A Novel Local Feature Descriptor and an Accurate Transformation Estimation Method for 3-D Point Cloud Registration
IEEE Trans. Instrum. Meas.(2023)
摘要
Point cloud registration plays an important role in 3-D computer vision. Local feature-based registration as a kind of effective and robust method has two critical steps: descriptor generation and transformation estimation. This article proposes a novel local feature descriptor termed local normal deviation statistic histogram (LNDSH) and an accurate transformation estimation method named two-point-based sample consensus with compatibility ranking (2SAC-CR). Our LNDSH is generated on a local reference axis (LRA), and fully encodes geometric and spatial information by six attributes in which a new attribute named mean normal deviation value (mndv) is proposed. mndv encodes mean normal deviation at each point, which is not influenced by the error of an LRA. In 2SAC-CR, an effective compatibility ranking is first conducted to increase the possibility of capturing correct correspondences. Then, an LRA-based hypothesis generation and a novel hypothesis verification strategy are alternately implemented based on the ranking to ensure an accurate and efficient “hypothesis generation and verification.” Finally, the maximum consensus is used to generate the output transformation, further reducing the error of the result. Extensive experiments conducted on six standard datasets verify that LNDSH has high descriptiveness and strong robustness, and 2SAC-CR possesses high accuracy and strong robustness. Rigorous comparisons with the state-of-the-art show the overall superiority of our methods.
更多查看译文
关键词
3-D transformation estimation,local feature descriptor,local reference axis (LRA),point cloud,point cloud registration
AI 理解论文
溯源树
样例
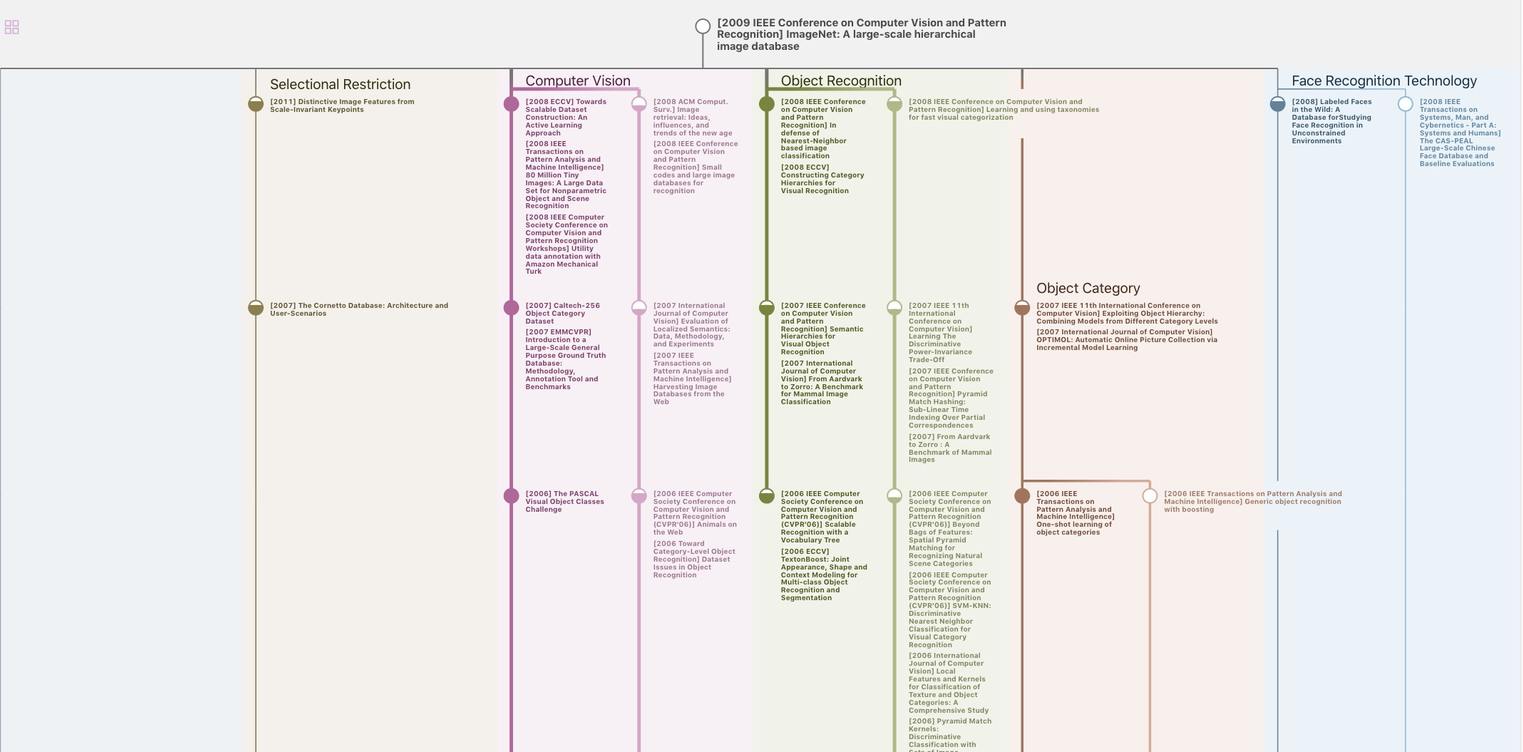
生成溯源树,研究论文发展脉络
Chat Paper
正在生成论文摘要