Sparse kernel k-means for high-dimensional data
Pattern Recognit.(2023)
摘要
The kernel k-means method usually loses its power when clustering high-dimensional data, due to a large number of irrelevant features. We propose a novel sparse kernel k-means clustering (SKKM) to extend the advantages of kernel k-means to the high-dimensional cases. We assign each feature a 0-1 indicator and optimize an equivalent kernel k-means loss function while penalizing the sum of the indicators. An alternating minimization algorithm is proposed to estimate both the class labels and the feature indicators. We prove the consistency of both clustering and feature selection of the proposed method. In addition, we apply the proposed framework to the normalized cut. In the numerical experiments, we demonstrate that the proposed method provides better/comparable performance compared to the existing high-dimensional clustering methods.
更多查看译文
关键词
Clustering,Feature selection,Kernel method
AI 理解论文
溯源树
样例
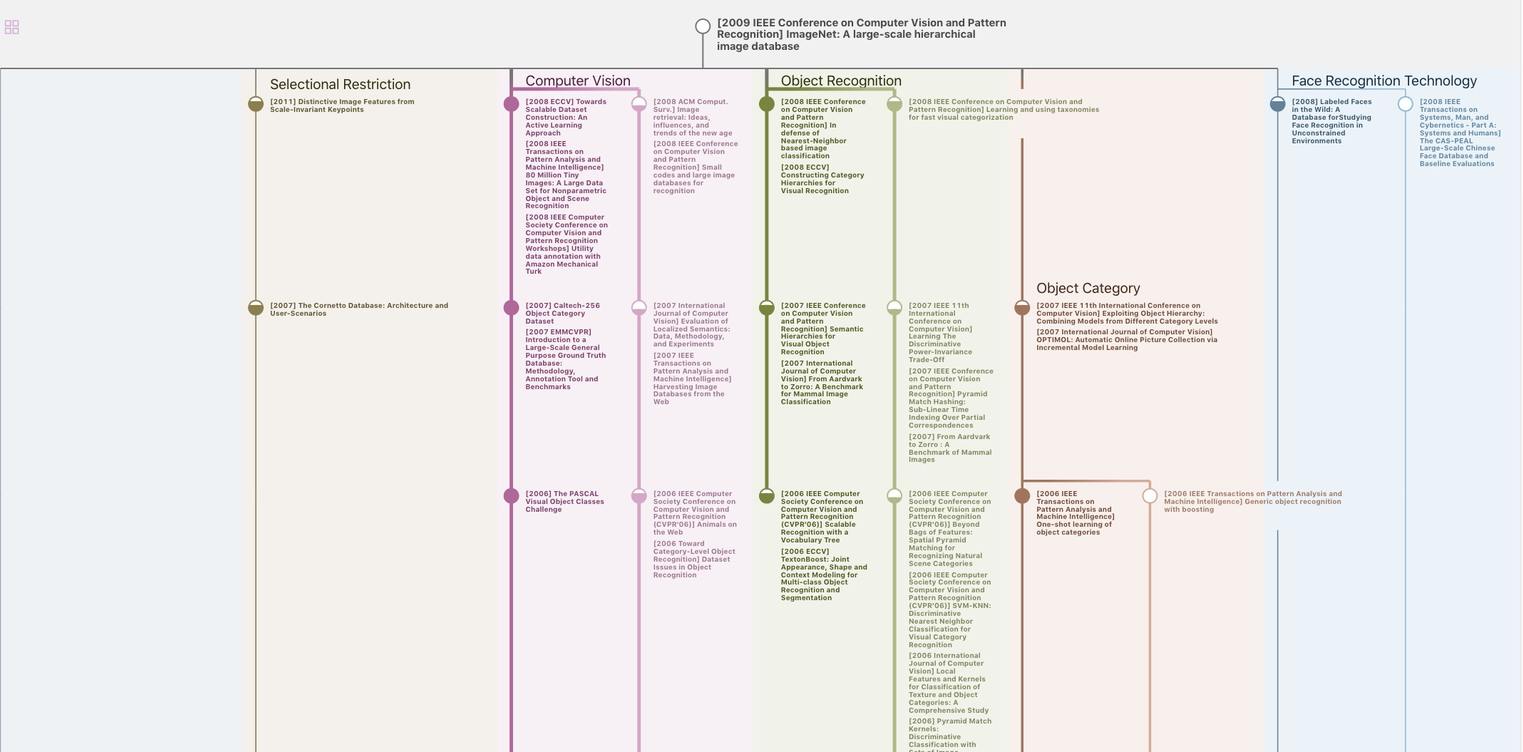
生成溯源树,研究论文发展脉络
Chat Paper
正在生成论文摘要