SegCrop: Segmentation-based Dynamic Cropping of Endoscopic Videos to Address Label Leakage in Surgical Tool Detection.
ISBI(2023)
摘要
In recent times, surgical data science has emerged as an important research discipline in interventional healthcare. There are many potential applications for analysing endoscopic surgical videos using machine learning (ML) techniques such as surgical tool classification, action recognition, and tissue segmentation. However, the efficacy of ML algorithms to learn robust features drastically deteriorates when models are trained on noise-affected data [1]. Appropriate data preprocessing for endoscopic videos is thus crucial to ensure robust ML training. To this end, we demonstrate the presence of label leakage when surgical tool classification is performed naively and present SegCrop, a dynamic U-Net model with an integrated attention mechanism to dynamically crop the arbitrary field of view (FoV) in endoscopic surgical videos to remove spurious label-related information from the data. In addition, we leverage explainability techniques to demonstrate how the presence of spurious correlations influences the model's learning capability.
更多查看译文
关键词
Surgical Data Science,Surgical Tool Detection,Image Segmentation,Robust ML,Explainable AI
AI 理解论文
溯源树
样例
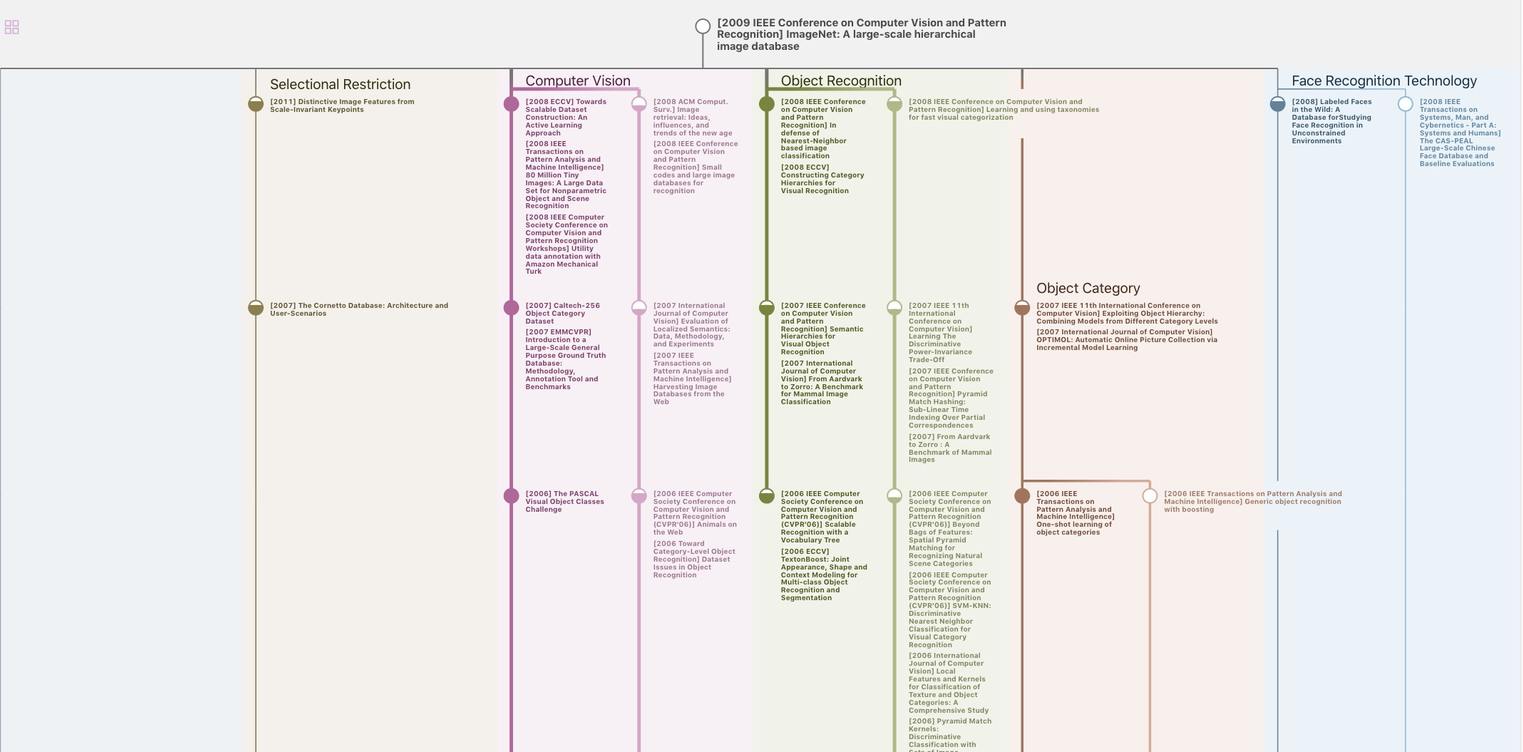
生成溯源树,研究论文发展脉络
Chat Paper
正在生成论文摘要