Reproducibility of Tumor Segmentation Outcomes with a Deep Learning Model
ISBI(2023)
摘要
In the last few years, there has been a growing awareness of reproducibility concerns in many areas of science. In this work, our goal is to evaluate the reproducibility of tumor segmentation outcomes produced with a deep segmentation model when MRI images are pre-processed (i) with two different versions of the same pre-processing pipeline, and (ii) by introducing numerical perturbations that mimic executions on different environments. Results show that these two variability sources can lead to important variations of segmentation outcomes: Dice can go as low as 0.59 and Hausdorff distance as high as 84.75. Moreover, both cases show a similar range of values, suggesting that the underlying causes for instability may be numerical stability. This work can be used as a benchmark to improve the numerical stability of the pipeline.
更多查看译文
关键词
reproducibility,deep learning,numerical (in)stability,tumor segmentation
AI 理解论文
溯源树
样例
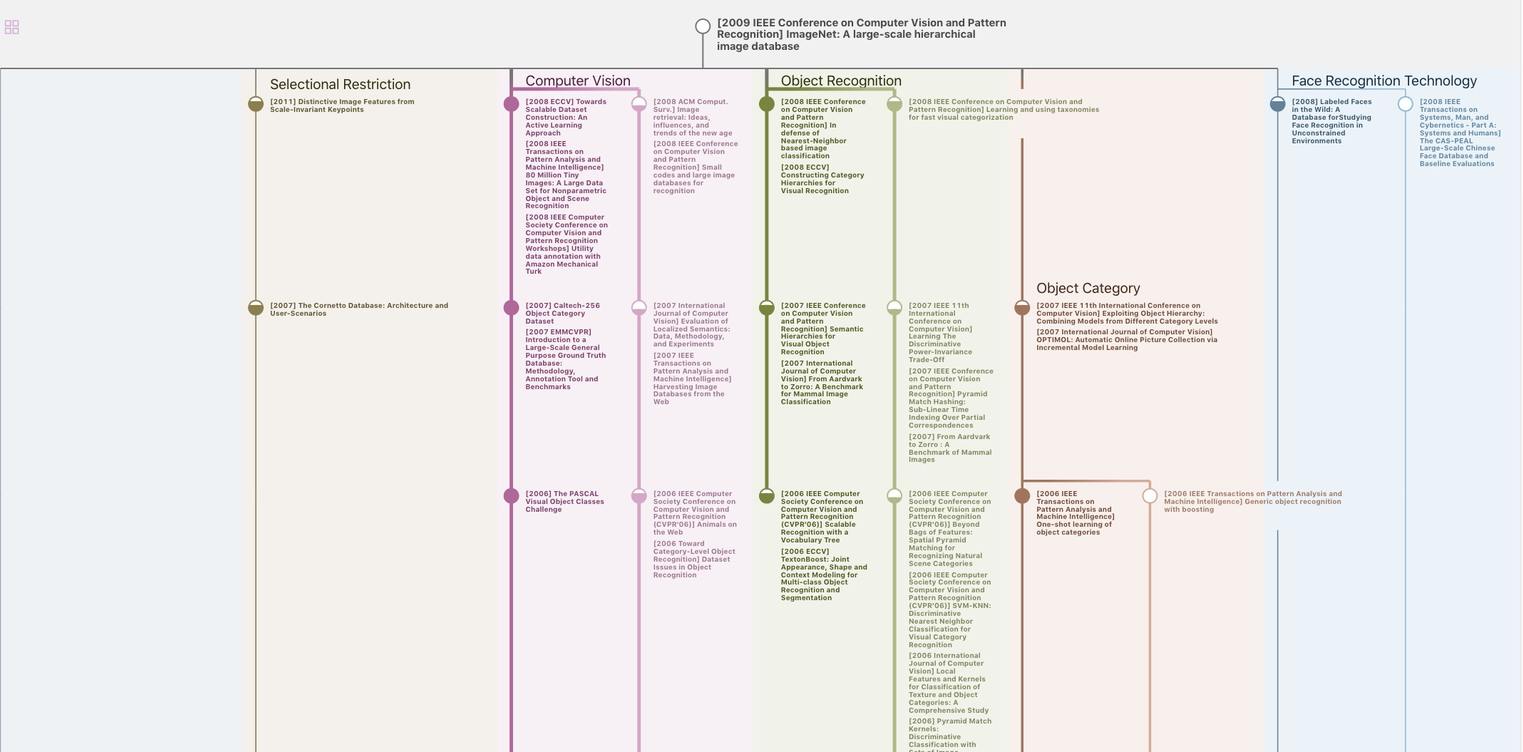
生成溯源树,研究论文发展脉络
Chat Paper
正在生成论文摘要