DGPF-RENet: A Low Data Dependence Network With Low Training Iterations for Hyperspectral Image Classification
IEEE Trans. Geosci. Remote. Sens.(2023)
摘要
The classification of ground objects from hyperspectral images (HSIs) is of great importance for human perception of information about the terrain and landscape. HSIs have numerous dimensions, and obtaining the data is difficult. The issue of slow convergence of neural network training is brought on by high-dimensional data, and the neural network's performance is impacted by the challenging data acquisition process. In order to achieve the effects of low data dependence and rapid convergence, we propose a redundancy elimination network architecture with a decoupled-gaze attention mechanism and phantom fractal modules (DGPF-RENet) for HSI classification. First, we propose the decoupled-gaze attention (DGA) mechanism to make full use of the correlation between adjacent bands and the continuity of neighboring pixels in HSIs. Then, a redundancy elimination module (REM) is proposed to reduce the number of feature points and eliminate redundant information while preserving the contextual information and relationships between pixels. Finally, the phantom fractal module (PFM) is proposed, which improves the scale of feature learning by fractalizing convolutions at multiple scales. Four publicly available HSIs datasets, including Indian Pines, Salinas, DFC2018, and WHUHi-HongHu, were used in our experiments. According to experimental findings, compared to other state-of-the-art methods, our method performs best with a small number of training samples and few iterations. We have released our code and models at https://github.com/yuhua666/DGPF-RENet.
更多查看译文
关键词
Attention,eliminate redundancy,feature learning,hyperspectral images (HSIs),image classification
AI 理解论文
溯源树
样例
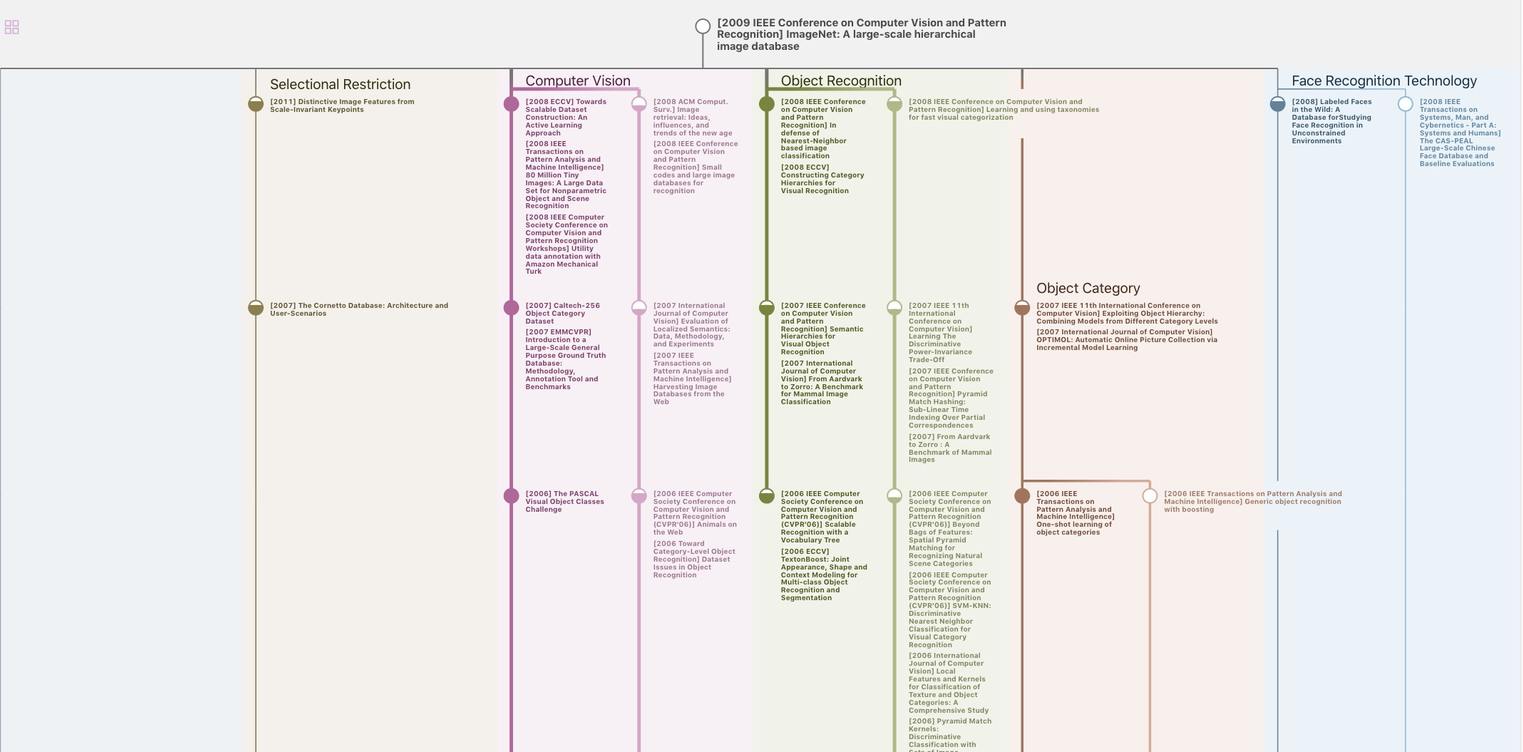
生成溯源树,研究论文发展脉络
Chat Paper
正在生成论文摘要