Efficient Compressed Sensing Reconstruction Algorithm for Nonnegative Vectors in Wireless Data Transmission.
J. Electr. Comput. Eng.(2023)
摘要
With the rapid development of 5G communication and wireless Internet of Things technology, the application of intelligent wearable devices based on wireless data transmission technology is becoming more and more popular. However, due to the bandwidth of wireless data transmission nodes and the power consumption of the device system, the use time and data storage of wearable devices are severely restricted. The compressed sensing (CS) technology has become an effective method to tackle this problem. CS technology includes data compressive sensing at the transmission end and data reconstruction at the receiving end. In this paper, we consider the reconstruction of nonnegative sparse vectors, an important problem in the area of CS for wireless data transmission. It is known that the interval-passing (IP) algorithm is a low-complexity message-passing type method for the problem. However, the reconstruction performance of the IP algorithm is inferior to that of the other state-of-the-art CS reconstruction algorithms such as the orthogonal matching pursuit (OMP) algorithm. In order to address the problem, we propose a two-stage reconstruction algorithm in this paper. The proposed algorithm applies the IP algorithm for the first stage of reconstruction. If the reconstruction fails, the OMP algorithm is then used on the basis of the results in the first stage. The proposed algorithm is evaluated and compared with other state-of-the-art algorithms by the probability of perfect reconstruction under the given sparsity order value. Simulation results suggest that the proposed two-stage algorithm can greatly improve the reconstruction performance of the IP algorithm and can even outperform the OMP algorithm. In addition, the low-complexity advantages of the IP algorithm are maintained in the proposed algorithm.
更多查看译文
关键词
nonnegative vectors
AI 理解论文
溯源树
样例
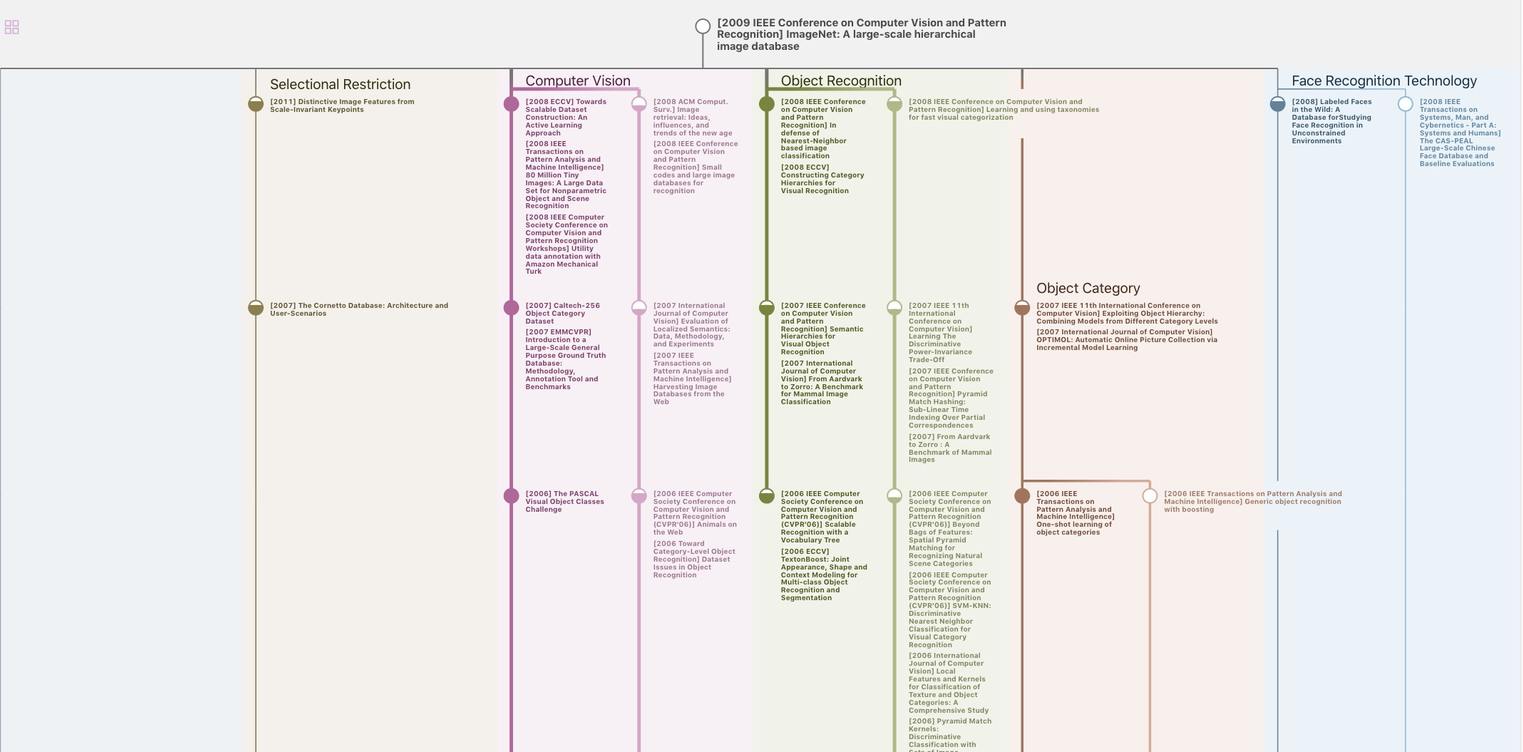
生成溯源树,研究论文发展脉络
Chat Paper
正在生成论文摘要