Spatial Lesion Graphs: Analyzing Liver Metastases with Geometric Deep Learning for Cancer Survival Regression.
ISBI(2023)
摘要
The degree of metastatic progress is a crucial factor in cancer assessment and is often estimated from few target lesions. However, features of the overall configuration of metastases, such as spread distance in the liver, offer further predictive information. Graphs can represent both local characteristics of individual lesions and their global spatial distribution. In this work, liver metastases stemming from a primary pancreatic tumor are transformed patient-wise into spatial lesion graphs and processed by geometric deep learning to estimate hazard ratios. The influence of lesion features and graph topologies is investigated in a data set of 78 patients to provide model design guidelines. Graph attention and differential pooling networks were selected as architectures to capitalize on the proposed topologies and provide meaningful visualizations of the decision process. Compared with conventional survival analysis methods and deep learning models, both networks show improvement with a maximum concordance of 68.9 %.
更多查看译文
关键词
Geometric deep learning, survival analysis, multi-focal diseases, graphs, cancer, metastasis, liver
AI 理解论文
溯源树
样例
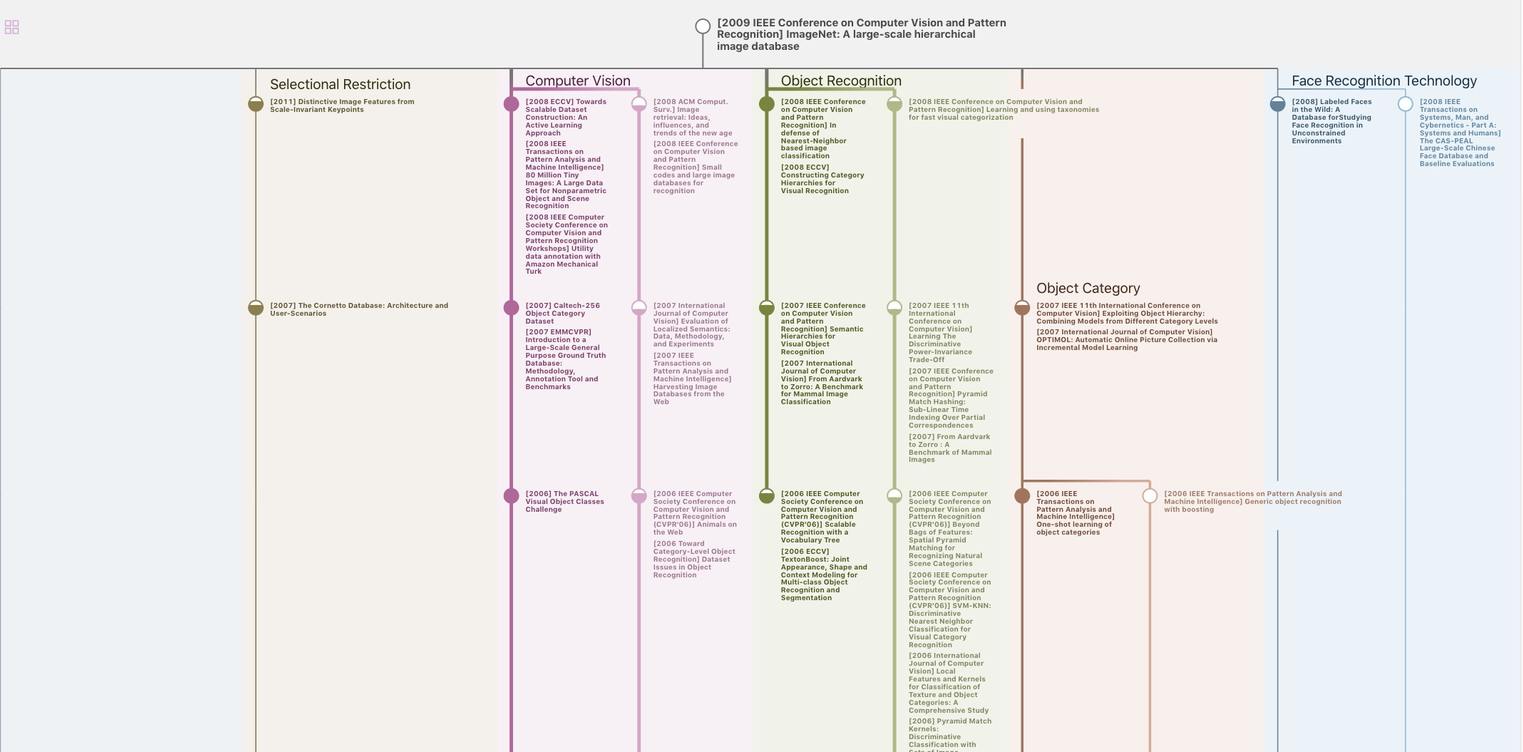
生成溯源树,研究论文发展脉络
Chat Paper
正在生成论文摘要