ExpertNet: Defeat noisy labels by deep expert consultation paradigm for pneumoconiosis staging on chest radiographs.
Expert Syst. Appl.(2023)
摘要
The pneumoconiosis staging task suffers from the stage ambiguity, especially in the early stage of pneumo-coniosis. The inter-stage reader variability caused by the stage ambiguity and the resulting noisy labels are even more detrimental to the learning of deep neural networks. In this article, we propose a novel deep expert consultation paradigm named ExpertNet to overcome the challenges caused by noisy labels in pneumoconiosis dataset collected from clinical diagnostic reports. The triple-network architecture of ExpertNet simulates an expert consultation group that provides reliable diagnostic suggestions from multiple perspectives. The novel quaternary sample partitioning strategy designed for ExpertNet is inspired by the fact that both certified radiologists and deep networks are troubled by hard samples, where the features are not fully correlated to their labels. The experiments confirm that ExpertNet outperforms the classical methods, which achieves an Accuracy of 89%, a Precision of 81.3%, a Sensitivity of 79.5%, an F1-score of 80.1%, and an AUC of 95.5%. Furthermore, analysis of the confusion matrix on the test set proves that ExpertNet is indeed very similar to an expert consultation group and effectively addresses the key challenge of hard-to-recognize early pneumoconiosis in clinical practice.
更多查看译文
关键词
pneumoconiosis staging,deep expertnet consultation paradigm,noisy labels
AI 理解论文
溯源树
样例
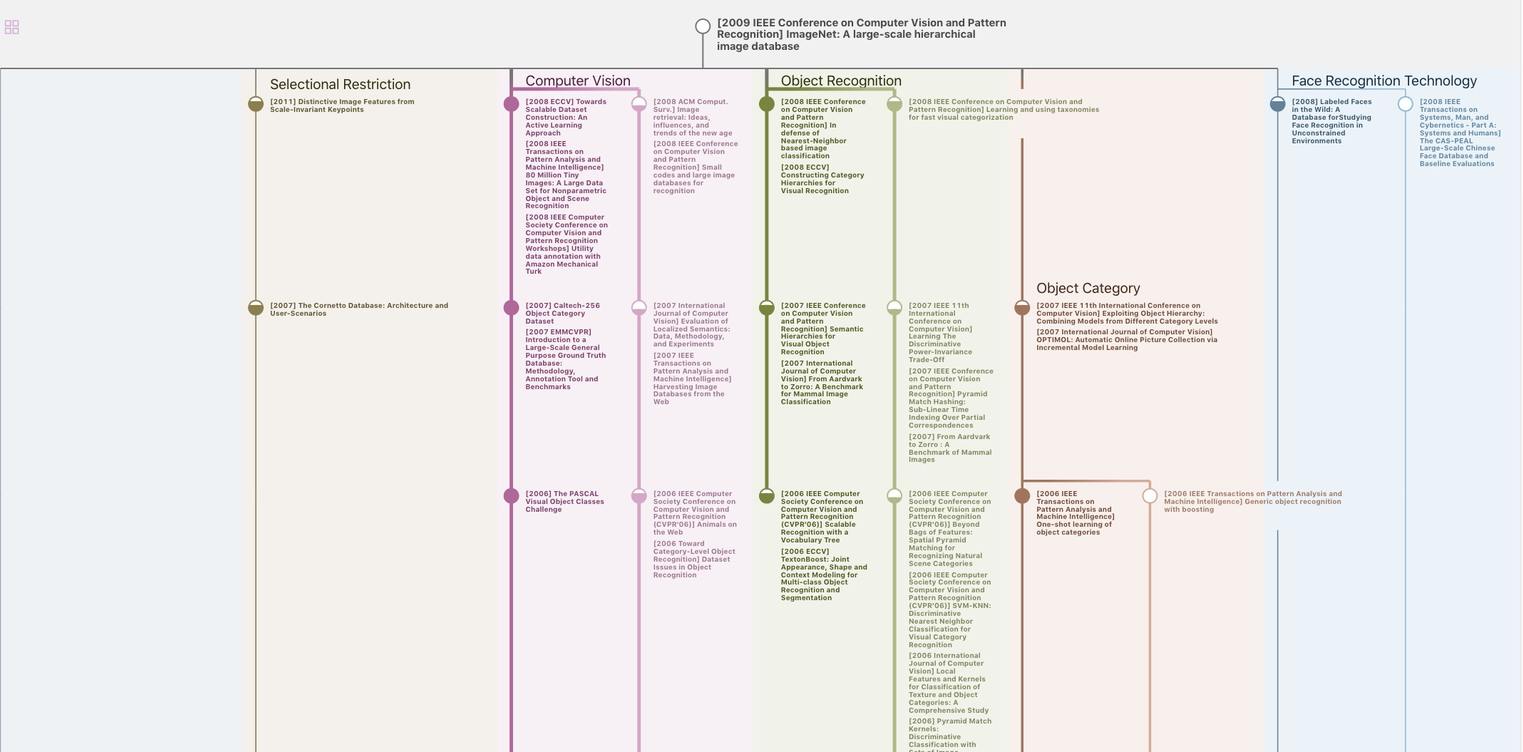
生成溯源树,研究论文发展脉络
Chat Paper
正在生成论文摘要