Ensemble Gaussian Mixture Filtering with Particle-localized Covariances.
FUSION(2023)
摘要
The ensemble Gaussian mixture filter (EnGMF) is a powerful filter for highly non-Gaussian and non-linear models that has practical utility in the case of a small number of samples, and theoretical convergence to full Bayesian inference in the ensemble limit. We aim to increase the utility of the EnGMF by introducing a particle-local notion of covariance into the Gaussian mixture estimate of the prior distribution. We show on a simple bivariate problem that each particle having its own local estimate of the covariance both has nice qualitative and quantitative properties, and significantly improves the estimate of the prior and posterior distributions for all ensemble sizes. We additionally show the utility of the proposed methodology for sequential filtering for the Lorenz ’63 equations, achieving a significant reduction in error in the low ensemble size regime.
更多查看译文
关键词
ensemble Gaussian mixture filter,localization,sequential filtering,data assimilation
AI 理解论文
溯源树
样例
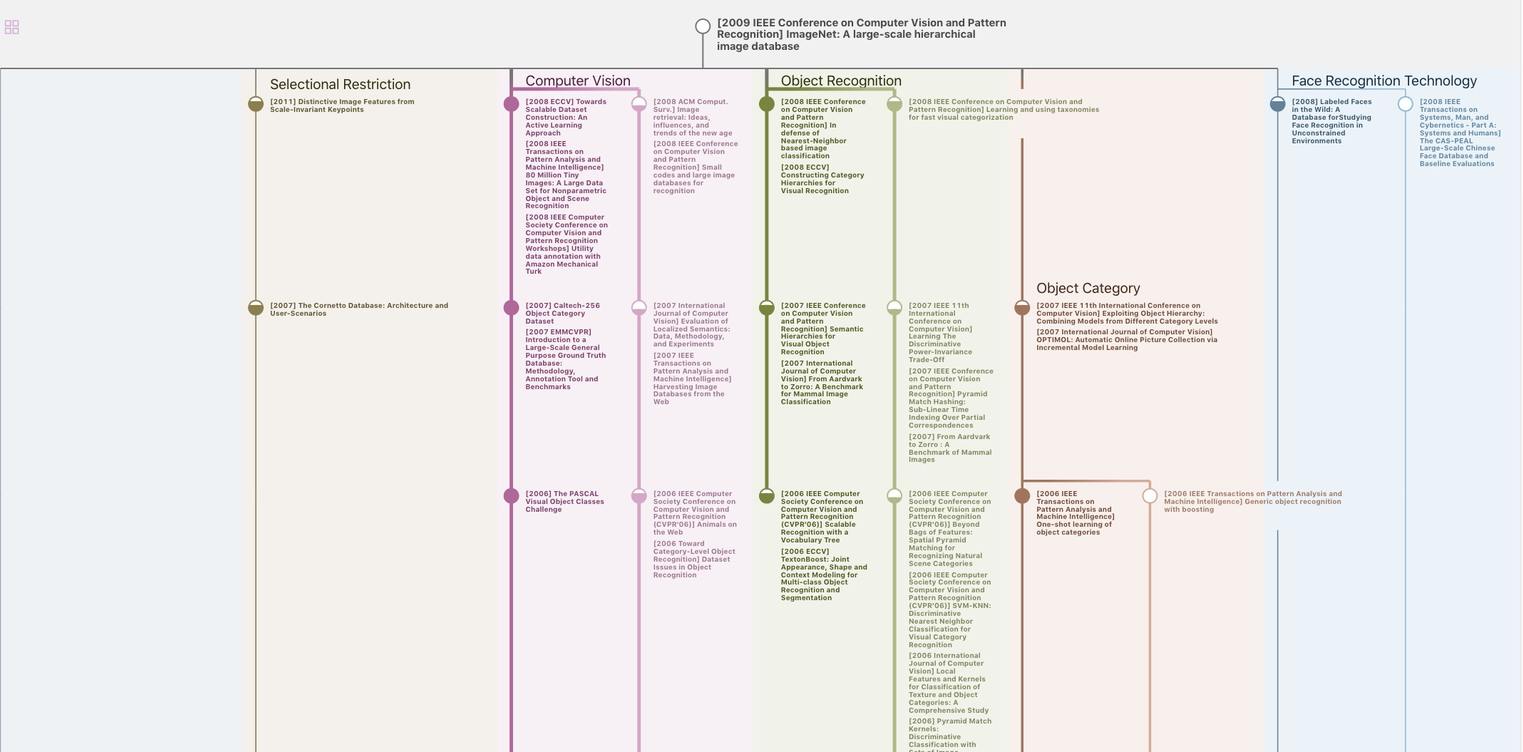
生成溯源树,研究论文发展脉络
Chat Paper
正在生成论文摘要