CNN Partitioning and Offloading for Vehicular Edge Networks in Web3
IEEE COMMUNICATIONS MAGAZINE(2023)
摘要
Web3, an emerging blockchain-based decentralized network, grants users ownership and enhances the collaboration among devices under monitoring. Benefiting from decentralization and in-memory computing, vehicular edge networks can process tasks such as road object detection distributedly without being attacked. Recently, to provide intelligent service for Web3 users, artificial intelligence applications have been booming, thus generating enormous deep learning models. These models are supposed to be deployed in the edge due to their massive computation. Further-more, edge servers may face overload and intolerable delay for the high concurrency of offloaded deep learning tasks. How to determine an optimal offloading decision in the highly dynamic and heterogeneous edge-cloud environment is still a challenge. To tackle the mentioned challenge, a dynamic offloading strategy based on game theory combined with convolutional neural network (CNN) partition for vehicular edge networks, named GPOV, is proposed. Specifically, CNN partition can utilize resources more efficiently and reduce the delay with parallelism. The game theoretic offloading decision strategy can determine the optimal offloading policy according to the real-time environment. The performance of our strategy is validated in the final part of this article.
更多查看译文
AI 理解论文
溯源树
样例
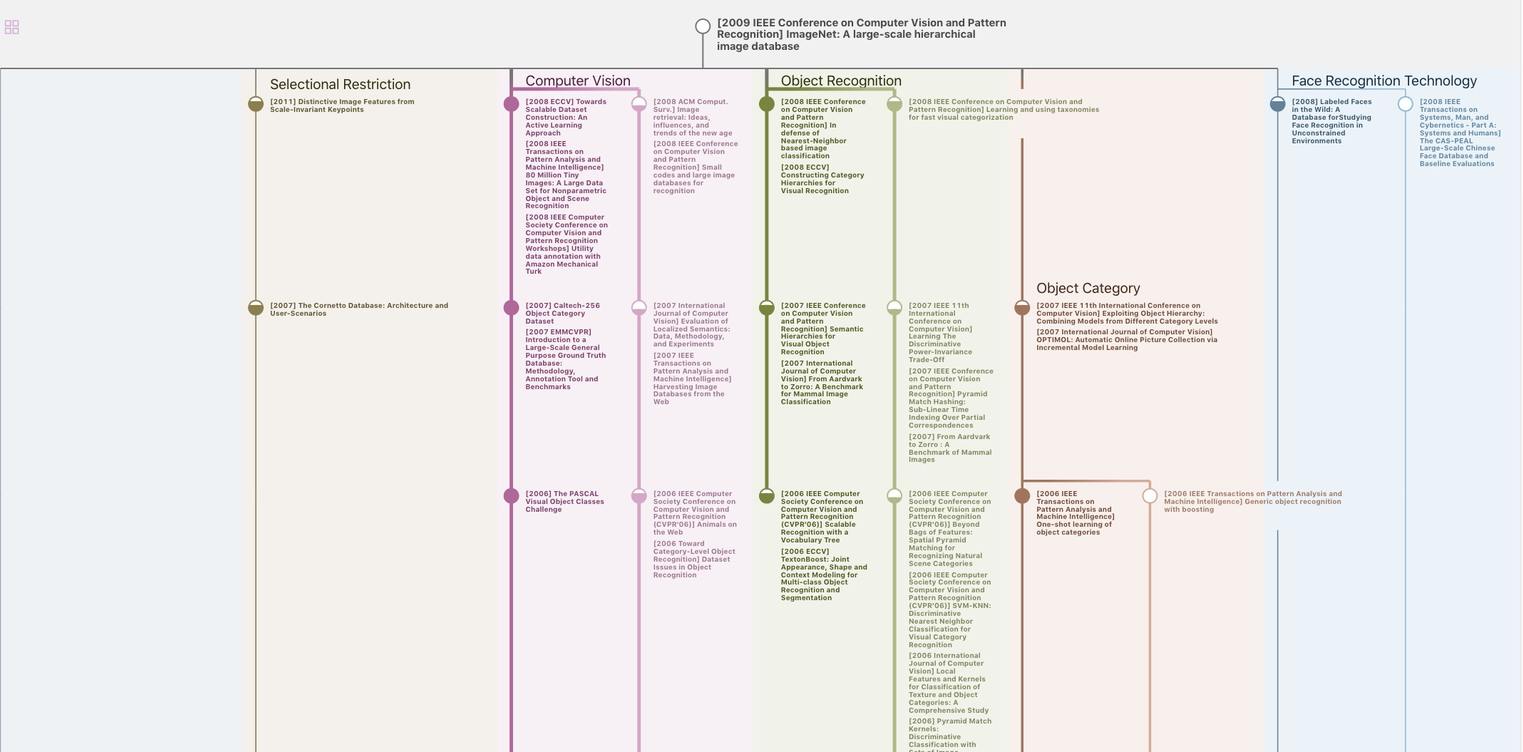
生成溯源树,研究论文发展脉络
Chat Paper
正在生成论文摘要