Multimodal Low-Rank Tensor Subspace Learning for Hyperspectral Image Restoration.
IEEE Geosci. Remote. Sens. Lett.(2023)
摘要
The restoration of hyperspectral images (HSIs) is a crucial process that eliminates various types of noise to improve subsequent applications. To effectively utilize the inherent low-rank and spatial smoothness of HSI data, this letter proposes a method that employs multimodal low-rank tensor subspace learning with total variation regularization (MLTSL-TV) model to denoise HSI data based on the observed measurements. The proposed approach utilizes a low-rankness measure of subspace tensors and learnable transform basis to represent a low-rank perspective, which enables adaptive exploitation of potential low-rank structures through multimodal tensor factorization in multiple orientations based on the observed HSI data. More importantly, we put forward a proximal alternating minimization (PAM) algorithm for efficiently solving the proposed model. Experiments were conducted on two simulated and one real HSI dataset, which were compared with representative approaches through both visual and quantitative analysis. The experimental results demonstrate that the proposed MLTSL-TV approach achieves satisfactory performance when compared to the state-of-the-art methods.
更多查看译文
关键词
Hyperspectral image (HSI) restoration, low rankness, multimodal tensor factorization, subspace learning
AI 理解论文
溯源树
样例
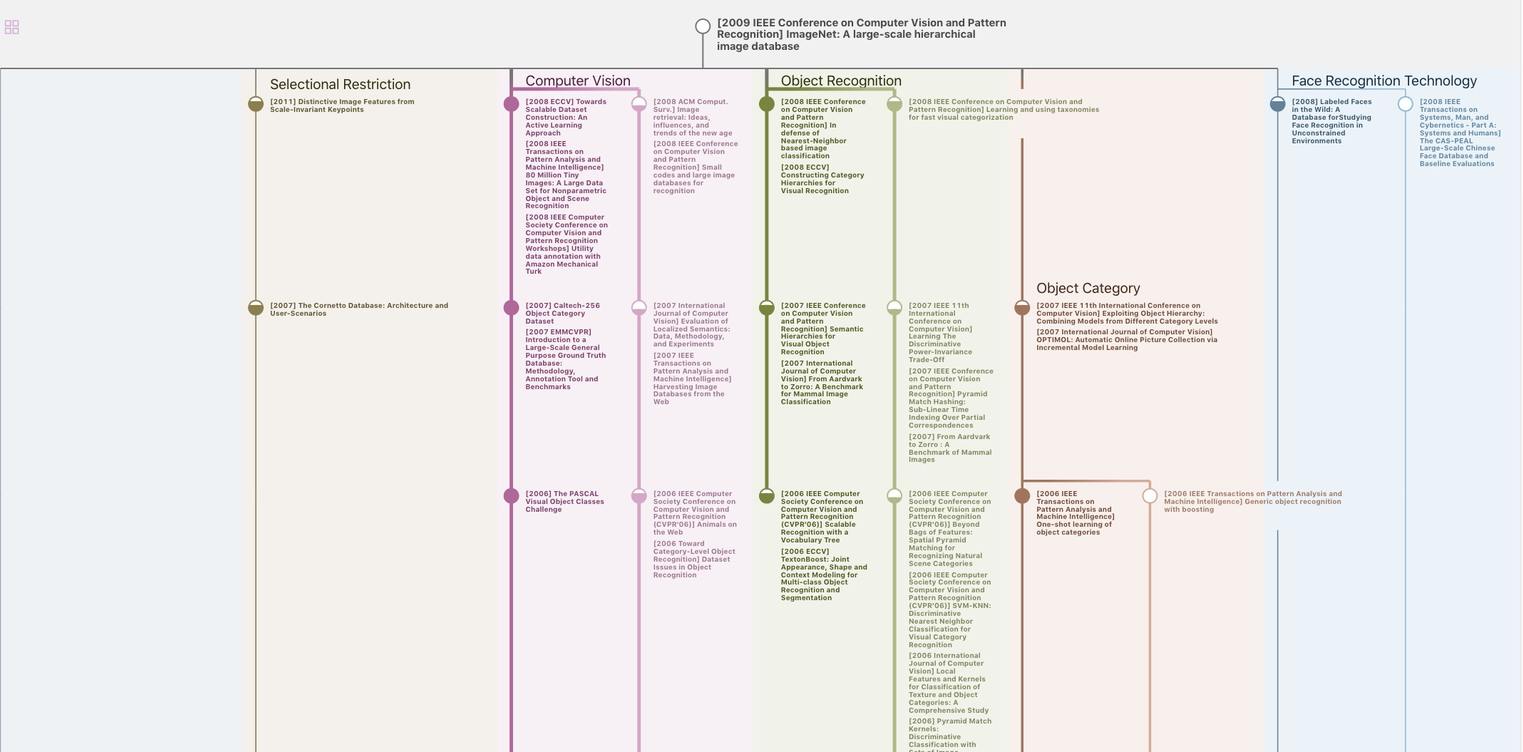
生成溯源树,研究论文发展脉络
Chat Paper
正在生成论文摘要