High-speed anomaly traffic detection based on staged frequency domain features.
J. Inf. Secur. Appl.(2023)
摘要
Anomaly detection methods based on machine learning assist in identifying attacker behavior concealed in critical infrastructure's high-speed network traffic. However, these methods generally experience problems including a lack of labeled data and poor performance. We suggest a detection method based on staged frequency domain features to address these issues. A small-step sliding window is used in the training phase to fully understand the frequency domain features of the traffic. We suggest SOM-Kmeans, an integrated clustering technique that can accurately distinguish between malicious and benign flows. We evaluate the SOM-Kmeans accuracy using open datasets and assess its effectiveness in a real network environment. The experimental results demonstrate that our method can detect anomaly traffic at high speed without sacrificing detection accuracy.
更多查看译文
关键词
Network security,Anomaly detection,Frequency domain features,Unsupervised learning
AI 理解论文
溯源树
样例
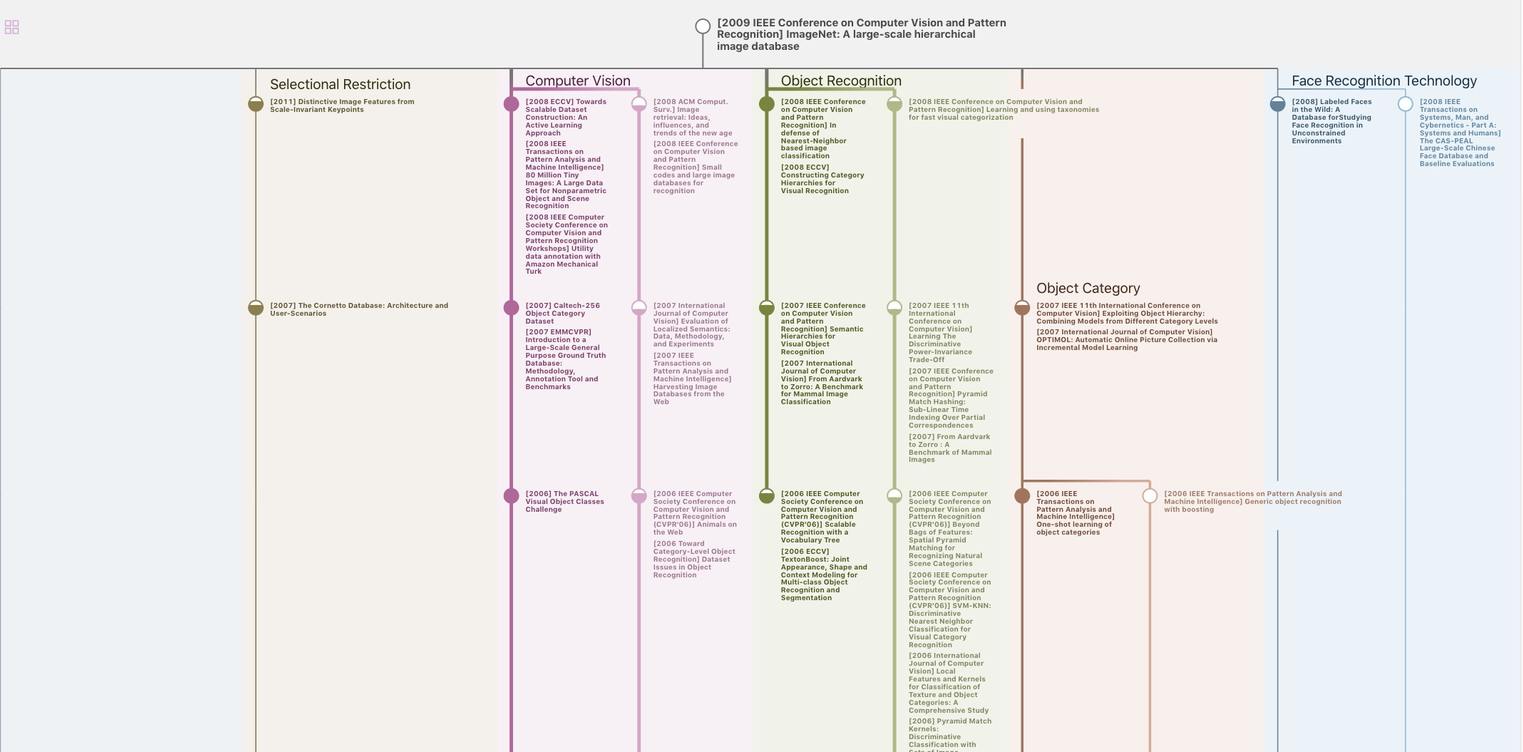
生成溯源树,研究论文发展脉络
Chat Paper
正在生成论文摘要