Belief propagation for marginal probabilities in multiple hypothesis tracking.
FUSION(2023)
摘要
This paper explores evaluation of association marginals in multiple hypothesis tracking. The work builds upon recent results where loop belief propagation (LBP) has been used in single-hypothesis cases. There are two contributions in the paper. The first is a novel factor graph representation of the joint multi-hypothesis association posterior. The second contribution is two algorithms that both use LBP to evaluate association marginals. The first method uses total probability in conjunction with hypothesis-conditioned LBP, and is called PHD-LBP. The second method is an LBP algorithm running directly on the full multi-hypothesis association graph with novel, specialized message definitions that are derived in this paper and efficient to compute and store in memory, and is called MH-LBP. Results show that both algorithms perform well with high correlation with the exact marginals for the majority of the cases.
更多查看译文
AI 理解论文
溯源树
样例
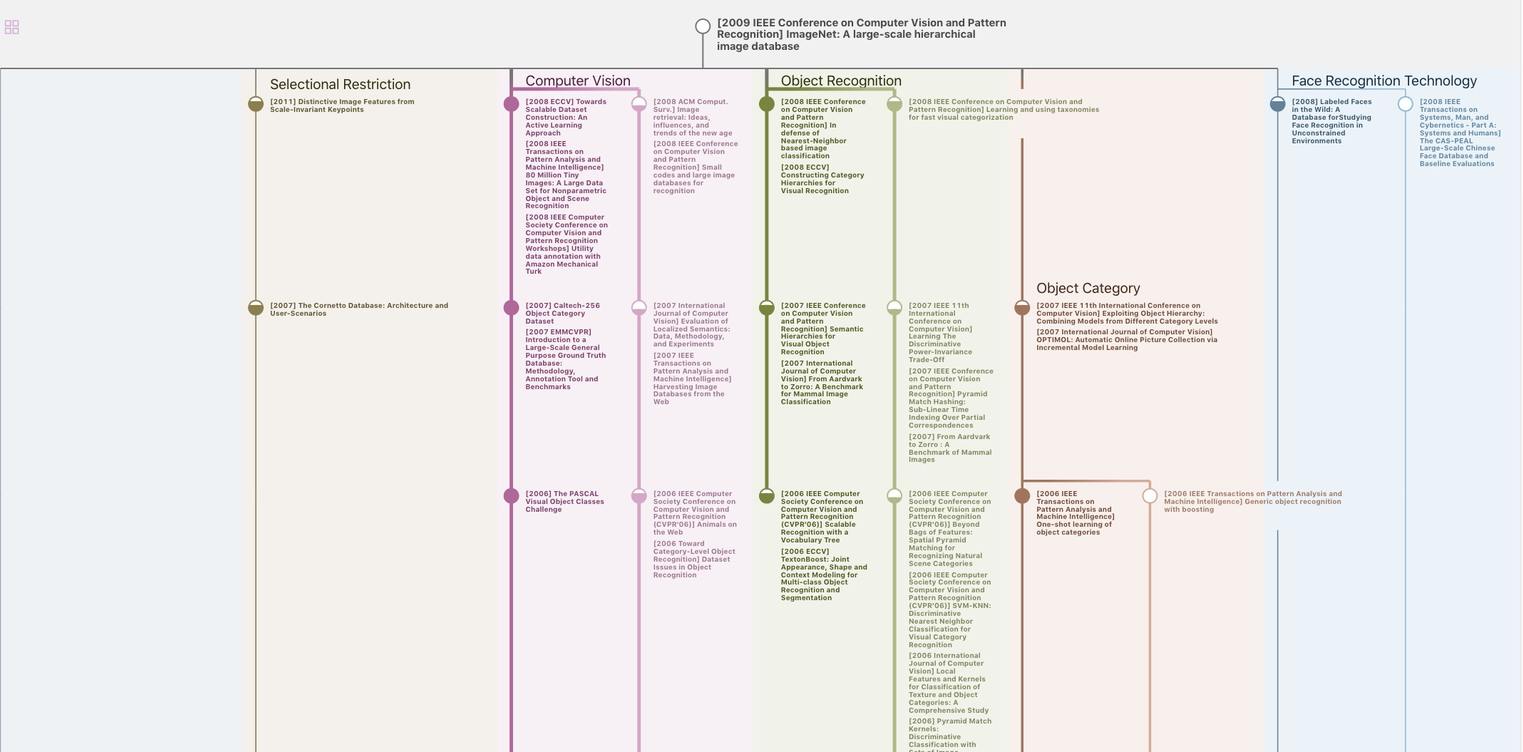
生成溯源树,研究论文发展脉络
Chat Paper
正在生成论文摘要