E2: Entropy Discrimination and Energy Optimization for Source-free Universal Domain Adaptation
ICME(2023)
摘要
Universal domain adaptation (UniDA) transfers knowledge under both distribution and category shifts. Most UniDA methods accessible to source-domain data during model adaptation may result in privacy policy violation and source-data transfer inefficiency. To address this issue, we propose a novel source-free UniDA method coupling confidence-guided entropy discrimination and likelihood-induced energy optimization. The entropy-based separation of target-known and unknown classes is too conservative for known-class prediction. Thus, we derive the confidence-guided entropy by scaling the normalized prediction score with the known-class confidence, that more known-class samples are correctly predicted. Due to difficult estimation of the marginal distribution without source-domain data, we constrain the target-domain marginal distribution by maximizing (minimizing) the known (unknown)-class likelihood, which equals free energy optimization. Theoretically, the overall optimization amounts to decreasing and increasing internal energy of known and unknown classes in physics, respectively. Extensive experiments demonstrate the superiority of the proposed method.
更多查看译文
关键词
Universal Domain Adaptation, Source-free Domain Adaptation, Confidence-guided Entropy, Energy
AI 理解论文
溯源树
样例
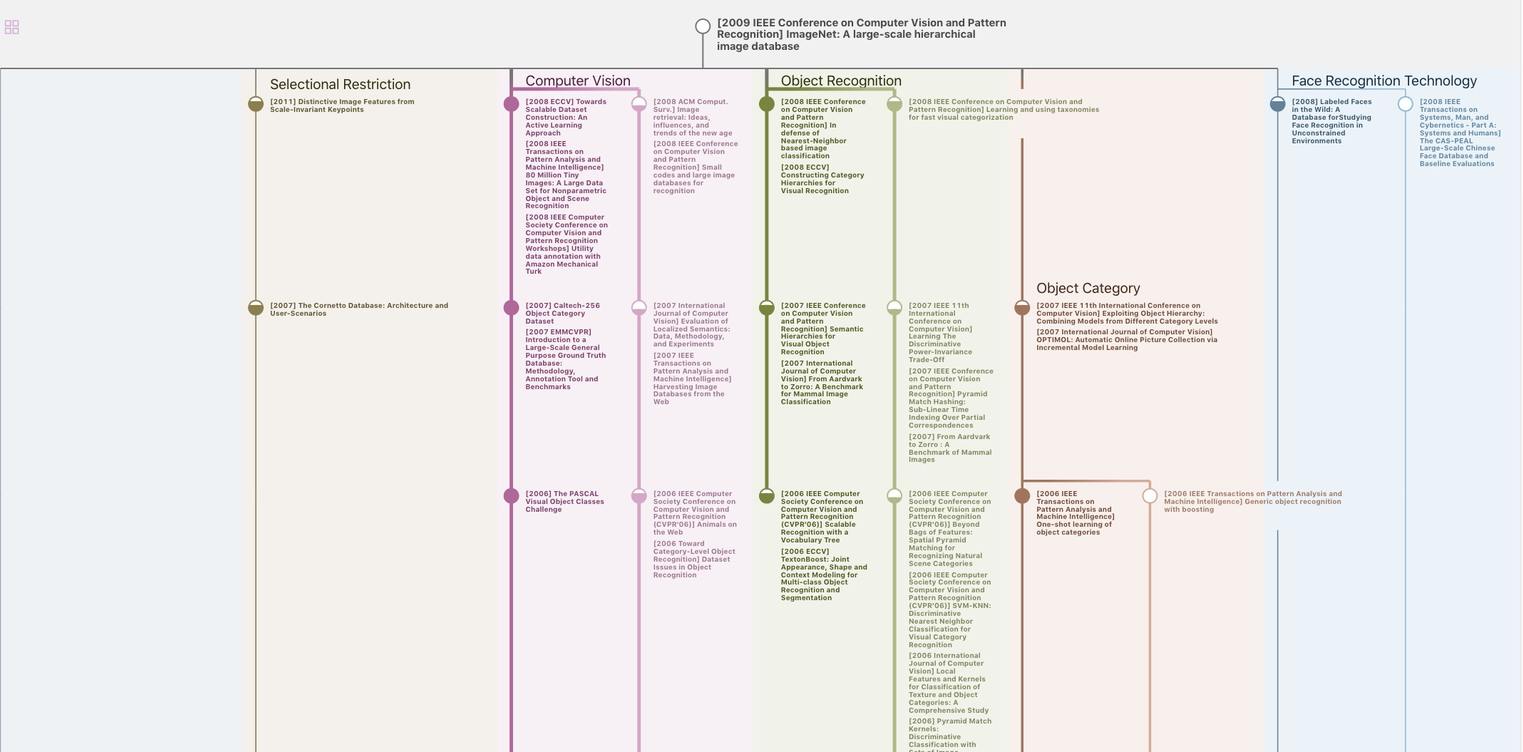
生成溯源树,研究论文发展脉络
Chat Paper
正在生成论文摘要