Multistream Convolutional Neural Network Fusion for Pixel-wise Classification of Peatland.
FUSION(2023)
摘要
Recently, Convolutional Neural Network (CNN) has shown higher performance than other machine learning methods for land classification. In this paper, we propose a CNN fusion architecture for peatland site type classification by combining multisource and multiresolution data. The data is acquired by optical and radar satellite remote sensing, airborne laser scanning data and multi-source forest inventory GIS datasets. Based on our data, we are dealing with the high-dimensional class-imbalanced dataset for solving pixel-wise classification of peatlands. To reduce the data dimension and find an optimal subset of inputs, we first applied the sequential feature selection method. Then, we proposed a window-based pixel classification approach based on the selected inputs. This approach can extract the spatial information around each training sample in a defined window region and produce a pixel-wise classification map. Experiments are carried out for ecological classification of peatlands in Finland.
更多查看译文
关键词
convolutional neural network,land cover classification,semantic segmentation,remote sensing
AI 理解论文
溯源树
样例
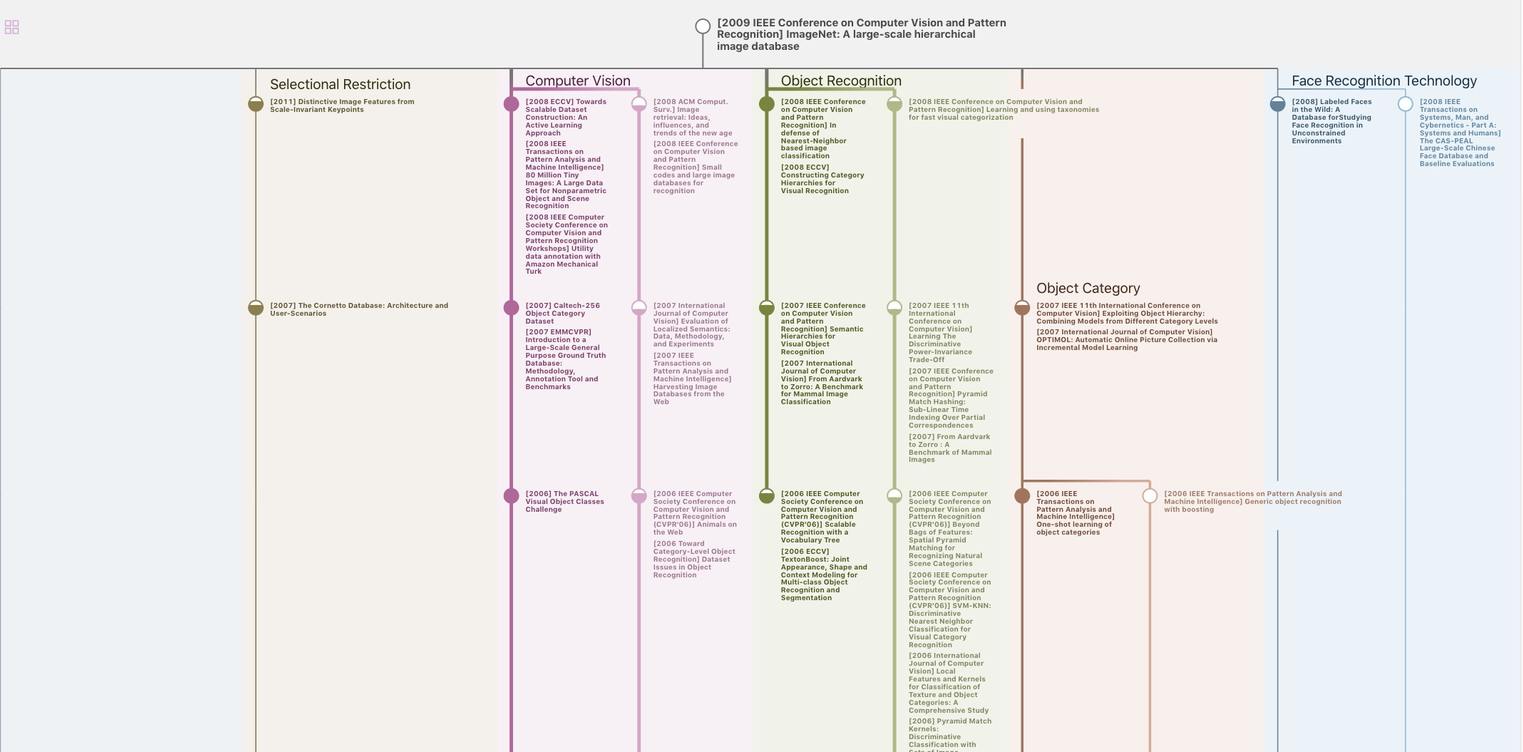
生成溯源树,研究论文发展脉络
Chat Paper
正在生成论文摘要