Deep reinforcement learning for solving resource constrained project scheduling problems with resource disruptions
ROBOTICS AND COMPUTER-INTEGRATED MANUFACTURING(2024)
摘要
The resource-constrained project scheduling problem (RCPSP) is encountered in many fields, including manufacturing, supply chain, and construction. Nowadays, with the rapidly changing external environment and the emergence of new models such as smart manufacturing, it is more and more necessary to study RCPSP considering resource disruptions. A framework based on reinforcement learning (RL) and graph neural network (GNN) is proposed to solve RCPSP and further solve the RCPSP with resource disruptions (RCPSP-RD) on this basis. The scheduling process is formulated as sequential decision-making problems. Based on that, Markov decision process (MDP) models are developed for RL to learn scheduling policies. A GNN-based structure is proposed to extract features from problems and map them to action probability distributions by policy network. To optimize the scheduling policy, proximal policy optimization (PPO) is applied to train the model end-to-end. Computational results on benchmark instances show that the RL-GNN algorithm achieves competitive performance compared with some widely used methods.
更多查看译文
关键词
Graph neural network,Reinforcement learning,Resource constrained project scheduling,problem with resource disruptions
AI 理解论文
溯源树
样例
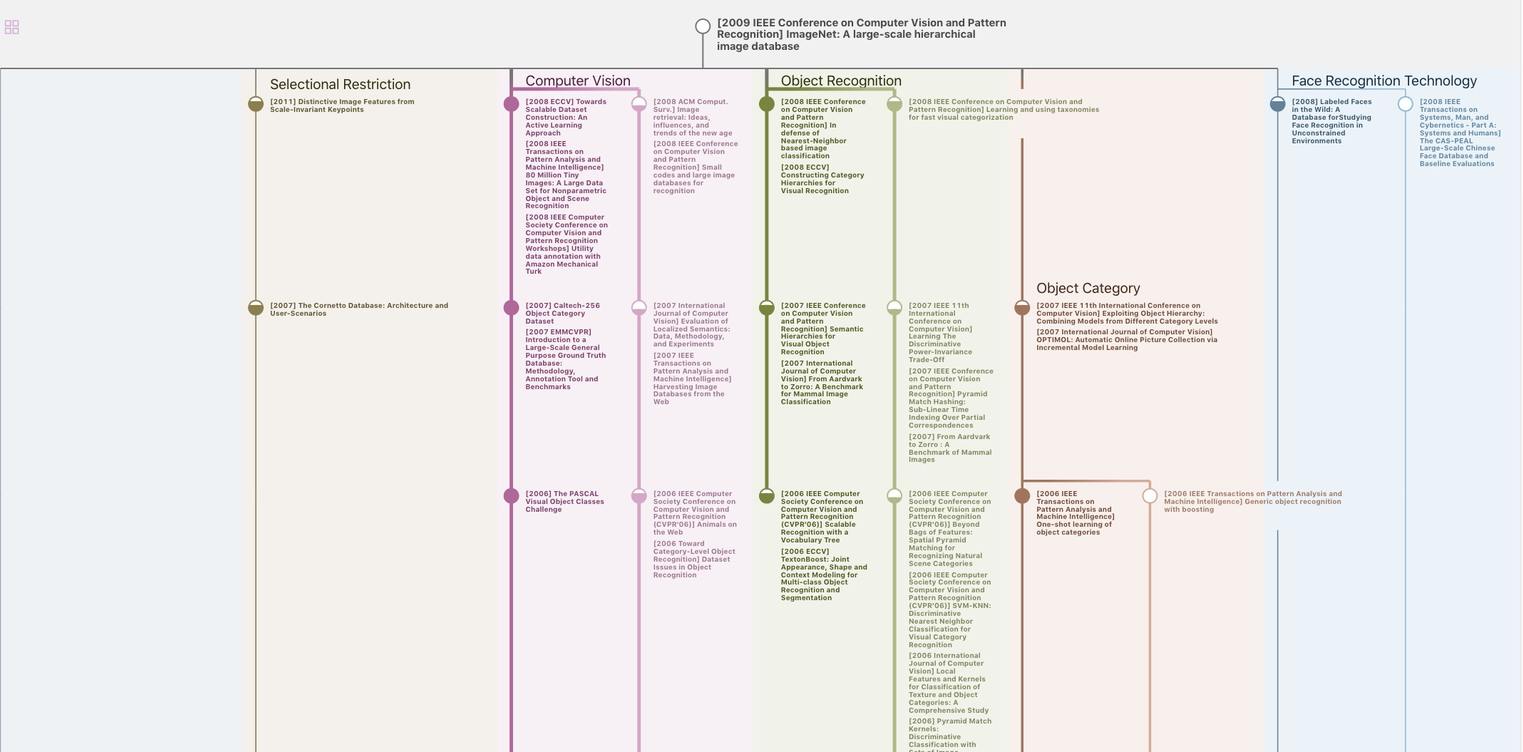
生成溯源树,研究论文发展脉络
Chat Paper
正在生成论文摘要