Generic Dynamic Graph Convolutional Network for traffic flow forecasting
Inf. Fusion(2023)
摘要
In the field of traffic forecasting, methods based on Graph Convolutional Network (GCN) are emerging. But existing methods still have limitations due to insufficient sharing patterns, inflexible temporal relations and static relation assumptions. To address these issues, a Generic Dynamic Graph Convolutional Network (GDGCN) for traffic flow forecasting is proposed. A generic framework with both parameter-sharing and independent blocks across stacked layers is proposed to explore parameter sharing systematically in all data dimensions, which can exploit distinct patterns from layer to layer and stable patterns across layers simultaneously. Then, we design a novel temporal graph convolutional block to view historical time slots as nodes in graph perspective and handle temporal dynamics with graph convolution. This temporal convolutional block can capture flexible and global temporal relations to have a better understanding of current traffic conditions. Lastly, a dynamic graph constructor is proposed to model not only the time-specific spatial dependencies between nodes, but also the changing temporal interactions between time slots to discover dynamic relations from data thoroughly. Experimental results on four real-world datasets show that GDGCN not only outperforms state-of-the-art methods, but also obtains interpretable dynamic spatial relations of segments. Codes are available at https://anonymous.4open.science/r/GDGCN.
更多查看译文
关键词
Traffic forecasting,Graph Convolutional Network,Temporal graph convolution,Parameter sharing
AI 理解论文
溯源树
样例
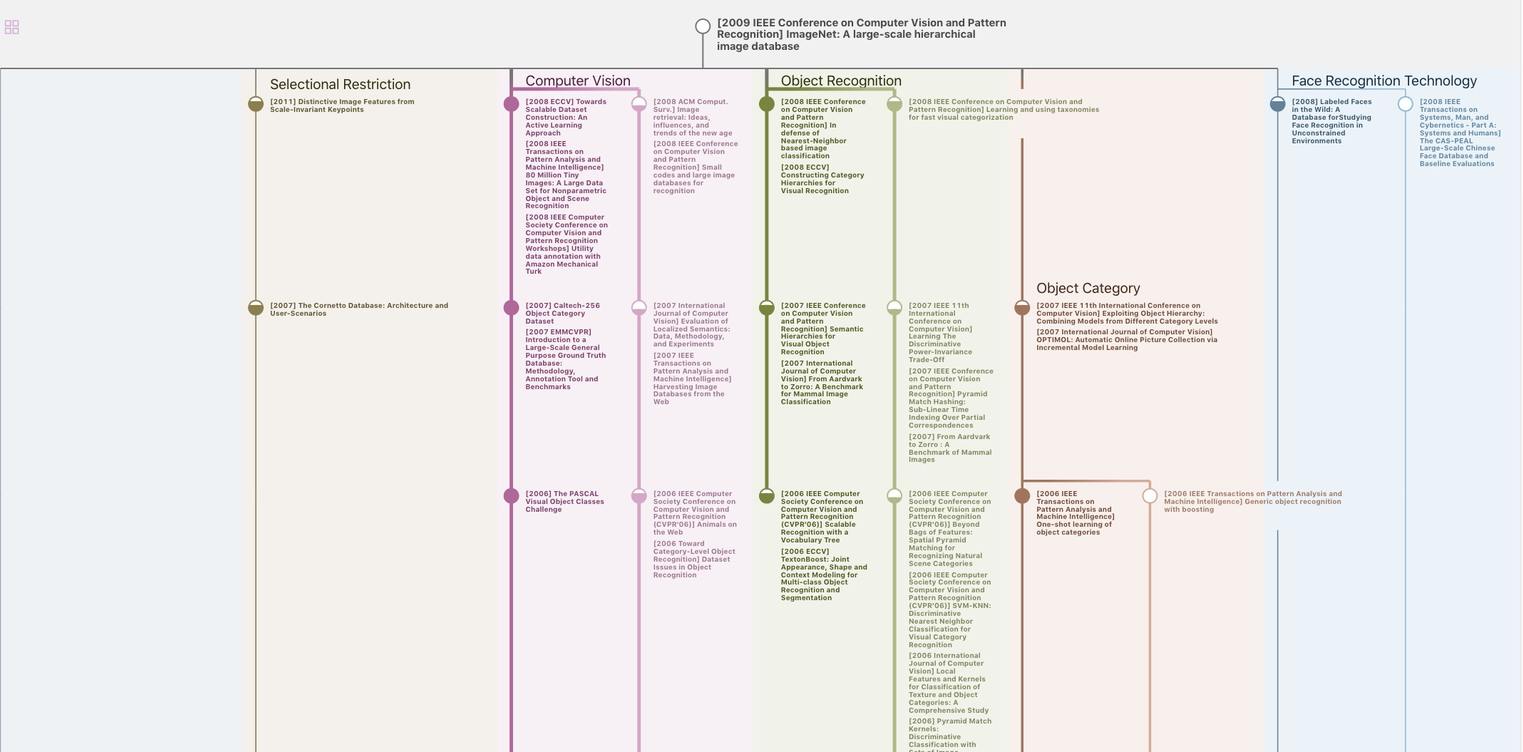
生成溯源树,研究论文发展脉络
Chat Paper
正在生成论文摘要