Multi-Agent Deep Reinforcement Learning-Based Distributed Optimal Generation Control of DC Microgrids.
IEEE Trans. Smart Grid(2023)
摘要
Optimal generation allocation is customarily applied to the DC microgrids to enable optimal operation. Conventionally, the optimization is implemented periodically to obtain the optimal bus voltage or output power references for given operating conditions, which will unavoidably deviate from the actual ones. Since slight disturbances such as load changes will trigger real-time control adjustments, hence, the overall cost will increase due to the disconnect between optimization and real-time control. To overcome this issue, it is preferable to directly apply the optimal control method to render an optimal time path of control actions in real-time. This paper has studied the optimal generation control problem as a constrained non-convex problem with non-linearity. DRL has been successfully applied to solve such problems without mathematically modeling the actual system; the links between states and actions are discovered via ongoing environmental interactions, decreasing the reliance on system parameter information. This paper also showed that TD3-based optimal control could be applied to DC microgrids using a monotonical policy gradient search approach. Furthermore, DRL's distributed training and execution framework is designed to realize real-time distributed control. The data sampling, storage, and experience buffer initialization strategy are customized to improve learning efficiency. The case study demonstrated its effectiveness.
更多查看译文
关键词
Multi-agent deep reinforcement learning,DC microgrids,data-efficient,optimal control,distributed system
AI 理解论文
溯源树
样例
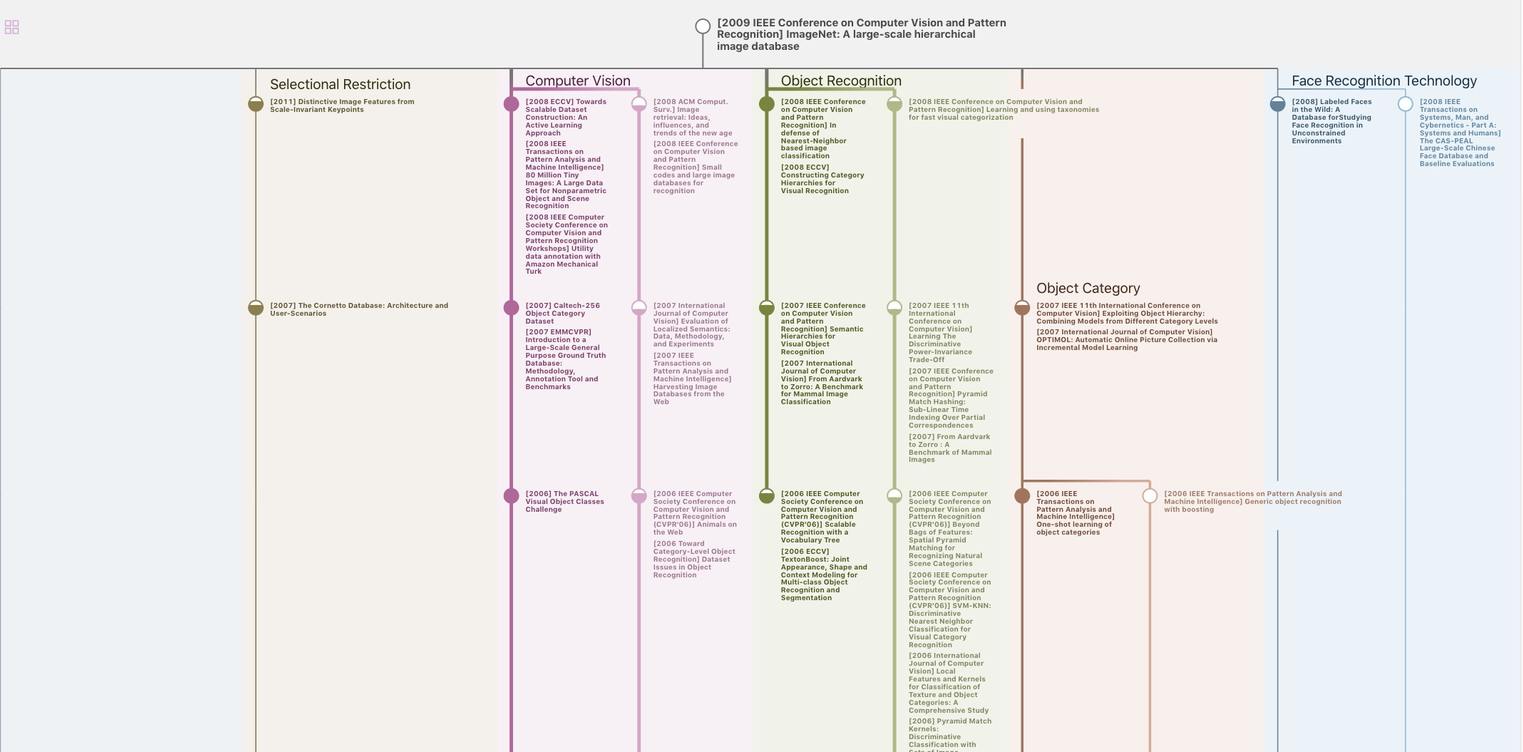
生成溯源树,研究论文发展脉络
Chat Paper
正在生成论文摘要