Out-of-distribution detection based on multi-classifiers.
Cogn. Comput. Syst.(2023)
摘要
Existing out-of-distribution detection models rely on the prediction of a single classifier and are sensitive to classifier bias, making it difficult to discriminate similar feature out-of-distribution data. This article proposed a multi-classifier-based model and two strategies to enhance the performance of the model. The model first trains several different base classifiers and obtains the predictions of the test data on each base classifier, then uses cross-entropy to calculate the dispersion between these predictions, and finally uses the dispersion as a metric to identify the out-of-distribution data. A large scatter implies inconsistency in the predictions of the base classifier, and the greater the probability of belonging to the out-of-distribution data. The first strategy is applied in the training process of the model to increase the difference between base classifiers by using various scales of Label smoothing regularisation. The second strategy is applied to the inference process of the model by changing the mean and variance of the activations in the neural network to perturb the inference results of the test data. These two strategies can effectively amplify the discrepancy in the dispersion of the in-distribution and out-of-distribution data. The experimental results show that the method in this article can effectively improve the performance of the model in the detection of different types of out-of-distribution data, improve the robustness of deep neural networks (DNN) in the face of unknown classes, and promote the application of DNN in systems and engineering with high security requirements.
更多查看译文
关键词
computer vision, machine learning
AI 理解论文
溯源树
样例
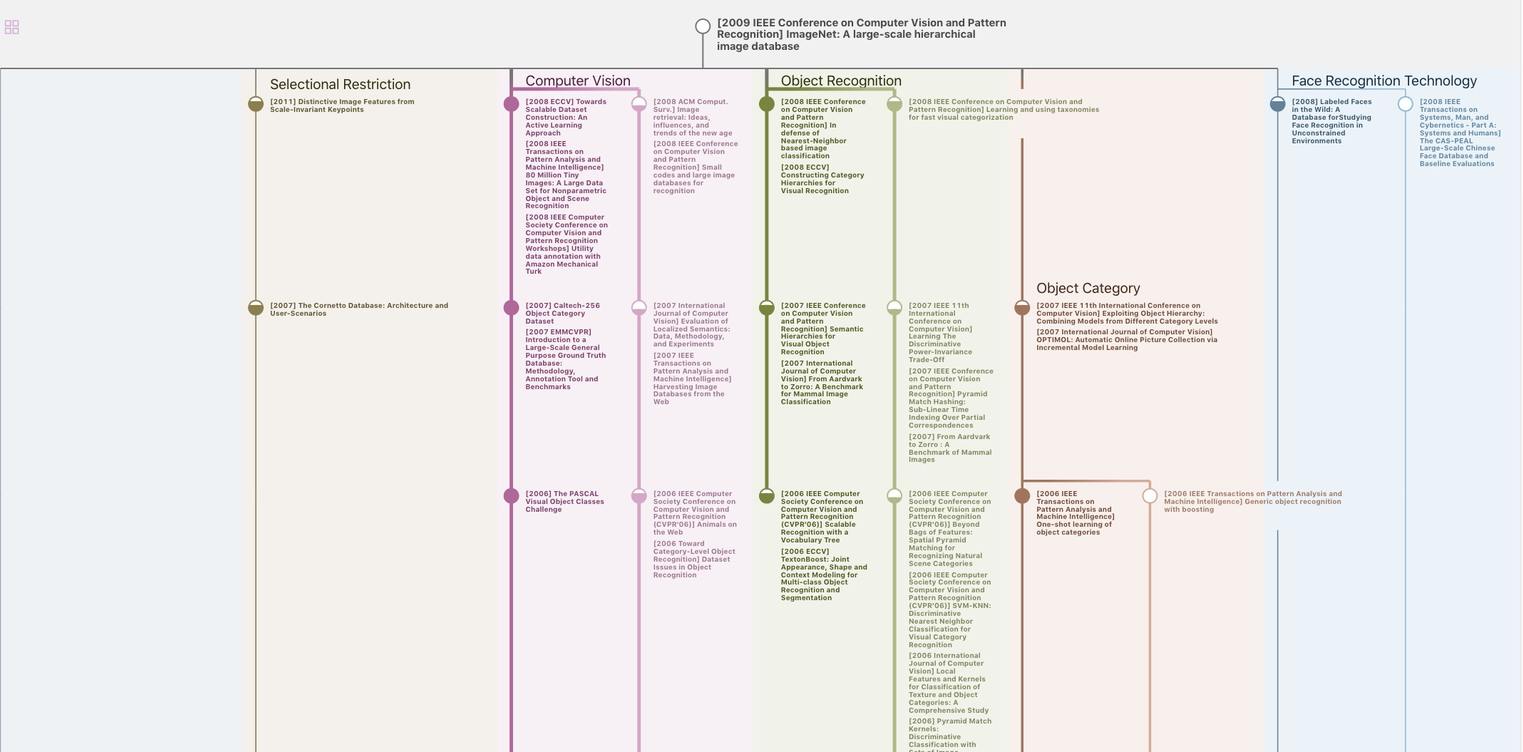
生成溯源树,研究论文发展脉络
Chat Paper
正在生成论文摘要