A Minimal Gated Multi-Modal Unit for Sensor Fusion in Insurance Telematics.
IEEE Access(2023)
摘要
Insurance Telematics is a recent service that is offered to drivers where a sensing platform measures vehicle's dynamics with the ultimate goal of inferring the level of risk that the driver experiences on the road. For this to happen, a robust set of sensors must be deployed within the vehicle and effective algorithms to process and classify driving patterns must be employed. As feasible as it looks, however, insurance telematics poses a great challenge when sensors offer information that is redundant or even show a low signal/noise ratio. The question here, then, is how to identify what sensing information is really useful and discard that one that only adds overhead. In this study we proposed an algorithmic approach, called minimal Gated Multimodal Unit (mGMU) that learns to filter out the proper amount of data from different input sources, making it an appealing choice for data fusion scenarios. We evaluate mGMU on three public multi modal (multiple sensor) datasets, created to classify driving styles, that comprise 3, 3 and 8 sensor sources. After a very extensive experimental setup that comprised around 30 different pipelines (made of feature extractors, information fusion approaches and a classification layer), results show that configurations based on mGMU obtain the best performance (with an increase of 2% with respect the second best approach and even outperforming the use of AutoEncoders by 5%). Moreover, as a proof of concept we deployed mGMU on three commercial smartphones, thus showing its efficiency given the number of parameters and FLOPS that were dramatically reduced. Given these results, we expect mGMU to be of great use on resource-constrained edge computing devices and rich sensor scenarios where data from different data sources must be exploited.
更多查看译文
关键词
Insurance telematics, aggressive driving, driving style classification, gated multi-modal unit, risky driving, sensor fusion
AI 理解论文
溯源树
样例
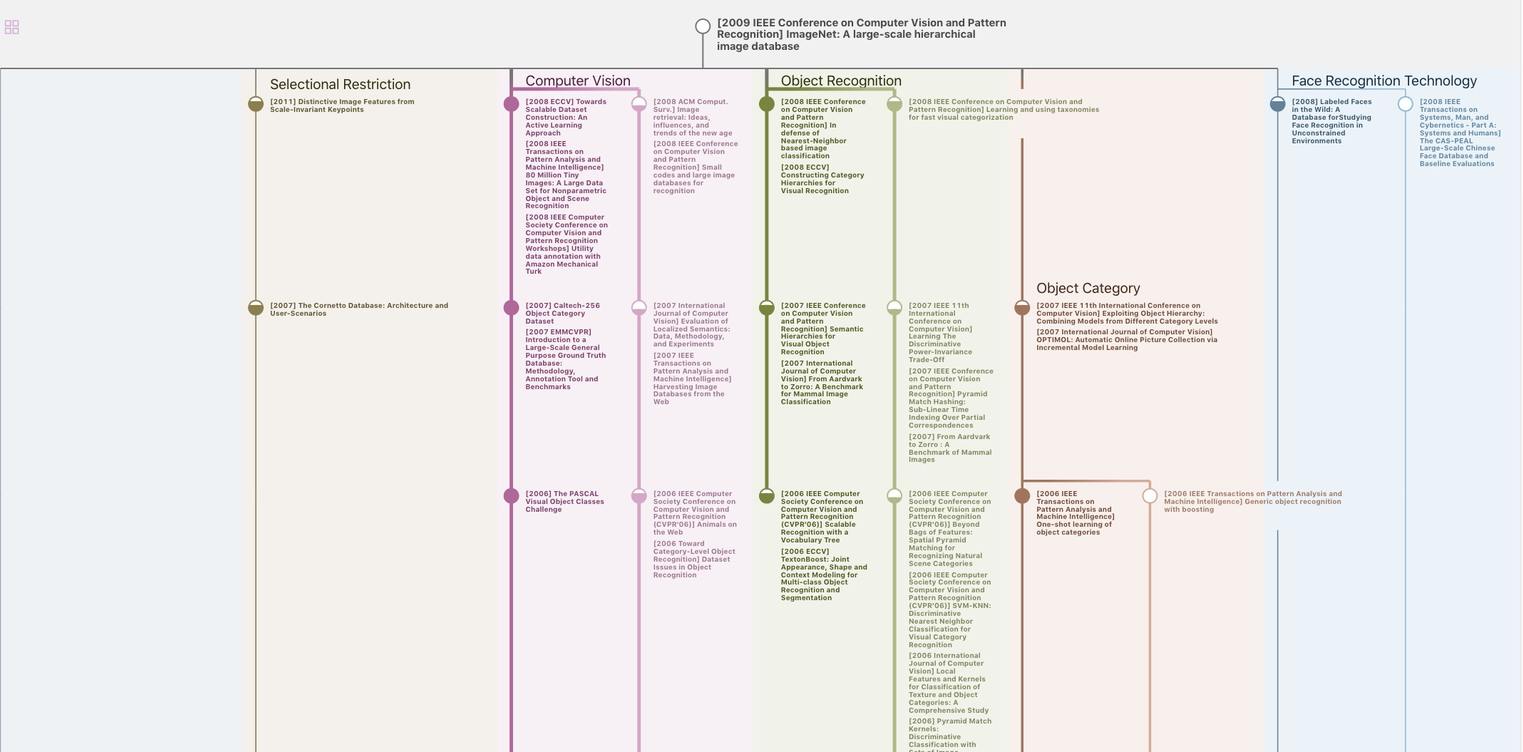
生成溯源树,研究论文发展脉络
Chat Paper
正在生成论文摘要