Scheduling Condition-based Maintenance: An Explainable Deep Reinforcement Learning Approach via Reward Decomposition.
FUSION(2023)
摘要
This paper presents an eXplainable Deep Reinforcement Learning (XDRL) based strategy for solving the proposed problem of fleet-level aircraft maintenance scheduling (AMS) optimization. The XDRL-AMS considers various factors such as the aircraft’s initial status, mission requirements, maintenance resource capacity, and operational constraints to create a maintenance schedule for a specified period. The schedule aims to balance both mission readiness and cost reduction. We developed an RL environment, called AMS-Gym, using the OpenAI Gym toolkit specifically designed for this problem. AMS-Gym is highly flexible, allowing for easy extension to more complex scenarios and incorporating additional explanatory capabilities. The explainable RL capability was achieved by utilizing a decomposed reward Deep Q-Network (drDQN) algorithm. In the context of the AMS scenario, the drDQN consists of two parts: (i) a DQN that aims to maximize the mission accomplishment objective, and (ii) a DQN that aims to minimize the maintenance cost objective. As a result, the proposed drDQN strategy can generate real-time aircraft maintenance decisions, explain why those decisions were selected, and present the tradeoffs between the chosen action and non-selected alternatives. Experiment results show that the proposed drDQN performs well, providing an approximate solution to the vanilla DQN with a simpler structure while offering the ability to explain its decisions. In addition, a web-based prototype with an intuitive textual and visual user interface was developed to demonstrate the feasibility of the drDQN approach.
更多查看译文
关键词
Aircraft Maintenance Scheduling,Explainable Artificial Intelligence,Explainable Reinforcement Learning,Markov Decision Process,Q-learning,Reward Decomposition,Decision Making,Autonomous Agent
AI 理解论文
溯源树
样例
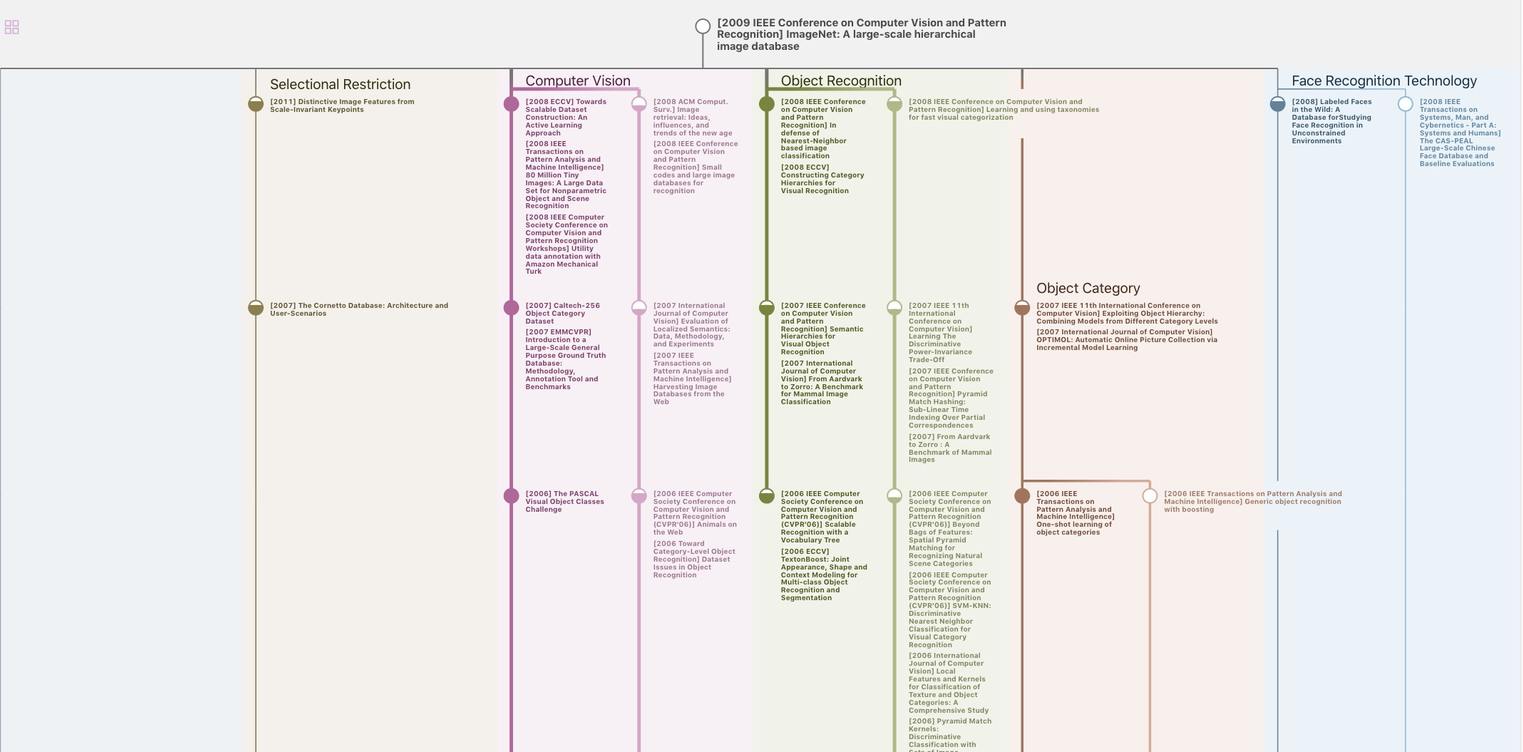
生成溯源树,研究论文发展脉络
Chat Paper
正在生成论文摘要