Enhanced Parkinson's Disease Diagnosis Through Convolutional Neural Network Models Applied to SPECT DaTSCAN Images
IEEE Access(2023)
摘要
Convolutional Neural Networks (CNNs) are highly regarded in Deep Learning (DL) and have shown promising results in medical image analysis, making them a leading model for Computer-Aided Diagnosis (CAD) systems. Their efficacy extends to the diagnosis of neurological disorders, including Parkinson's Disease (PD), which is typically identified through Single Photon Emission Computed Tomography (SPECT) scans. However, relying solely on visual inspection of SPECT images during medical examinations can introduce inaccuracies due to subjective factors. We propose a CAD system for automatic PD diagnosis using pre-trained CNN models, Transfer Learning (TL) technique, and the Bilinear Pooling method to address this issue. The study employs several CNN architectures, specifically Efficient-Net B0, and Mobile-Net V2 models, and a custom CNN architecture. These pre-trained architectures were originally trained on ImageNet and adapted to the current task using the TL technique. These architectures are leveraged with a bilinear pooling form, resulting in three Bilinear CNN (BCNN) models. These models are applied to pre-processed SPECT image data of PD patients and Healthy Controls (HC), categorized into three distinct datasets. The proposed method is evaluated on a total of 2720 SPECT images (1360 PD and 1360 HC subjects) obtained from the Parkinson's Progression Marker Initiative (PPMI) dataset. The findings show that the BCNN EfficientNet-B0-MobileNet-V2 model achieved the highest accuracy score of 98.47%, surpassing other developed CNN models and outperforming existing methods. In conclusion, the proposed CAD system provides an efficient diagnostic tool to assist physicians in making accurate PD diagnoses, independent of subjective factors.
更多查看译文
关键词
Bilinear pooling,convolutional neural networks,deep learning,Parkinson's disease,SPECT
AI 理解论文
溯源树
样例
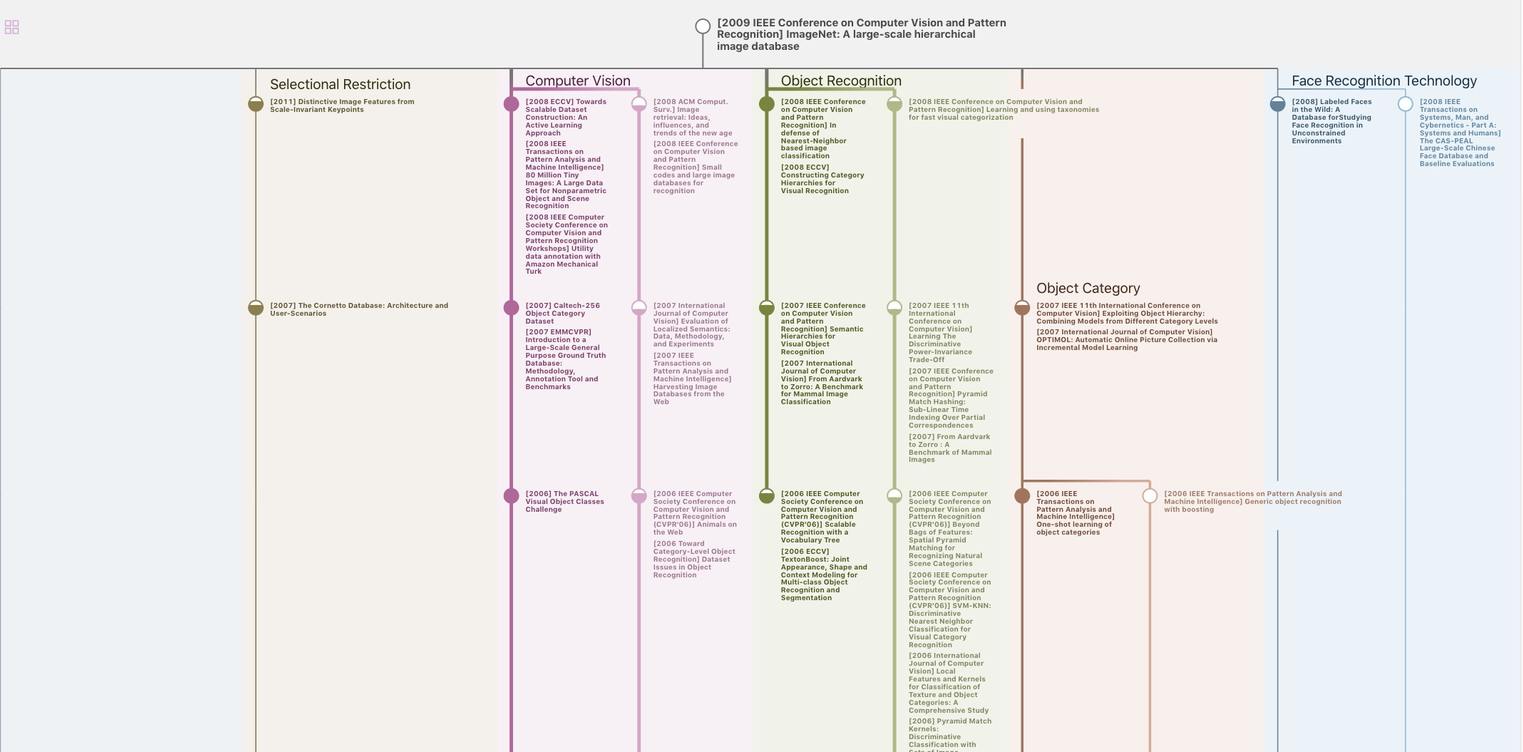
生成溯源树,研究论文发展脉络
Chat Paper
正在生成论文摘要