CrossDomain Recommendation Based on MetaData Using Graph Convolution Networks.
IEEE Access(2023)
摘要
Recent advancements in the domain of recommender systems have stemmed from the inspiration of representing the user-item interaction into graphs. These heterogeneous graphs comprehensively capture the non-linear relationships between users and items alongwith features and emneddings. Graph Convolution Networks (GCNs) are state-of-the-art graph-based learning models that learn and represent the graph structures by recursively stacking layers of convolution and non-linear activation operations. GCNs are augmented with the strength of deep learning paradigms resulting in achieving better performance as compared to traditional CF (Collaborative Filtering) methods. Despite modern improvements in the domain of recommender systems, cold-start users are a daunting challenge in the design of recommender systems since the conventional recommendation services are based on solely one data source. During the recent years, cross-domain recommendation methods have gained popularity because of the availability of information in multiple domains for cold start users. We supplement this information by utilizing the data contained in the metadata of users alongwith the strength of modelling graphs using GCNs. Our proposed algorithm seams the strength of GCNs with cross-domain paradigm utilizing the richness in metadata in user's feedback to overcome the sparsity in user-item rating matrix. The combined advantages of GCNs and cross-domain approaches alleviated the issues of cold-start users by transferring user preferences from a source domain to a target domain.
更多查看译文
关键词
graph convolution networks,metadata
AI 理解论文
溯源树
样例
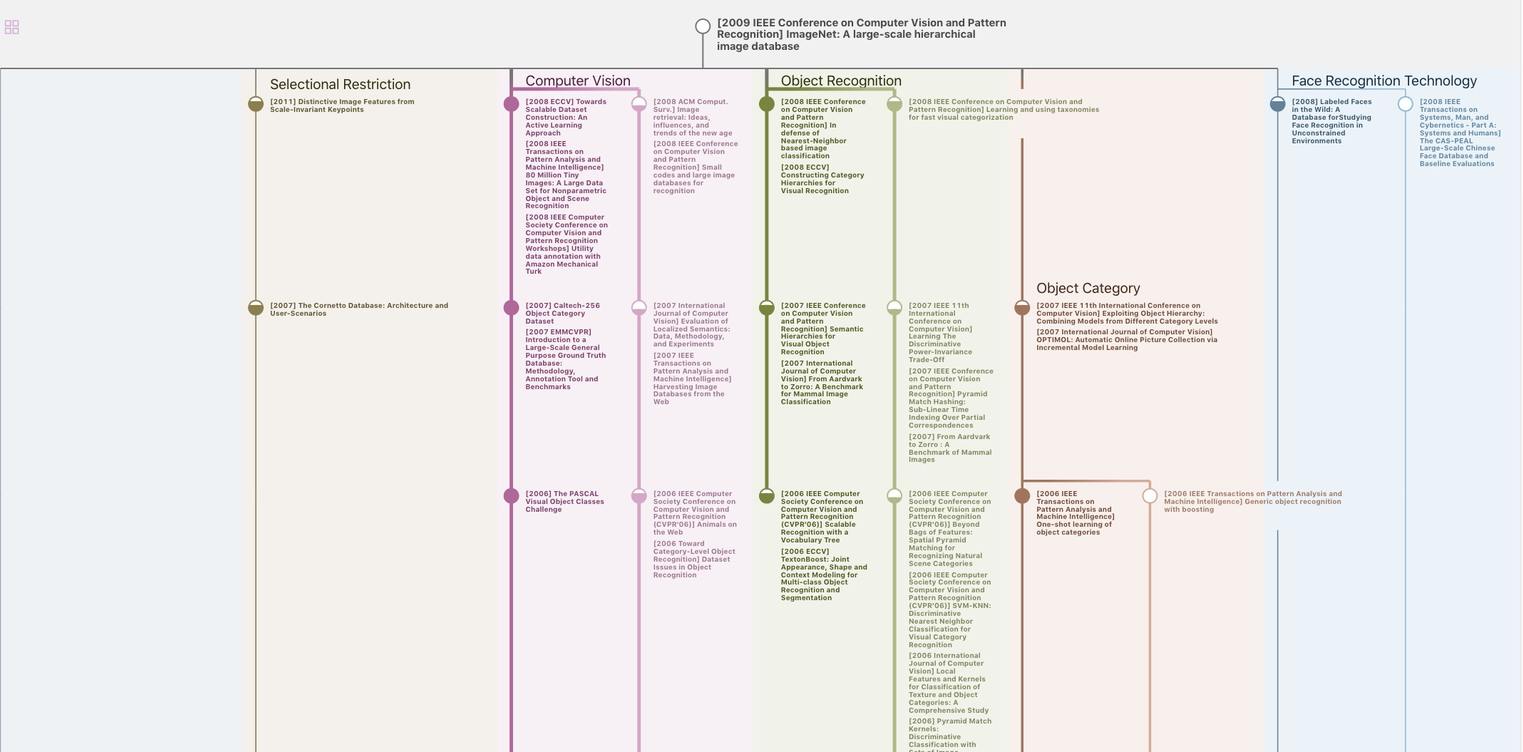
生成溯源树,研究论文发展脉络
Chat Paper
正在生成论文摘要