Learning-Based Material Decomposition in Dual Energy CT Using an Unrolled Estimator.
ISBI(2023)
摘要
Reconstructing multiple material-specific images in dual-energy CT is a challenging non-linear inverse problem. Traditionally, the reconstruction process consists of two steps: material decomposition and tomographic reconstruction. Model-based iterative reconstruction methods that combine material decomposition and tomographic reconstruction into a unified "one-step" framework provide improved estimates, but require longer reconstruction times. To address this, we propose a supervised machine learning technique to accelerate one-step iterative dual-energy CT reconstruction. Specifically, we train a deep neural network embedded in an "unrolling" of a model-based iterative algorithm. We demonstrate this approach on the problem of identifying three materials (adipose tissue, fibroglandular tissue, and calcifications) from simulated breast dual-energy CT data. Empirically, we find that the unrolling approach gives accurate material map estimates in few iterations, and outperforms a baseline image-domain learning approach.
更多查看译文
关键词
dual-energy CT,material decomposition,model-based iterative reconstruction,machine learning
AI 理解论文
溯源树
样例
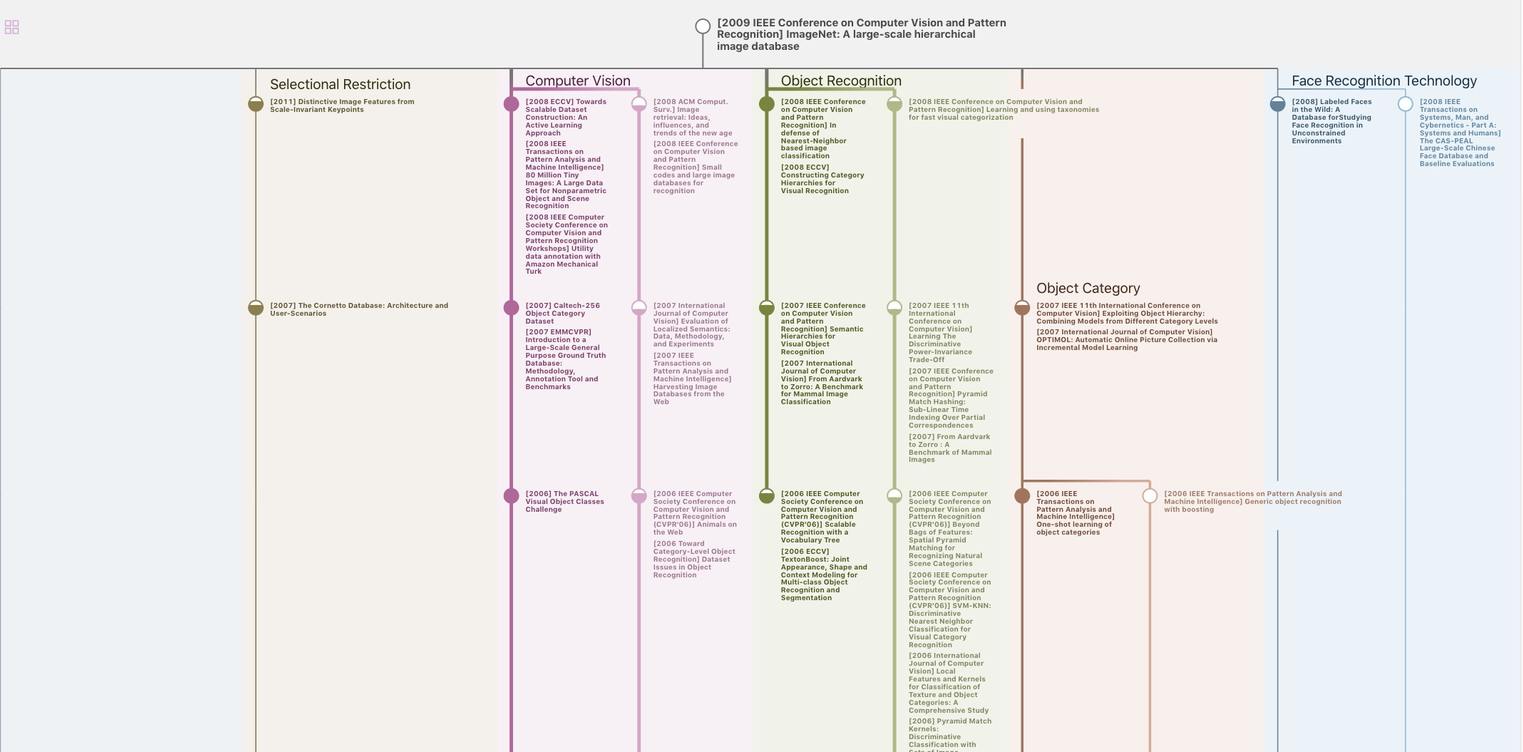
生成溯源树,研究论文发展脉络
Chat Paper
正在生成论文摘要