Semisupervised Building Instance Extraction From High-Resolution Remote Sensing Imagery.
IEEE Trans. Geosci. Remote. Sens.(2023)
摘要
Automatic building instance extraction from high-resolution (HR) remote sensing imagery (RSI) is crucial for urban planning and mapping. The dominant approaches are based on the full-supervised learning paradigm that requires a large number of labeled samples to train their models, which is very time-consuming and labor-intensive. To alleviate this problem, this study proposes a semisupervised building instance extraction method that integrates teacher–student learning and pseudo-labeling to improve the building instance extraction from HR RSI. Specifically, the proposed method consists of three modules, the hybrid data augmentation (HDA) module, the pseudo-label generation (PLG) module, and the contour refinement (CR) module. The HDA module is designed to enrich the diversity of labeled samples to optimize the teacher model. The PLG module generates pseudo-labels from unlabeled data to train a student model with pseudo-labels. Finally, the CR module is designed to refine the contours of buildings. Experimental results on three challenging public datasets demonstrate that the proposed method achieves superior performance and exhibits great robustness at different proportions of labeled data and different building scenarios. This study provides a new approach for extracting building instances from HR RSI in scenarios with insufficient labeled samples and a methodological reference for various applications of semisupervised on RSIs.
更多查看译文
关键词
Building instance extraction,high-resolution (HR) remote sensing imagery (RSI),pseudo-label,semisupervised
AI 理解论文
溯源树
样例
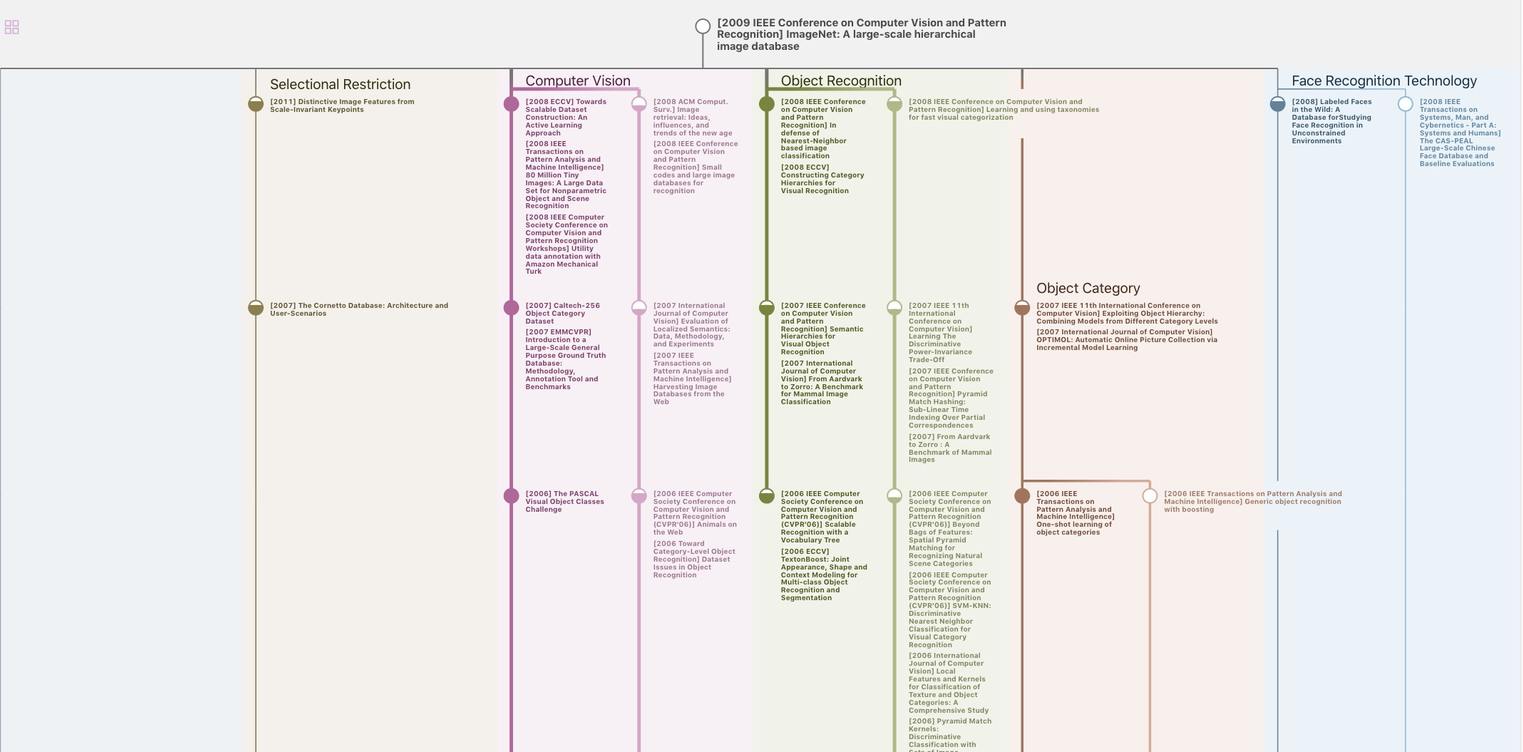
生成溯源树,研究论文发展脉络
Chat Paper
正在生成论文摘要