Focus nuance and toward diversity: exploring domain-specific fine-grained few-shot recognition
Neural Comput. Appl.(2023)
摘要
In real-world industrial applications, learning to recognize novel visual categories from a few samples is challenging and promising. Although some efforts have been made in the academic field for few-shot classification studies, there is still a lack of high-precision fine-grained few-shot classification models in some specific fields, especially in the fine-grained agricultural field. As far as we know, this study is the first work on meta-learning few-shot classification for fine-grained plant disease classification ( specific to disease severity ). We propose a multi-perspective hybrid attention meta-learning model based on a Batch Nuclear-norm constraint. The model explores discriminative features by focusing on key regions, and the hybrid attention module is divided into two sub-modules, soft attention model and patch-hard attention model. The discriminability and diversity constraint module is introduced in the loss function to constrain the Batch Nuclear-norm of the classification matrix, which improves the discriminative properties of the classification model and increases its diversity at the same time. In this paper, a large number of experiments have been carried out on multiple datasets. The experimental results demonstrate that our work has better performance than state-of-the-art models. It can be said that our work is a valuable supplement to the domain-specific industrial application models.
更多查看译文
关键词
focus nuance,diversity,recognition,domain-specific,fine-grained,few-shot
AI 理解论文
溯源树
样例
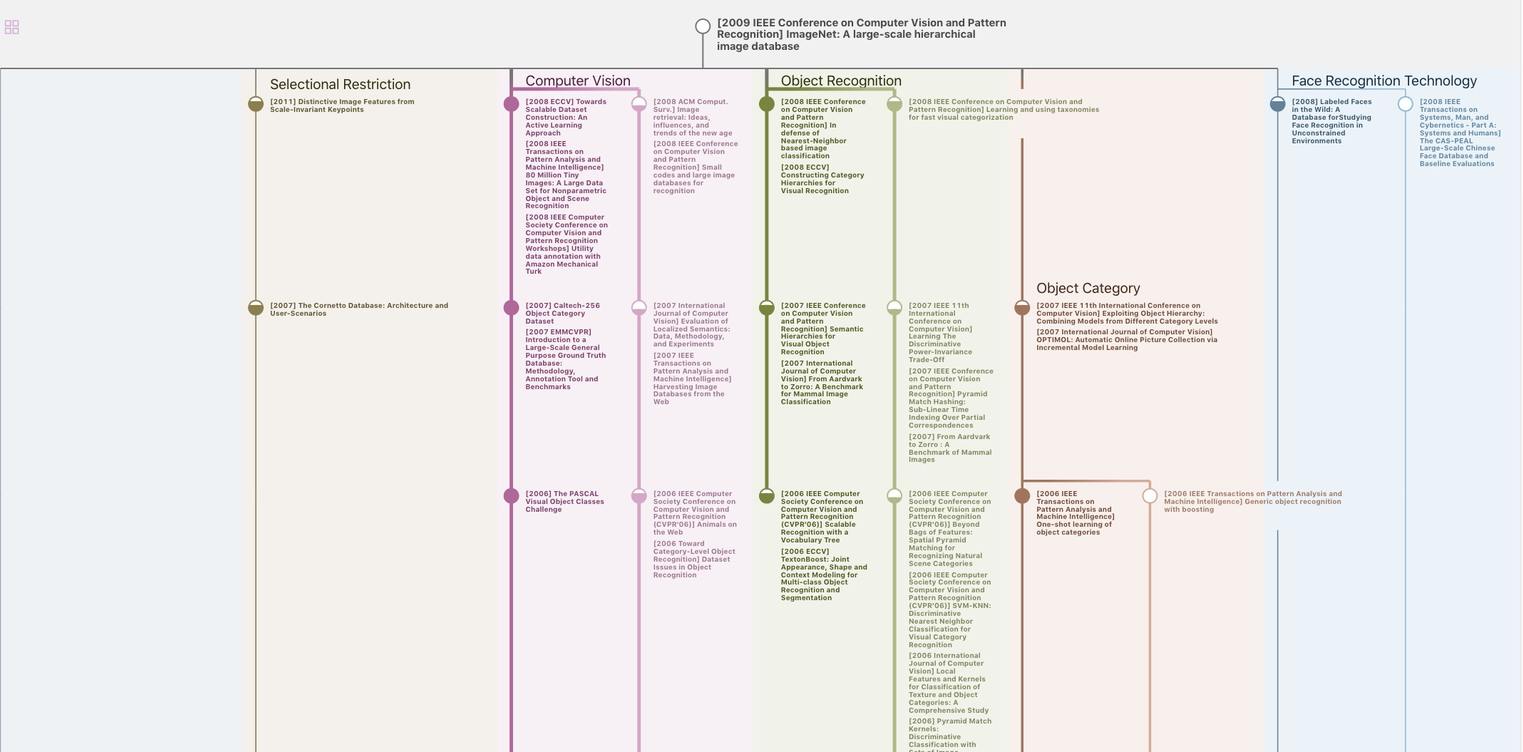
生成溯源树,研究论文发展脉络
Chat Paper
正在生成论文摘要