Nonexpert Crowds Outperform Expert Individuals in Diagnostic Accuracy on a Skin Lesion Diagnosis Task.
ISBI(2023)
摘要
A recent study [1] showed that individual physicians with at least ten years of experience as dermatologists achieved 74.7% accuracy on average in labeling images from the multiclass International Skin Imaging Collaboration (ISIC) 2018 challenge dataset. Using a novel gamified crowdsourcing method, we collected 144,383 nonexpert opinions over two weeks on the medical image annotation platform DiagnosUs, and the resulting crowd consensus labels obtained by aggregating using a plurality rule achieved a significantly higher accuracy of 78.1% (p=0.0014), a multiclass ROC AUC (area under the receiver operating characteristic curve) of 0.948 (95% CI 0.936-0.959), and malignant versus benign ROC AUC of 0.928 (95% CI 0.911-0.943). These results suggest an opportunity to harness gamified methods to assist in the creation of high-quality labeled datasets that could benefit medical artificial intelligence (AI) development.
更多查看译文
关键词
Skin lesion classification,ISIC,crowdsourcing,gamification
AI 理解论文
溯源树
样例
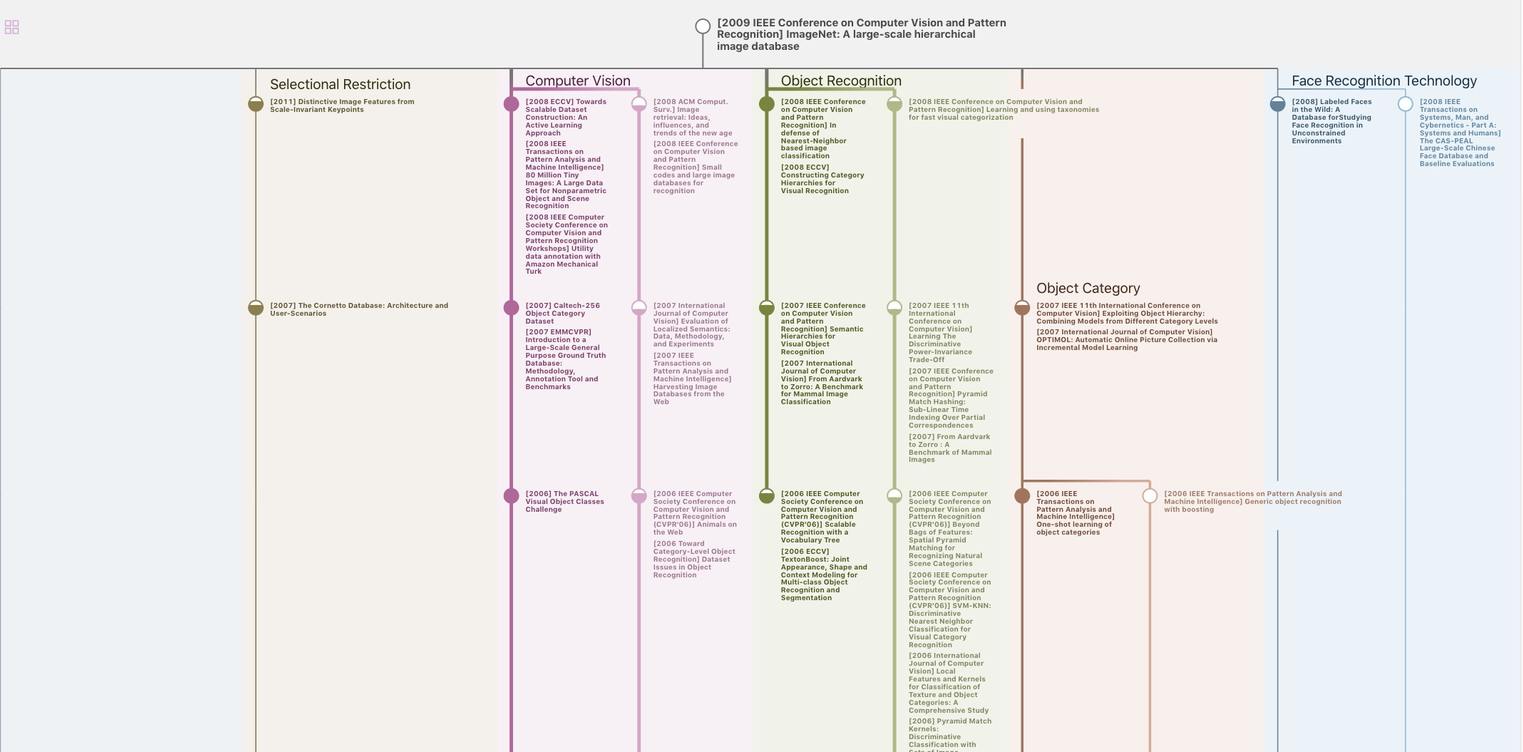
生成溯源树,研究论文发展脉络
Chat Paper
正在生成论文摘要