Reconfigurable Pedestrian Detection System Using Deep Learning for Video Surveillance.
IEICE Trans. Inf. Syst.(2023)
摘要
We present a reconfigurable deep learning pedestrian detection system for surveillance systems that detect people with shadows in different lighting and heavily occluded conditions. This work proposes a region-based CNN, combined with CMOS and thermal cameras to obtain human features even under poor lighting conditions. The main advantage of a reconfigurable system with respect to processor-based systems is its high performance and parallelism when processing large amount of data such as video frames. We discuss the details of hardware implementation in the proposed real-time pedestrian detection algorithm on a Zynq FPGA. Simulation results show that the proposed integrated approach of R-CNN architecture with cameras provides better performance in terms of accuracy, precision, and F1-score. The performance of Zynq FPGA was compared to other works, which showed that the proposed architecture is a good tradeoff in terms of quality, accuracy, speed, and resource utilization.
更多查看译文
关键词
quality, accuracy, speed, key deep learning, foreground detection, pedestrian detection, R-CNN, video surveillance
AI 理解论文
溯源树
样例
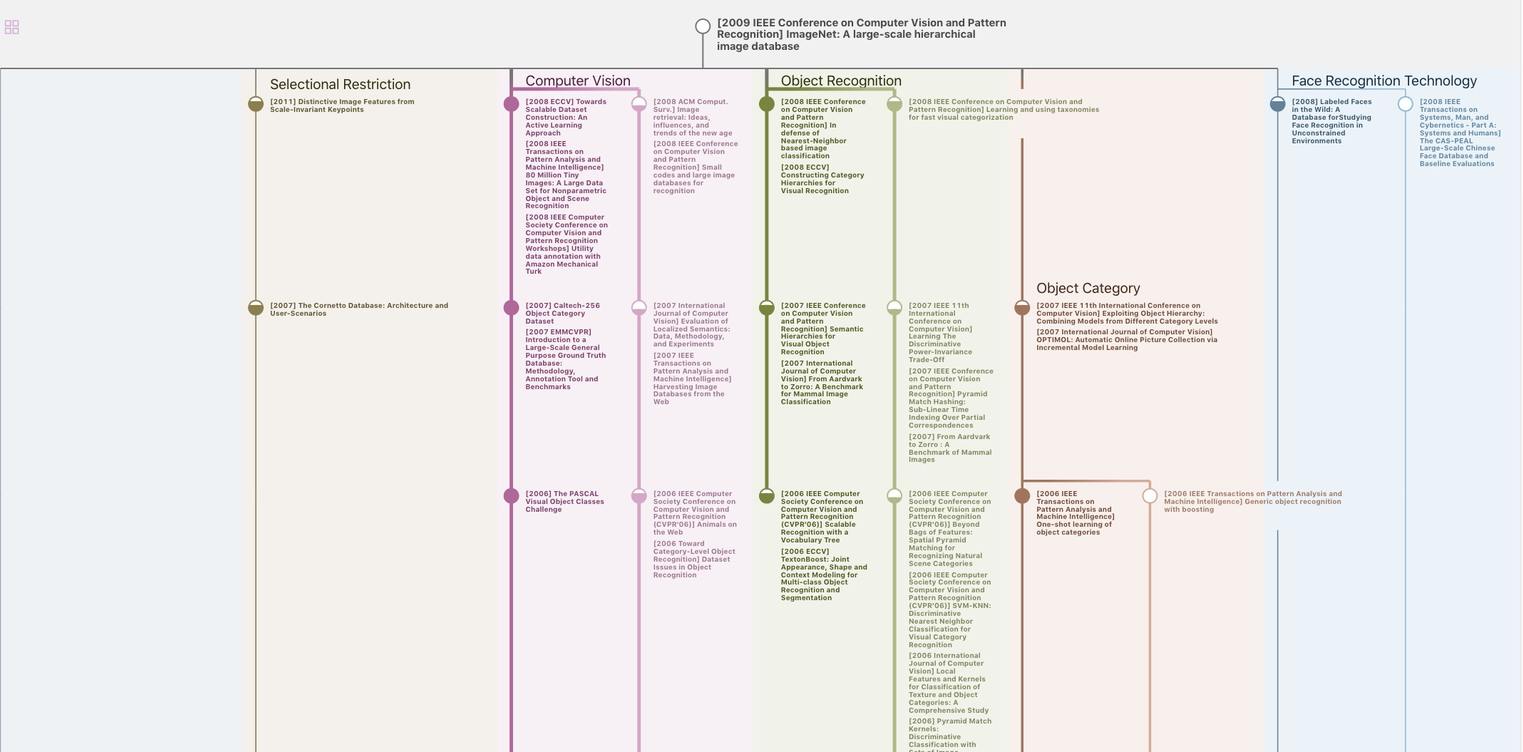
生成溯源树,研究论文发展脉络
Chat Paper
正在生成论文摘要