nutrient status and for net primary productivity. Therefore, rapid and accurate acquisition of crop PNC information can help reduce fertilizer and increase efficiency in modern agriculture. This study investigated whether an optimized texture index constructed from hyperspectral characteristic wavelength images acquired from a unmanned aerial vehicle (UAV) may be used to estimate PNC in potatoes over multiple growth stages. A potato field trial conducted in 2019 in Beijing, China, included different planting densities, nitrogen fertilizer gradients, and potato varieties with three replicates. A UAV remote sensing platform served to acquire hyperspectral images during three critical N demand periods for potatoes. In addition, we simultaneously conducted field sampling to obtain ground-truth PNC measurements. Following the classical form of vegetation indices, 12 texture indices were constructed based on hyperspectral texture features, and an arbitrary variable combination optimization algorithm was used to optimize these indices. Finally, the texture index, which had the highest correlation with potato PNC, was used as the best indicator for estimating the PNC status of potato at multiple growth stages and whether this indicator, in combination with spectral information, could further improve the accuracy of potato PNC estimation was subsequently explored. The results showed that (i) the optimal texture index TTI3 (R494-Cor, R578-Hom, R514-Sem) maintained a good linear relationship with PNC at the late stage of potato growth, and the accuracy and stability of the PNC estimation models constructed based on it was significantly better than that of a single texture metric. (ii) Compared with spectral information alone, the texture index combined with spectral features improved the accuracy of potato PNC estimation. More specifically, TTI3 (R494-Cor, R578-Hom, R514-Sem) combined with the three-band spectral index TBI 5 (530, 734, 514) achieved the best estimation accuracy with calibrated R2, root mean square error (RMSE), and normalized RMSE of 0.77%, 0.28%, and 9.88%, respectively. The results of this study showed that the texture index constructed by combining multiple texture metrics enhanced the association between texture features and potato PNC over multiple growth stages, thus improving the monitoring accuracy of potato nitrogen nutrition status. This study can provide a reference for accurately managing crop nitrogen nutrition.
更多查看译文
关键词
Potato,Plant nitrogen content,Texture index,Unmanned aerial vehicle,Hyperspectral
AI 理解论文
溯源树
样例
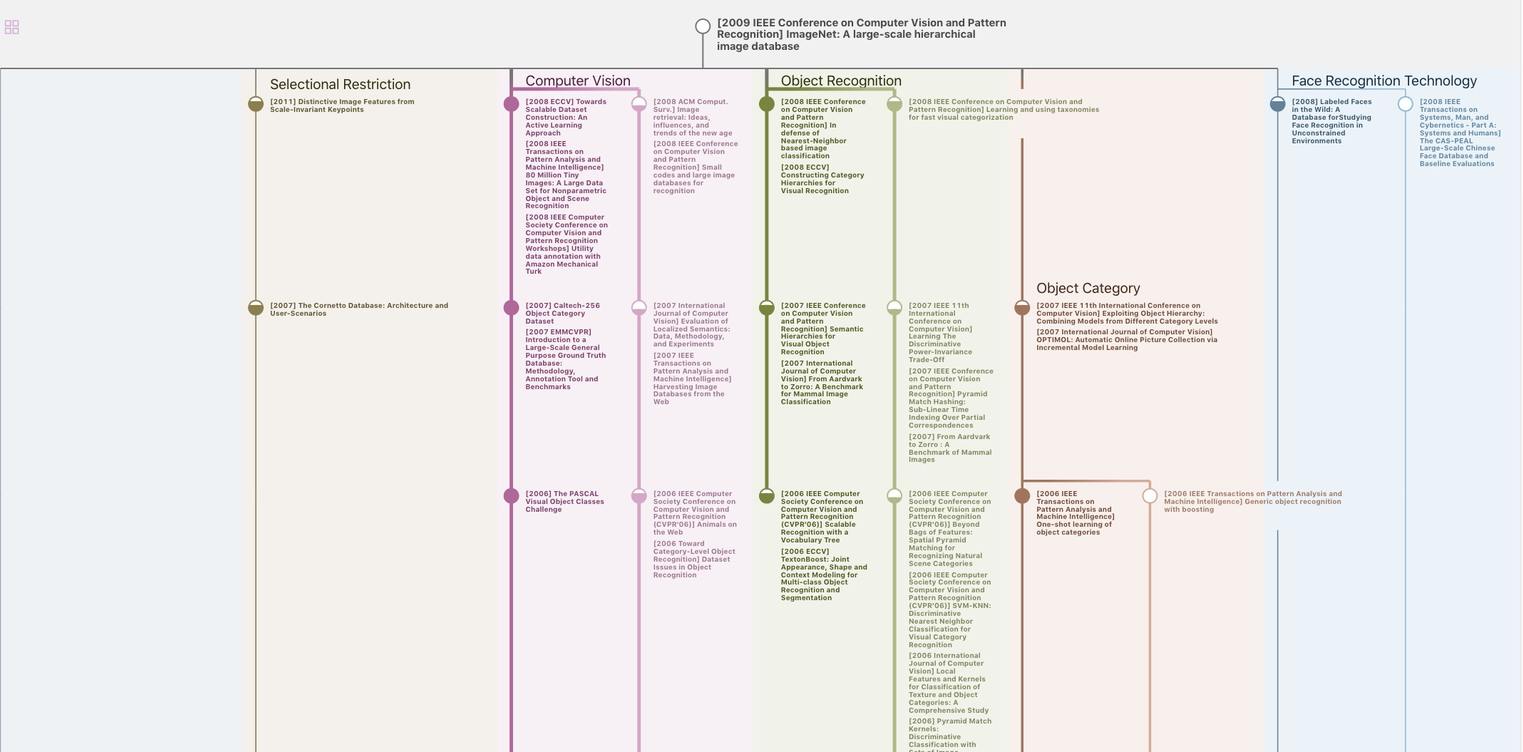
生成溯源树,研究论文发展脉络
Chat Paper
正在生成论文摘要