PCT-GAN: A Real CT Image Super-Resolution Model for Trabecular Bone Restoration.
ISBI(2023)
摘要
We consider the real-world super-resolution (SR) problem for medical images with two distinct imaging modalities. Most previous attempts either focused on image enhancement between similar imaging modalities or used the down-sampled or simulated low-resolution images as input to train their model, which reduces the clinical significance of the work. We, therefore, collect a dataset with paired clinical computed tomography (CT) and microcomputed tomography (mu CT) of vertebra specimens and develop the progressive CT generative adversarial network (PCT-GAN) model to generate high-resolution images. PCT-GAN is designed using the progressive strategy by first denoising the input clinical CT image and then increasing its spatial resolution. To encourage our model to generate trabecular bone details from clinical CTs, we propose to combine wavelet and edge losses with the L1 and adversarial losses for model training. Experimental results show that the proposed method outperforms the state-of-the-art methods in terms of quantitative measurements and visual results.
更多查看译文
关键词
Real-world medical image super-resolution, generative adversarial network, computed tomography image enhancement
AI 理解论文
溯源树
样例
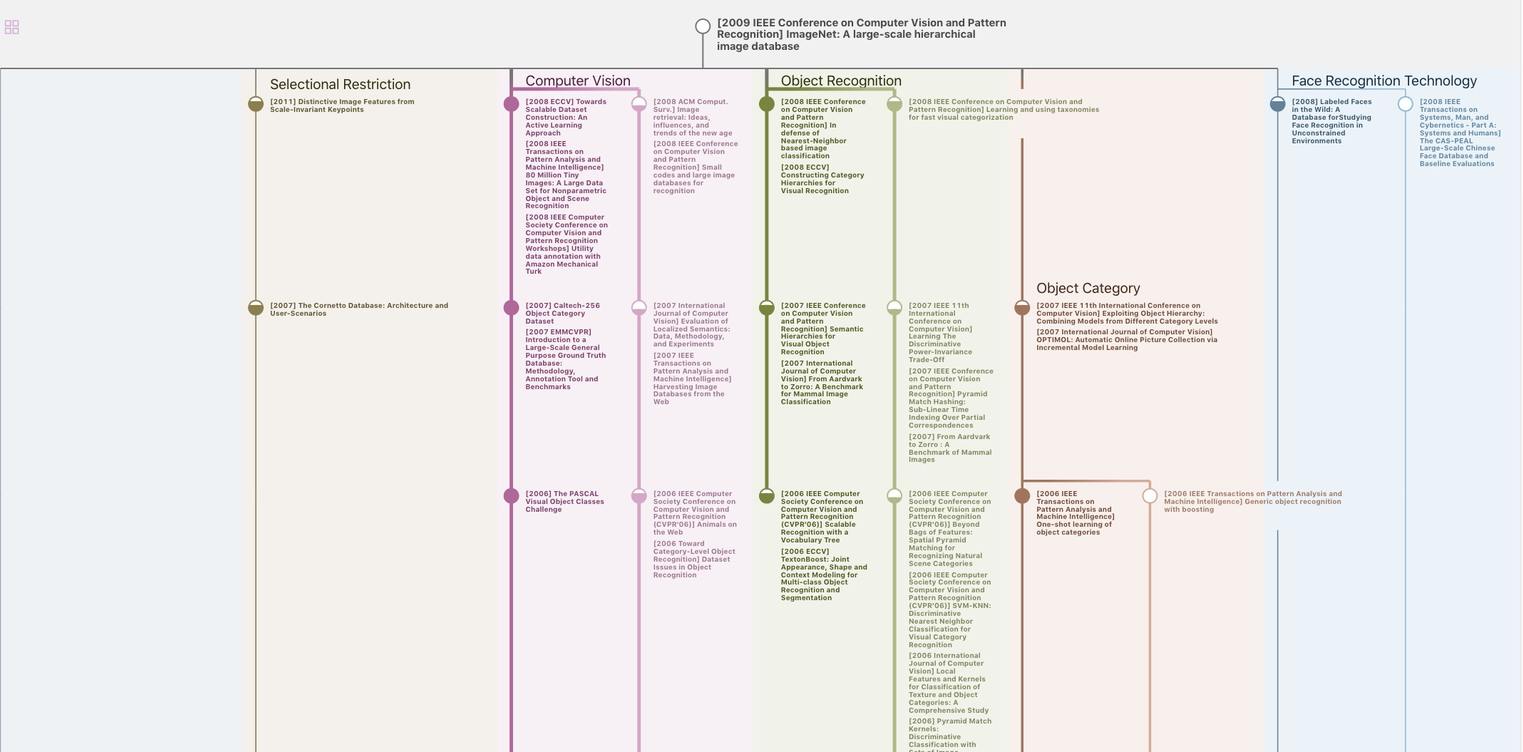
生成溯源树,研究论文发展脉络
Chat Paper
正在生成论文摘要