Voxel-Level fMRI Analysis by Representation Learning and Deep Clustering for Alzheimer's Disease.
ISBI(2023)
摘要
With the progression of neurodegenerative disease, functional connectivity between brain regions has changed, which can be reflected locally by Blood-oxygen-level-dependent (BOLD) signal measured in functional magnetic resonance imaging (fMRI). Most studies assume BOLD signals are homogeneous within brain regions, ignoring voxel-level changes. In this paper, we propose a novel framework for voxel-based feature extraction and recollection to characterize the BOLD signal and analyze the functional connectivity of brain networks and uncover biomarkers for abnormalities. Specifically, a weakly-supervised learning strategy is adopted to extract discriminative representation from original BOLD signals. Considering the heterogeneity of BOLD signals within brain regions of interest (ROIs), we employ an unsupervised-based deep clustering method to automatically recollect features to different clusters. Experiments on Alzheimer's Disease (AD) recognition using Graph neural network (GNN) validate the effectiveness of our framework. To the best of our knowledge, this is the first work to consider BOLD signal heterogeneity for feature extraction to measure functional connectivity in GNN, which provides a voxel-level scenario that can be migrated to other tasks.
更多查看译文
关键词
Weakly-supervised Representation Learning, Deep Clustering, GNN, fMRI, Alzheimer's Disease
AI 理解论文
溯源树
样例
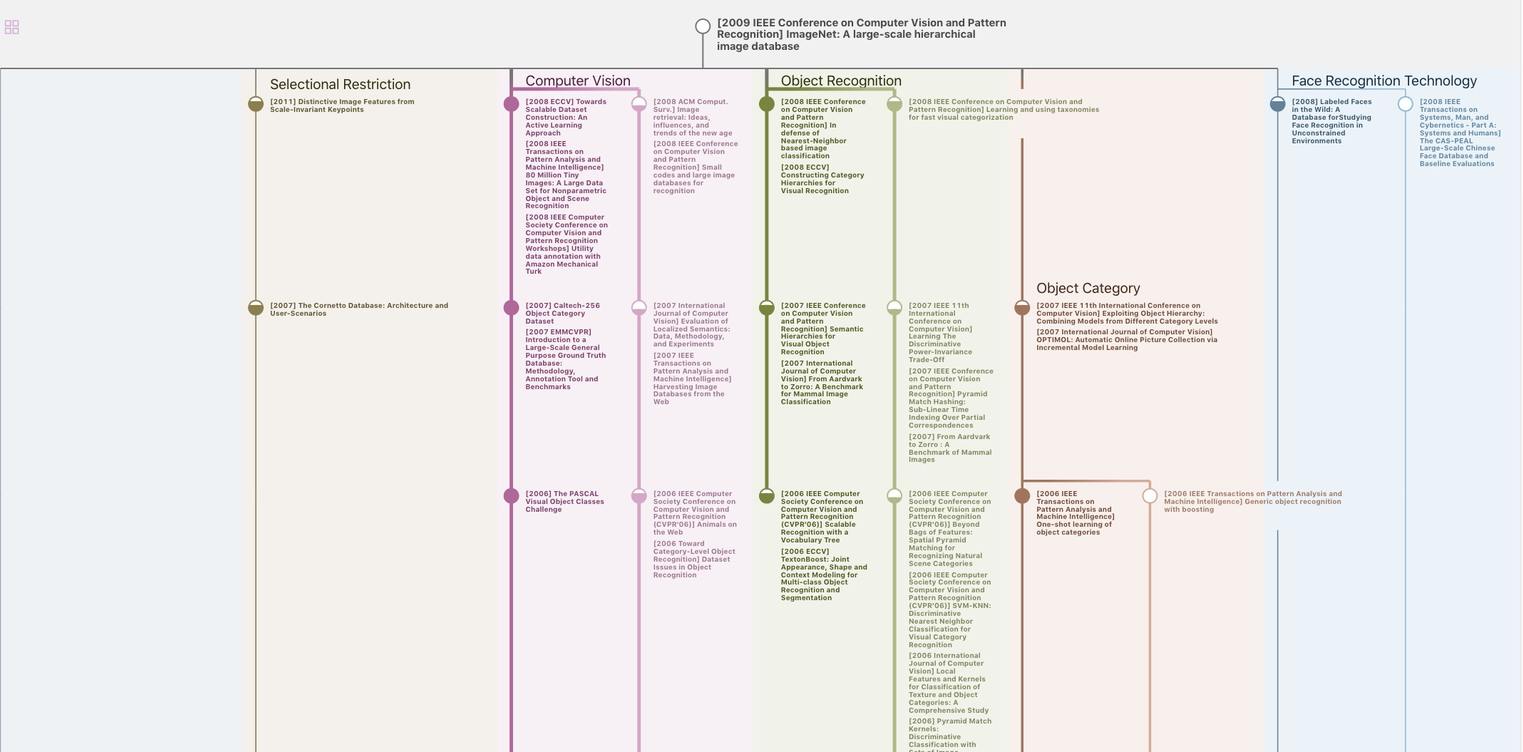
生成溯源树,研究论文发展脉络
Chat Paper
正在生成论文摘要