G2LL: Global-To-Local Self-Supervised Learning for Label-Efficient Transformer-Based Skin Lesion Segmentation in Dermoscopy Images.
ISBI(2023)
摘要
Skin lesion segmentation in dermoscopy images is highly relevant for lesion assessment and subsequent analysis. Recently, automatic transformer-based skin lesion segmentation models have achieved high segmentation accuracy owing to their long-range modeling capability. However, limited labeled data for training the lesion segmentation models results in sub-optimal learning results. In this paper, we propose a Global-to-Local self-supervised Learning (G2LL) method for transformer-based skin lesion segmentation models to alleviate the problem of insufficient annotated data. Firstly, a structure-wise masking strategy for Masked Image Modeling (MIM) is proposed to force the model to learn the reconstruction of masked structures by exploring the semantic local contexts. Instead of masking patches randomly in the whole view, it computes superpixels to divide the images into several structured regions. Then, it masks the fixed number of patches in each region, thus it allows the exploration of the structural knowledge and solves the shape variance in the meanwhile. Secondly, a self-distilling architecture is deployed to enhance global context learning where the masked images are sent to a student network and the relative unmasked images are fed to a teacher network for knowledge distillation. In this context, extensive experiments on both the ISIC-2017 and the ISIC-2019 datasets containing a total of 28 081 images show that the proposed approach is superior to state-of-the-art self-supervised learning methods.
更多查看译文
关键词
skin lesion segmentation, self-supervised learning, structure-wise masking
AI 理解论文
溯源树
样例
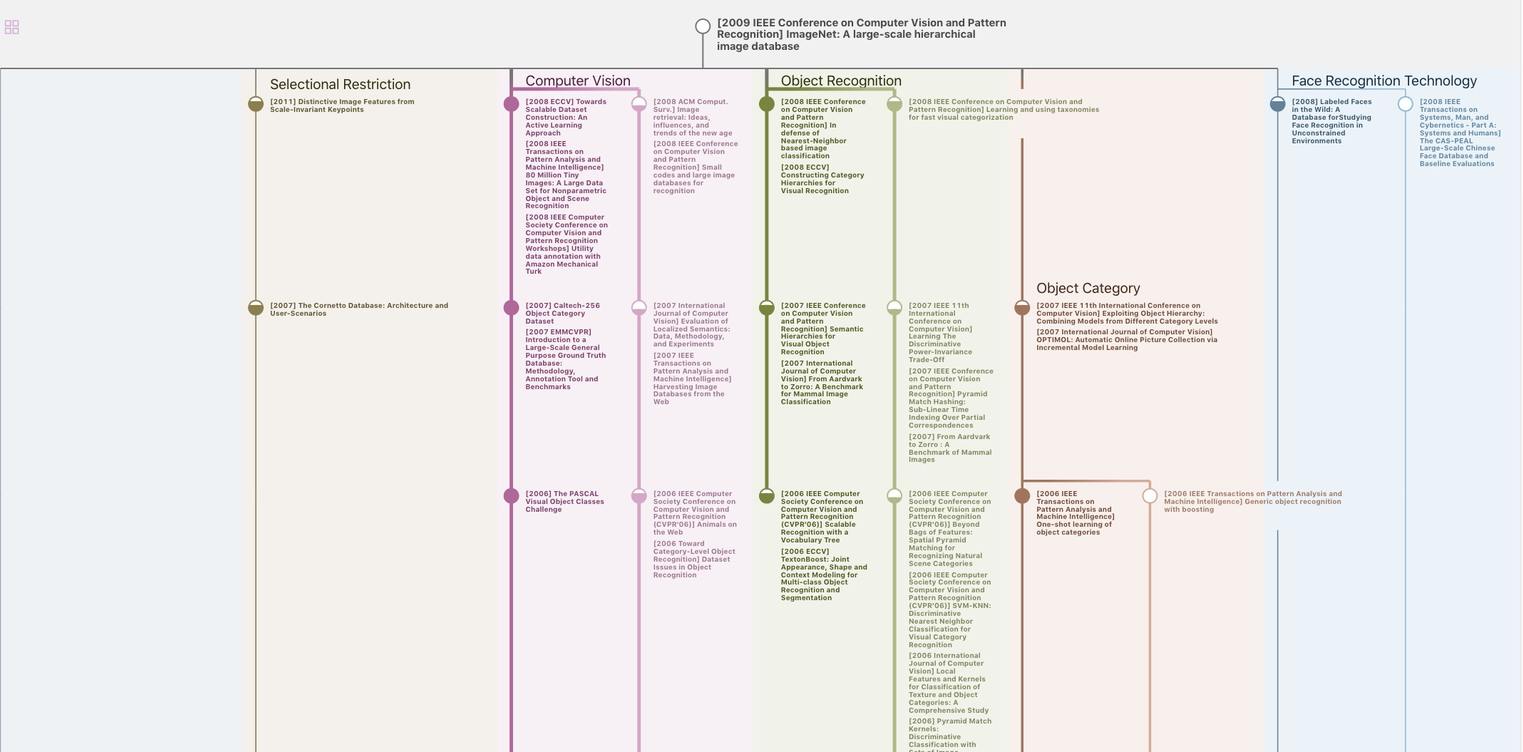
生成溯源树,研究论文发展脉络
Chat Paper
正在生成论文摘要