Model-based Deep Learning for Maneuvering Target Tracking.
FUSION(2023)
摘要
Maneuvering target tracking, where the system undergoes abrupt changes in the underlying motion model, can be challenging. We propose a model-based deep learning approach for prediction of maneuvering targets to exploit partial knowledge of the system physics-based models during training, without requiring an explicit characterization or fine tuning of model parameters. We formulate a supervised training scheme to learn the dynamics of state-space models and capture the jump processes governing model transitions by minimizing the prediction loss of an encoder-decoder network from model-based generated data. The effectiveness of the proposed method is demonstrated in two maneuvering target tracking scenarios using synthetic and real-world test data. The results show that the model-based encoder-decoder network achieves notably improved performance in terms of target prediction compared to conventional multiple-model solutions, especially when facing model inaccuracies, jumps, and dominant nonlinearities during target maneuvers.
更多查看译文
关键词
Model-based deep learning,state-space models,encoder-decoder,maneuvering target tracking
AI 理解论文
溯源树
样例
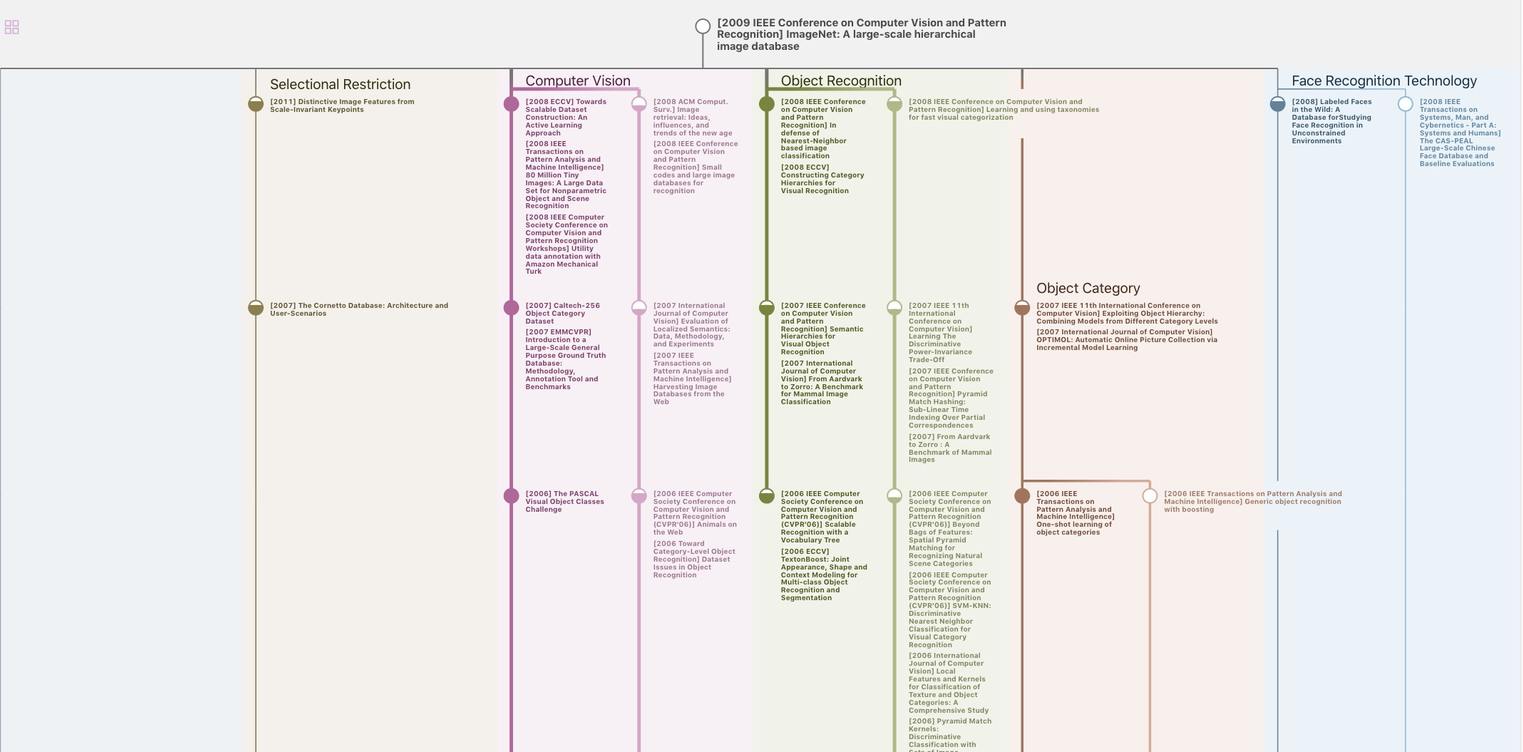
生成溯源树,研究论文发展脉络
Chat Paper
正在生成论文摘要