Random Forest-Based Online Detection and Location of Internal Short Circuits in Lithium Battery Energy Storage Systems With Limited Number of Sensors.
IEEE Trans. Instrum. Meas.(2023)
摘要
For fault detection in energy storage systems, the current topologies and detection methods require a large number of sensors. Therefore, this article proposes a random forest (RF)-based online detection and localization method to monitor faulty cells in lithium battery energy storage systems. First, the internal short circuit (ISC) is diagnosed by combining voltage and current signals to reduce the number of sensors used for monitoring. The feature extraction method proposed in this article requires at most 2M + 1 sensors in the N x M topology, which can reduce the use of M(N - 2) - 1 sensors compared to current research methods. Then, using RF to process the extracted features reduces the detection and localization time. Finally, constant-current discharge 100-Omega ISC resistance data are used to train the RF model, and different ISC resistance data for New European Driving Cycle (NEDC) conditions are used to verify the accuracy and precision of the trained RF model. The results show that the topology optimization and algorithm proposed in this article can use simple sample data of constant-current discharge to train the algorithm and monitor fault information and location in milliseconds during practical applications.
更多查看译文
关键词
Energy storage system,fault diagnosis,feature extraction,internal short circuit (ISC),random forest (RF)
AI 理解论文
溯源树
样例
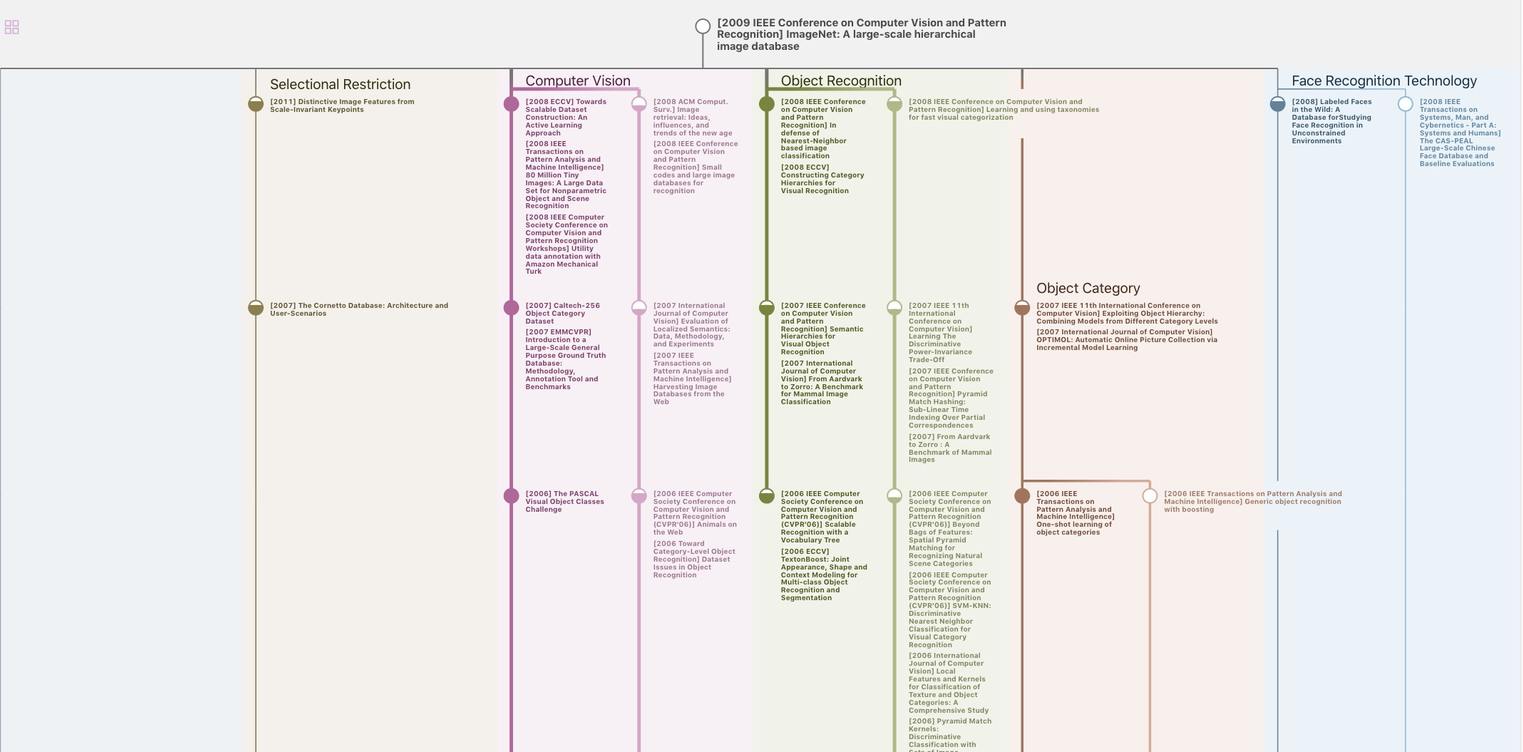
生成溯源树,研究论文发展脉络
Chat Paper
正在生成论文摘要