Dual population approximate constrained Pareto front for constrained multiobjective optimization.
Inf. Sci.(2023)
摘要
For constrained multiobjective optimization problems (CMOPs), the ultimate goal is to obtain a set of well-converged and well-distributed feasible solutions to approximate the constrained Pareto front (CPF). Various constraints may change the position and/or shape of the CPF. This poses great challenges to the approximation of the CPF. This is especially true when the CPF mainly lies on constraint boundaries (i.e., CPF and unconstrained PF have little or even no intersection). To tackle this issue, we propose a novel dual population algorithm for approximating the CPF from both sides of the constraint boundaries. Specifically, Population 1 uses the constrained-domination principle to approximate the CPF from the sides of feasible regions only; Population 2 adopts an improved c-constrained method to approximate the CPF from both the feasible as well as infeasible regions. Offspring generated by both populations are merged and combined with Population1 and Population2. In addition, some selected members of Population1 and Population 2 are permitted to migrate to the combined populations to facilitate knowledge sharing. Systematic experiments carried out on three benchmark test suites and 10 real-world CMOPs show the proposed algorithm achieved superior or competitive performance, especially for CMOPs where the CPF is mainly located at constraint boundaries. Therefore, on the basis of dual population, approximating CPFs from both sides of feasible and infeasible regions contributes an alternative approach to solving CMOPs.
更多查看译文
关键词
Constrained multiobjective optimization,Dual population,Constraint boundaries,Evolutionary algorithms
AI 理解论文
溯源树
样例
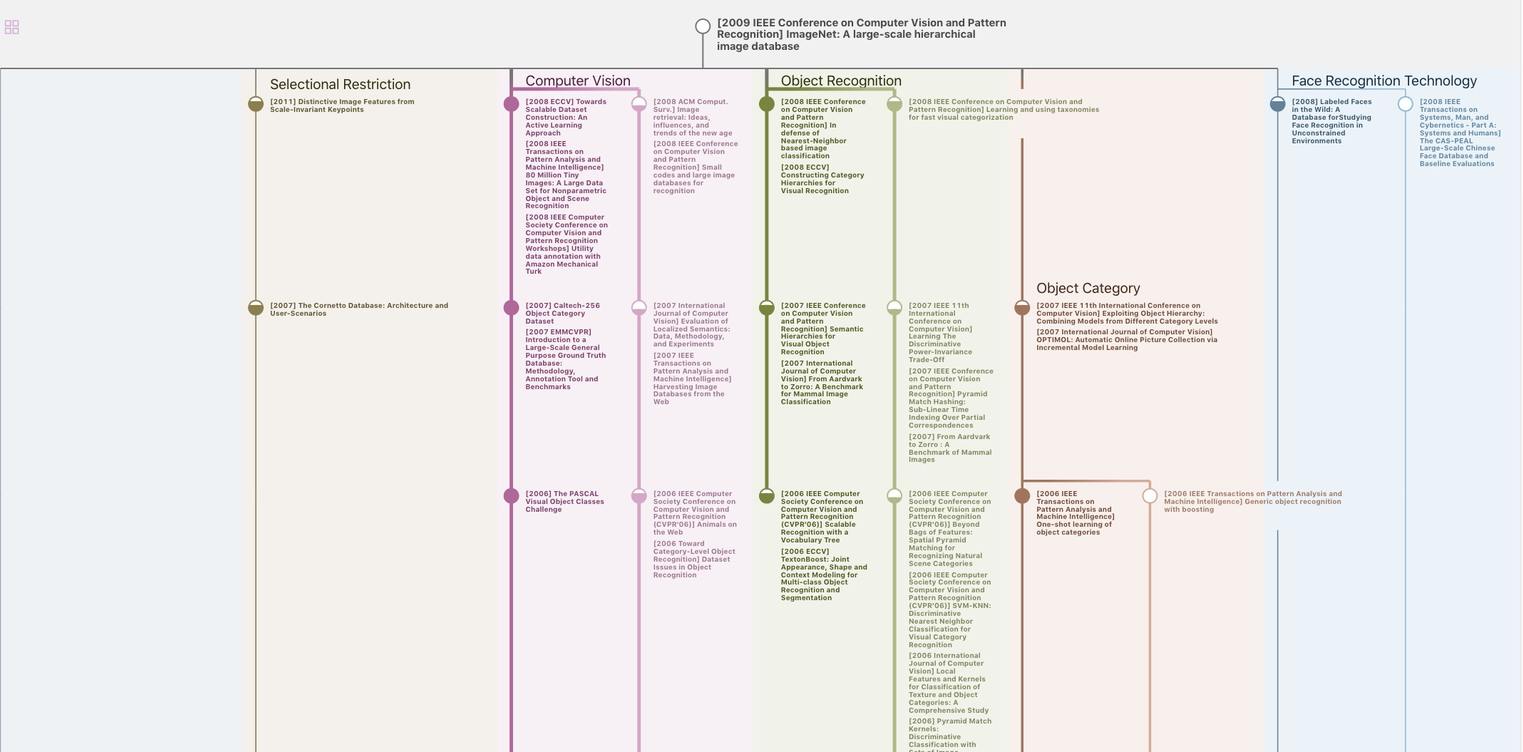
生成溯源树,研究论文发展脉络
Chat Paper
正在生成论文摘要