Strain-Level Identification and Analysis of Avian Coronavirus Using Raman Spectroscopy and Interpretable Machine Learning
ISBI(2023)
摘要
Strain-level identification of viruses is important for decision making in public health management. Recently, Raman spectroscopy has attained great attention in virus identification since it enables rapid and label-free analysis. In this paper, we present an interpretable machine learning approach for strain-level identification of avian coronaviruses based on Raman spectra. Specifically, we design a spectral transformer to classify the Raman spectra of 32 avian coronavirus strains. After training, relevance maps can be generated through gradient and relevance propagation to further understand the contribution of each wavenumber to the identification. Experimental results show that the proposed method outperforms several machine learning and deep learning baseline models, and achieves 72.72% accuracy in the 32-class identification problem. The relevance maps generated reveal some wavenumber ranges that are important for the identification of almost all strains, and these ranges correlate with Raman peak ranges for lipids, nucleic acids, and proteins.
更多查看译文
关键词
Virus Identification, Raman Spectroscopy, Interpretable Machine Learning
AI 理解论文
溯源树
样例
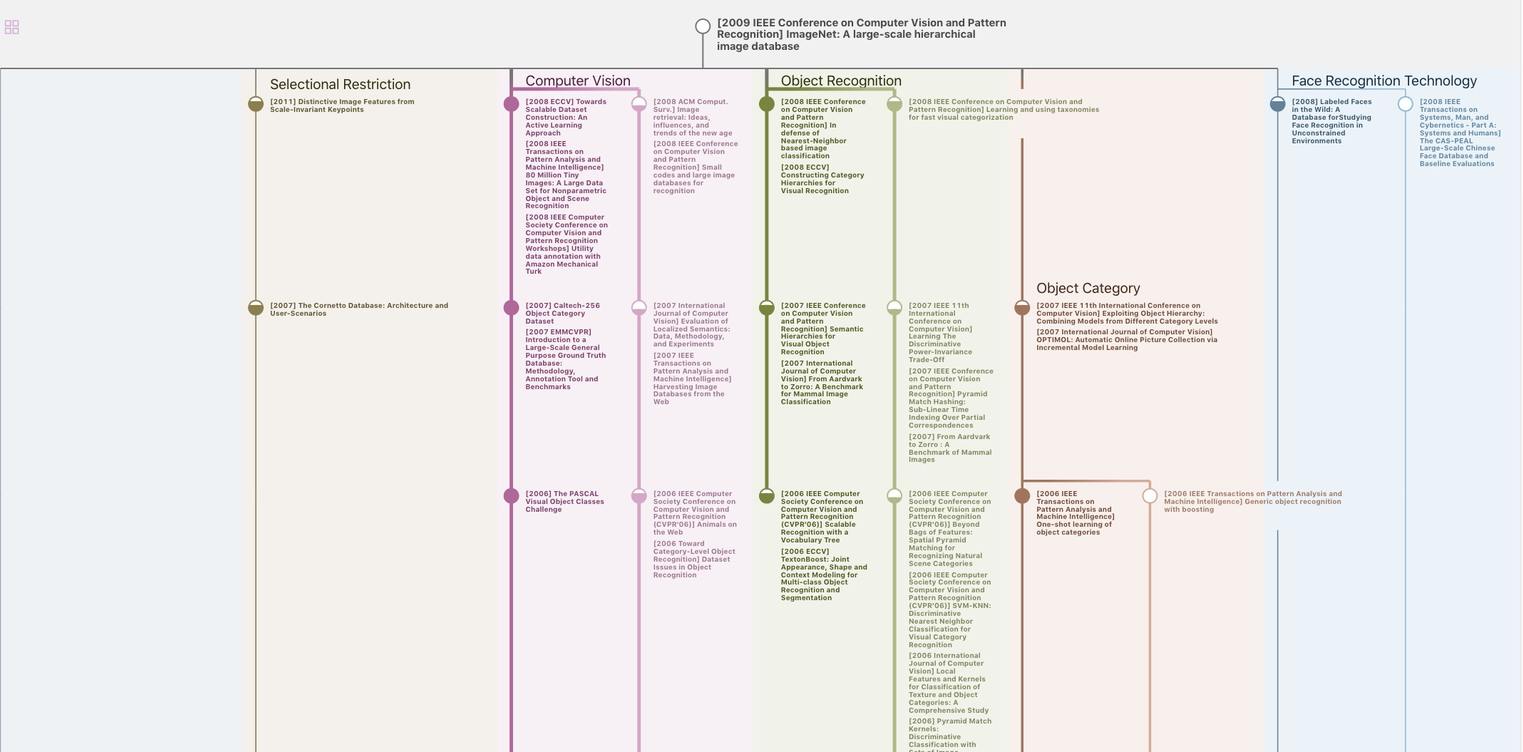
生成溯源树,研究论文发展脉络
Chat Paper
正在生成论文摘要