Exploring Uncertainty for Clinical Acceptability in Head and Neck Deep Learning-Based OAR Segmentation.
ISBI(2023)
摘要
One of the most important steps in head and neck (HN) cancer radiotherapy treatment planning is to accurately delineate the organs at risk (OARs). Deep learning (DL) has proven to be an efficient tool for this task, but its implementation into the clinic is hindered by a lack of trust among users, among other factors. We propose to evaluate a DL-based segmentation tool with the following metrics: (a) a clinical assessment to analyze the clinical acceptability of the predicted OAR segmentations; (b) a classification method to identify possible erroneous segmentations based on the uncertainty of their predictions. Results showed a high acceptance of DL contours, with all cases being approved with no or minor editing. The classification model correctly detected 99% of correct contours and 57% and 85% of possible and reliable segmentation outliers. These metrics successfully validated our DL-based model to segment fifteen HN OARs.
更多查看译文
关键词
Deep learning, organ at risk, automated segmentation, head and neck radiotherapy, uncertainty
AI 理解论文
溯源树
样例
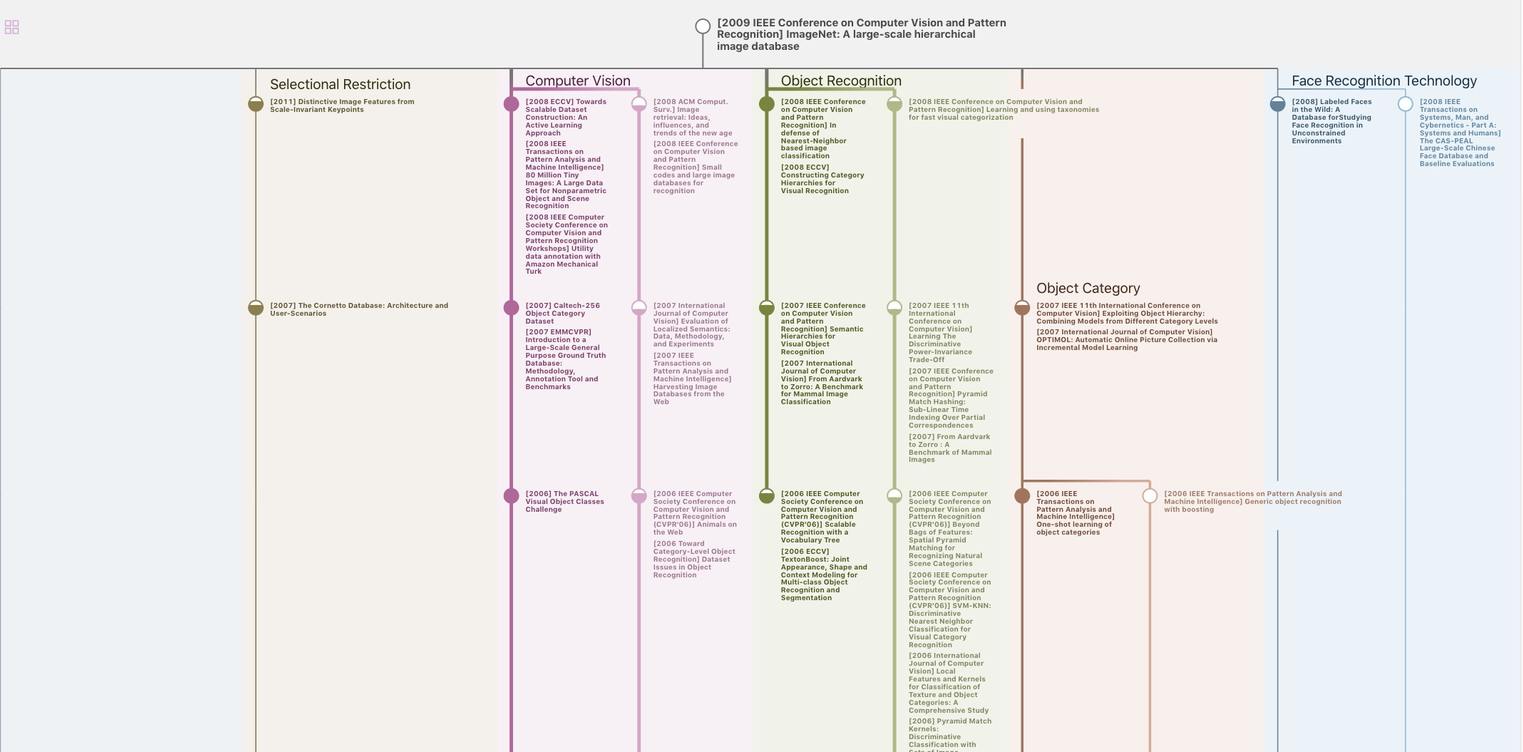
生成溯源树,研究论文发展脉络
Chat Paper
正在生成论文摘要