Expectation-Maximization Regularised Deep Learning for Tumour Segmentation.
ISBI(2023)
摘要
We present an expectation-maximization (EM) regularized deep learning (EMReDL) approach for weakly supervised tumor segmentation using partially labelled MRI. The proposed framework is demonstrated on glioblastoma, characterized by diffusion infiltration. Physiological MRI provides specific information regarding infiltration over structural MRI but is hindered by its low resolution for precise labeling. To exploit partial labels, we design two components in EMReDL: 1) a physiological prior prediction model: a neural network-based binary classifier trained by the labels of core tumor and normal-appearing regions. The trained classifier generates a physiological prior map passed to 2) a segmentation model regularized under an EM framework for weakly supervised learning. We evaluate the performance on a dataset with preoperative multiparametric and recurrence MRI. Results show that EMReDL can effectively segment the infiltrated tumor from the partially labeled MRI, with an accuracy higher than the model trained without physiological MRI and other competing approaches. We will publish the code with example data soon.
更多查看译文
关键词
Expectation Maximization, Weakly Supervised Learning, Tumor Segmentation
AI 理解论文
溯源树
样例
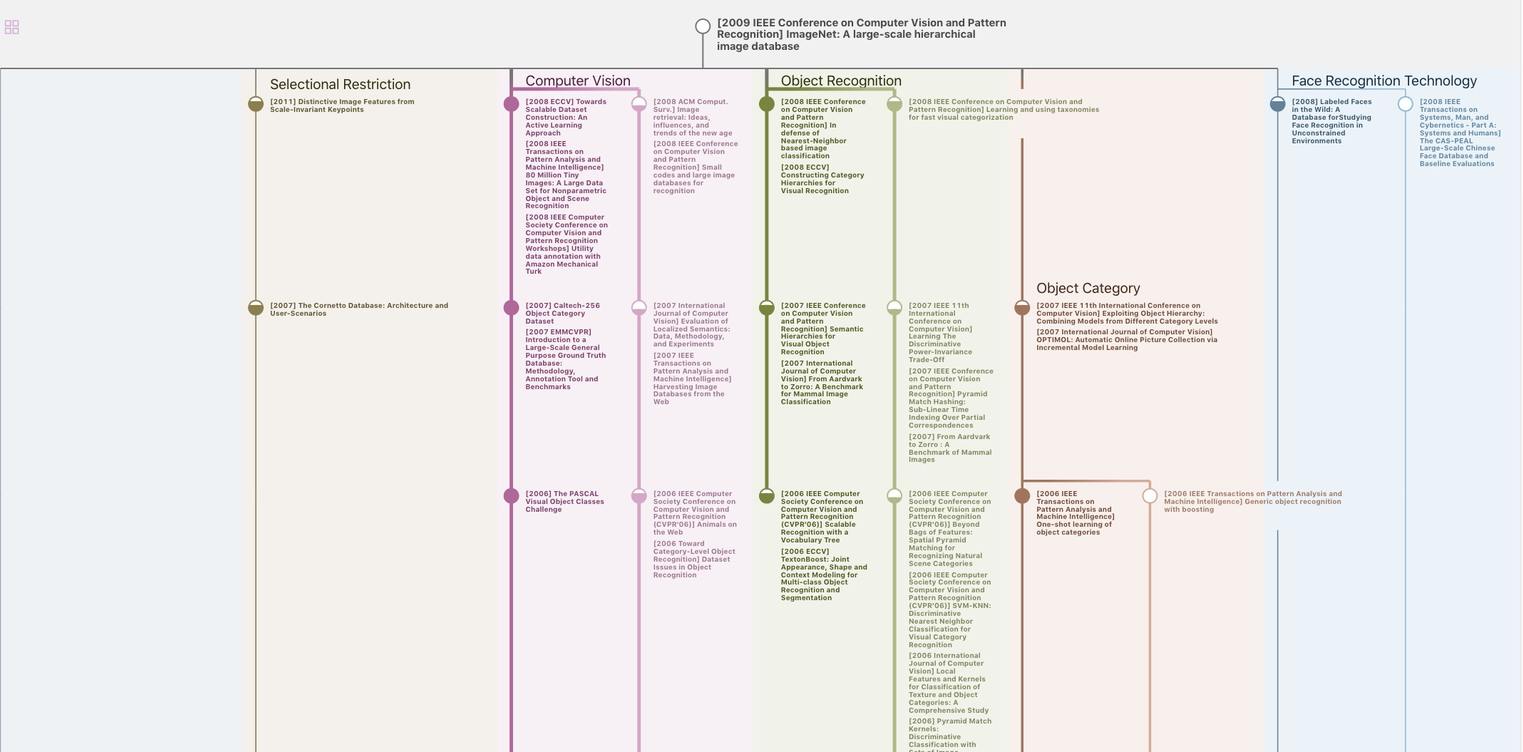
生成溯源树,研究论文发展脉络
Chat Paper
正在生成论文摘要