HAT: A Visual Transformer Model for Image Recognition Based on Hierarchical Attention Transformation.
IEEE Access(2023)
摘要
In the field of image recognition, Visual Transformer (ViT) has excellent performance. However, ViT, relies on a fixed self-attentive layer, tends to lead to computational redundancy and makes it difficult to maintain the integrity of the image convolutional feature sequence during the training process. Therefore, we proposed a non-normalization hierarchical attention transfer network (HAT), which introduces threshold attention mechanism and multi head attention mechanism after pooling in each layer. The focus of HAT is shifted between local and global, thus flexibly controlling the attention range of image classification. The HAT used the smaller computational complexity to improve it's scalability, which enables it to handle longer feature sequences and balance efficiency and accuracy. HAT removes layer normalization to increase the likelihood of convergence to an optimal level during training. In order to verify the effectiveness of the proposed model, we conducted experiments on image classification and segmentation tasks. The results shows that compared with classical pyramid structured networks and different attention networks, HAT outperformed the benchmark networks on both ImageNet and CIFAR100 datasets.
更多查看译文
关键词
Visual transformer, attention transfer mechanism, hierarchical network, image feature, image recognition
AI 理解论文
溯源树
样例
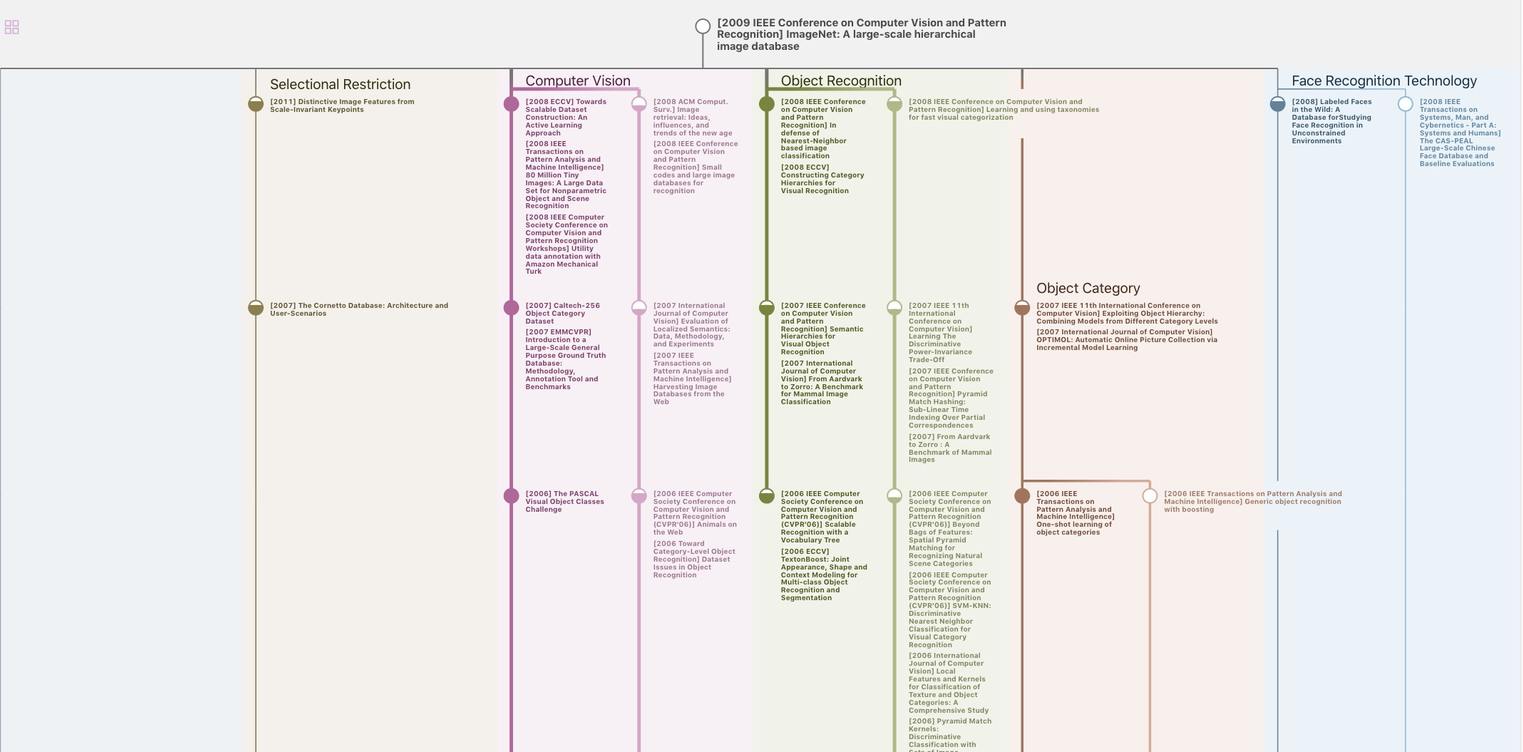
生成溯源树,研究论文发展脉络
Chat Paper
正在生成论文摘要