Adaptive Mixture Model Reduction based on the Composite Transportation Dissimilarity.
FUSION(2023)
摘要
Providing efficient yet accurate statistical models is a challenging problem in many applications. When elementary models are not sufficiently descriptive, mixtures of densities can be used. A complexity management issue arises when mixture models are employed: the number of components should be a trade-off between the complexity and the accuracy of the model. However, in general, it is not obvious how to determine the right number of mixture components for a specific application. In a previous work, theoretical foundations to address such a topic have been laid, grounded on the use of the Composite Transportation Dissimilarity between mixtures, and a preliminary criterion to manage the complexity of a mixture model has been proposed. In this paper, additional theoretical insights are provided that allow to formulate a novel adaptive mixture reduction algorithm. Numerical tests show that in most cases the new algorithm constitutes a significant improvement over the previous one.
更多查看译文
关键词
Model Selection,Kullback-Leibler Divergence,Mixture Reduction,Optimal Transport Theory
AI 理解论文
溯源树
样例
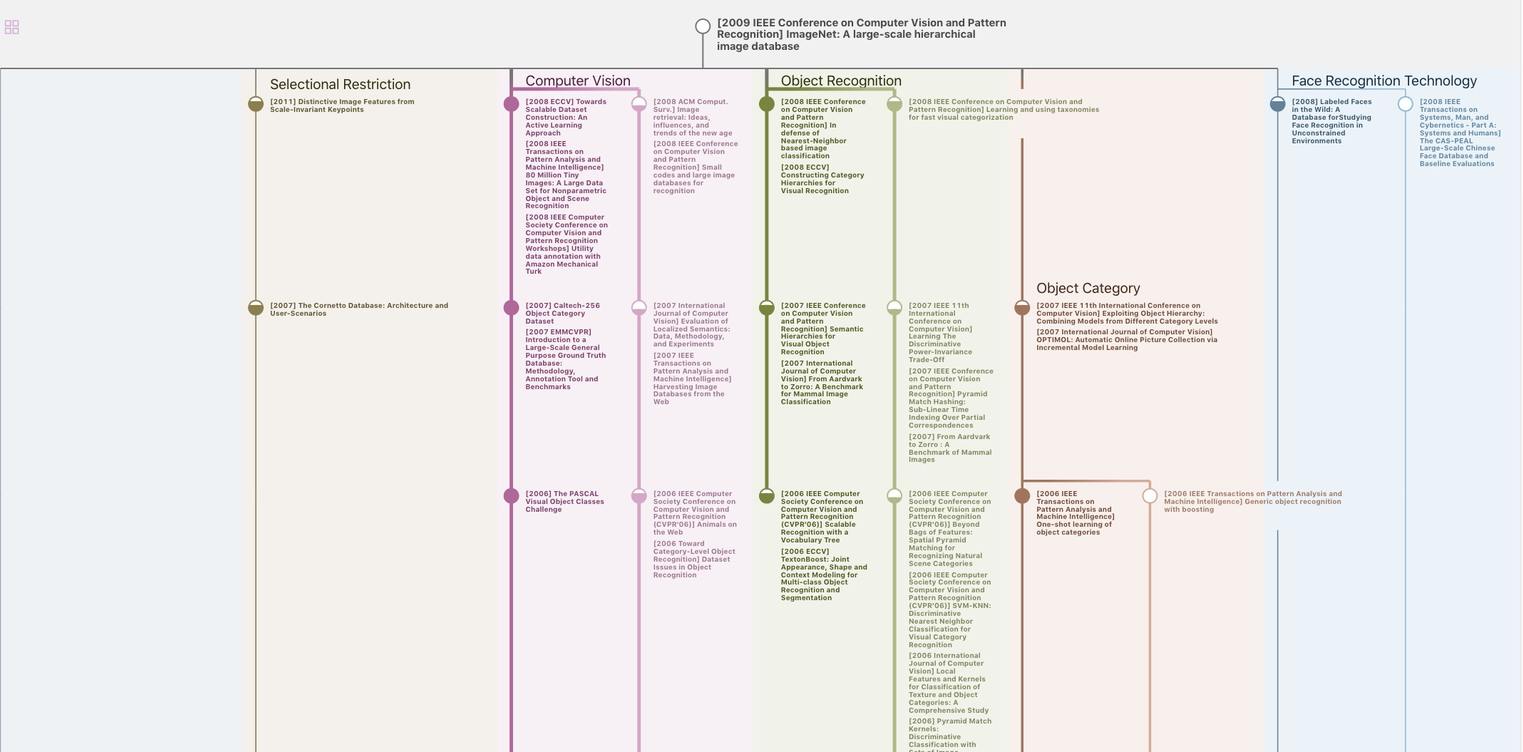
生成溯源树,研究论文发展脉络
Chat Paper
正在生成论文摘要