DBAFormer: A Double-Branch Attention Transformer for Long-Term Time Series Forecasting
Hum. Centric Intell. Syst.(2023)
摘要
The transformer-based approach excels in long-term series forecasting. These models leverage stacking structures and self-attention mechanisms, enabling them to effectively model dependencies in series data. While some approaches prioritize sparse attention to tackle the quadratic time complexity of self-attention, it can limit information utilization. We introduce a creative double-branch attention mechanism that simultaneously captures intricate dependencies in both temporal and variable perspectives. Moreover, we propose query-independent attention, taking into account the near-identical attention allocated by self-attention to different query positions. This enhances efficiency and reduces the impact of redundant information. We integrate the double-branch query-independent attention into popular transformer-based methods like Informer, Autoformer, and Non-stationary transformer. The results obtained from conducting experiments on six practical benchmarks consistently validate that our novel attention mechanism substantially improves the long-term series forecasting performance in contrast to the baseline approach.
更多查看译文
关键词
forecasting,attention,transformer,double-branch,long-term
AI 理解论文
溯源树
样例
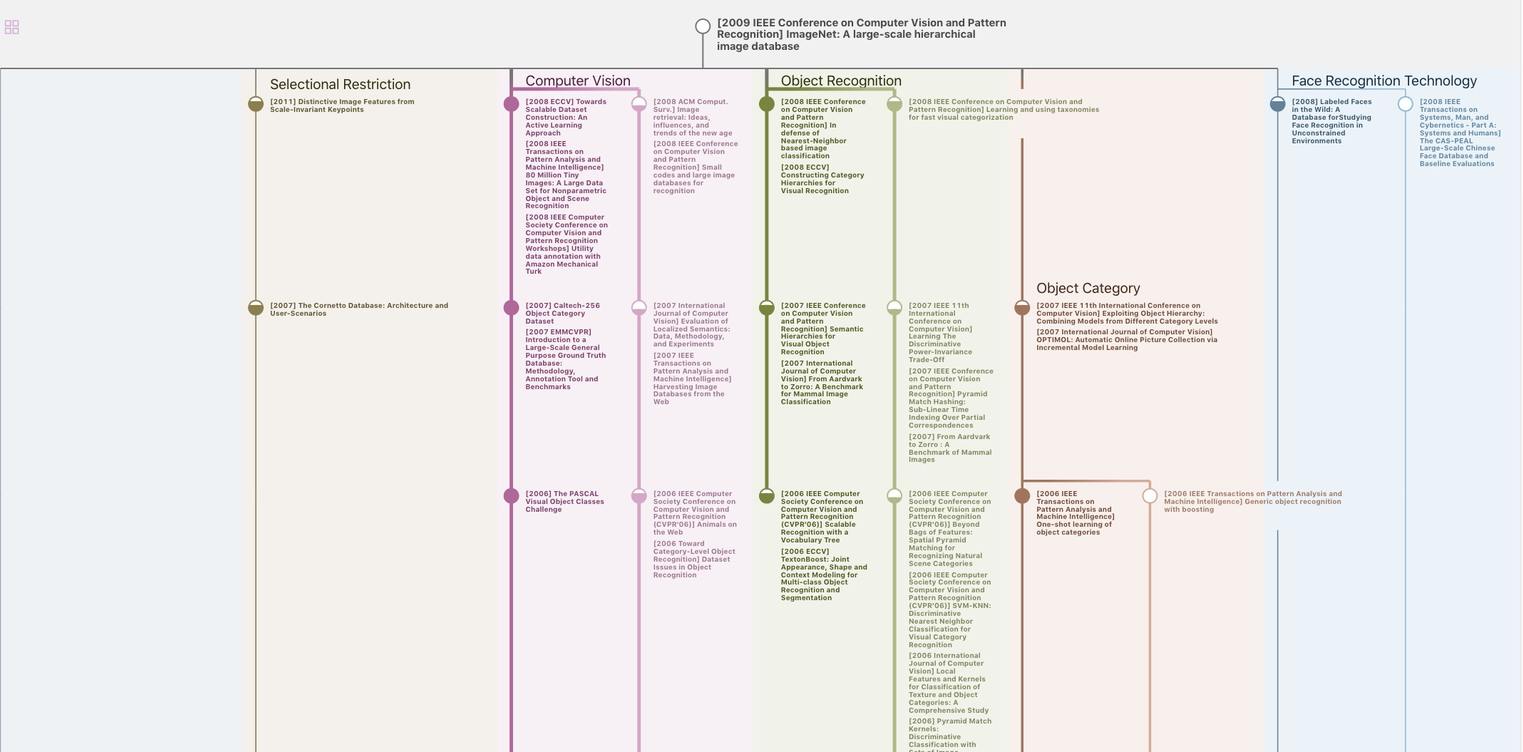
生成溯源树,研究论文发展脉络
Chat Paper
正在生成论文摘要